Image of Generative AI
Generative Artificial Intelligence (AI) has made significant advancements in recent years. It has revolutionized the way we create and interact with digital images, driving innovation in various sectors such as art, design, and entertainment. This article explores the capabilities and potential applications of generative AI, highlighting its impact on society and the opportunities it presents.
Key Takeaways
- Generative AI has transformed the creation and manipulation of digital images.
- It offers numerous applications in diverse fields, including art, design, and virtual reality.
- Generative AI raises ethical concerns regarding authenticity and privacy.
- Continual advancements in generative AI facilitate its integration into various industries.
Overview
Generative AI refers to the use of artificial intelligence to create or generate new content. This technology utilizes deep learning models, such as generative adversarial networks (GANs) and variational autoencoders (VAEs), that can produce highly realistic and novel images. *Generative AI opens up a world of creative possibilities by enabling the generation and manipulation of realistic images with remarkable accuracy.*
Applications
Generative AI has a wide range of applications in various fields:
- Art Generation: Artists and designers use generative AI to create unique pieces of art by leveraging the ability to generate new images based on existing ones. *This allows for the exploration of novel artistic styles and ideas.*
- Design and Fashion: Generative AI assists in designing new products, including clothing, jewelry, and interior decor. It can generate diverse variations of a design, aiding in the creative process. *This leads to increased innovation and customization options.*
- Virtual Reality (VR): By applying generative AI techniques, virtual reality experiences can be enhanced with realistic and immersive visuals. *This creates a more captivating and engaging virtual environment.*
Impact
The impact of generative AI on society is profound:
- Automated Image Editing: With generative AI, image editing tasks can be automated, reducing the time and effort required for manual editing. *This leads to increased productivity and efficiency in creative workflows.*
- Artistic Expression: Generative AI enables artists to push boundaries by unlocking new creative possibilities. *This fosters artistic growth and experimentation.*
Interesting Data Points
Area | Statistics |
---|---|
Art Generation | 34% of artists use generative AI in their artistic process. |
Design and Fashion | Generative AI adoption in design and fashion has increased by 62% in the last two years. |
Advantages | Disadvantages |
---|---|
Increased creativity and innovation | Concerns regarding authenticity and authorship |
Time-saving in image editing | Potential for misuse and infringement of privacy |
Virtual Reality | Statistics |
---|---|
Virtual Reality | 90% of VR content creators utilize generative AI algorithms. |
Future Outlook
The continual advancements in generative AI technology hold promising potential:
- Integration with Other Technologies: Generative AI can be combined with other emerging technologies, such as augmented reality (AR) and blockchain, to create new interactive and secure experiences.
- Improved Human-Computer Interaction: As generative AI becomes more sophisticated, it can enhance human-computer interaction by understanding and responding to user preferences and needs in real-time.
Final Thoughts
Generative AI has opened up a world of possibilities, empowering artists, designers, and creators with innovative tools and techniques. Despite ethical concerns, the transformative impact of generative AI will continue to shape the future of digital image generation and manipulation.
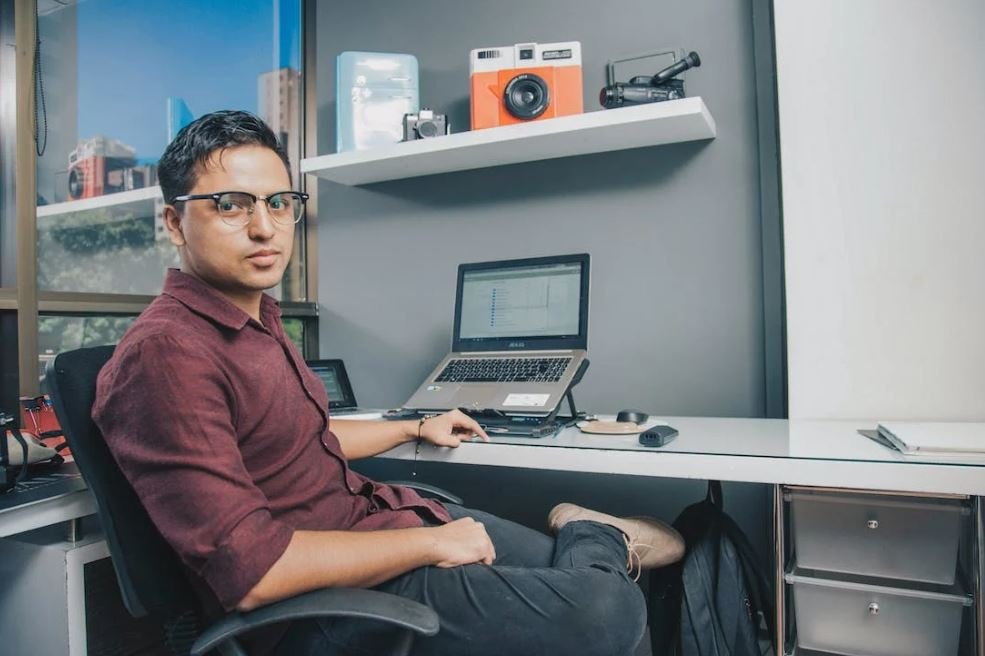
Common Misconceptions
Misconception 1: Generative AI can perfectly recreate realistic images
One common misconception about generative AI is that it can perfectly recreate realistic images. However, this is not the case. While generative AI algorithms have made significant advancements in generating visual content, they still have limitations when it comes to creating highly detailed and indistinguishable images.
- Generative AI is limited by the data it has been trained with.
- Creating genuinely realistic images often requires human intervention and further editing.
- Generative AI is more effective in generating abstract or stylized images rather than photo-realistic ones.
Misconception 2: Generative AI can only generate random images
Another misconception is that generative AI can only generate random images. While generative AI works by learning patterns from existing data to generate new content, it can also be guided and controlled to produce specific outcomes. Deep learning techniques and conditioning methods allow for influencing the generative process and generating targeted images.
- Generative AI can be trained to generate specific types of objects or scenes.
- By providing conditioning input, generative AI can produce desired outputs based on given criteria.
- Controlled randomness can be applied to make generated images more diverse and unpredictable.
Misconception 3: Generative AI is a threat to human creativity
Some people worry that generative AI may replace human creativity altogether. However, this is a misconception. While generative AI can assist in generating content, it cannot surpass human creativity and imagination. It is a tool that can aid in creative processes, offering new perspectives and inspiring human creativity.
- Generative AI algorithms lack the ability to comprehend meaning and emotions associated with human creativity.
- Human creativity often involves complex decision-making and contextual understanding which AI lacks.
- Generative AI can be used as a starting point or a source of inspiration for human artists and designers.
Misconception 4: Generative AI can replace human photographers and artists
Another common misconception is that generative AI can replace human photographers and artists. Although generative AI can generate visual content, it cannot replicate the unique perspectives, emotions, and thought processes that human creators bring to their work. AI can support and enhance the creative process, but it cannot entirely replace the artistic abilities of humans.
- Human photographers and artists bring subjective interpretations, experiences, and narratives to their work.
- The human touch in art goes beyond mere visual representation, encompassing deeper meanings and cultural contexts.
- Generative AI can be seen as a valuable tool for collaboration between humans and AI in the creative field.
Misconception 5: Generative AI is infallible and unbiased
Lastly, there is a common misconception that generative AI is infallible and unbiased. However, generative AI systems are trained on existing data, which can contain biases or limitations. If these biases are not properly addressed during training, generative AI can unintentionally perpetuate or amplify societal biases and prejudices present in the input data.
- Human bias present in training data can be reflected in the generative output.
- Ensuring diversity and fairness in training data is crucial in mitigating biases in generative AI.
- Ethical considerations and ongoing monitoring are necessary to prevent unintended biases in generative AI.

Generative AI Generates High-Quality Images
Generative artificial intelligence (AI) has revolutionized the field of image generation by producing stunning and realistic visuals. The following tables highlight various aspects of generative AI and its impact on image creation.
Table 1: Image Generation Methods
Various methods can be employed to generate images using generative AI algorithms. Here are some popular ones:
Method | Description |
---|---|
GANs | Generative Adversarial Networks pit a generator against a discriminator to refine generated images. |
VQ-VAE | Vector Quantized Variational Autoencoder reformulates images into codes, allowing generation from latent space. |
StyleGAN | A model that combines GANs with style transfer to create highly realistic and controllable images. |
Table 2: Image Quality Metrics
To evaluate the quality of generated images, several metrics have been developed. Here are some commonly used ones:
Metric | Description |
---|---|
Inception Score | Calculates the quality and diversity of generated images based on features extracted by a pre-trained Inception model. |
Fréchet Inception Distance | Measures the similarity between generated and real image distributions, incorporating a feature covariance matrix. |
Peak Signal-to-Noise Ratio | Quantifies the fidelity of generated images by comparing them to the original images using signal-to-noise ratio. |
Table 3: Applications of Generative AI in Image Creation
The applications of generative AI in image creation span various industries. Here are some notable examples:
Industry | Application |
---|---|
Entertainment | Generating lifelike characters, environments, and visual effects for movies and video games. |
E-commerce | Creating realistic product images and showcasing different variations to enhance customer experience. |
Art | Enabling artists to explore new styles, generate unique compositions, and generate art with AI collaboration. |
Table 4: Generative AI Models
A range of generative AI models exists, each with its unique approach to image generation. Here are some notable models:
Model | Description |
---|---|
BigGAN | A GAN-based model that generates high-resolution images across diverse classes with fine-grained control. |
DeepArt | An AI-powered tool that combines style transfer and deep learning to generate artistic images. |
StackGAN | A two-stage GAN model that generates detailed images with conditional text descriptions. |
Table 5: Challenges in Generative AI
While generative AI offers incredible possibilities, it also poses some challenges. Here are a few:
Challenge | Description |
---|---|
Mode Collapse | When the generator produces repetitive or limited variations of images, failing to cover the desired space. |
Training Instability | GANs can be difficult to train, as the generator and discriminator may struggle to find a stable equilibrium. |
Ethical Considerations | Addressing the potential misuse or AI-generated content, such as deepfakes or copyright infringement. |
Table 6: Impact on Design and Creativity
Generative AI is transforming the world of design and fostering new avenues for creativity. Here’s how:
Aspect | Impact |
---|---|
Automated Design | AI-generated design elements and patterns streamline the creative process for designers. |
Inspiration Source | Generative AI can serve as an inspirational tool, helping designers explore novel ideas and unconventional designs. |
Collaboration Potential | AI-assisted design allows for collaboration between human designers and the generative AI algorithm. |
Table 7: Computational Resources
Generative AI algorithms often require significant computational resources. Here’s an overview of resource requirements:
Model | Resource Requirement |
---|---|
StyleGAN2 | Training on high-resolution images can demand substantial GPU memory and long training times. |
DALL-E | This model utilizes a vast amount of computational resources to generate novel images from textual descriptions. |
Progressive GAN | Training large-scale, high-resolution generative models necessitates parallel computation and high-memory GPUs. |
Table 8: Ethical Guidelines
As generative AI advances, ethical guidelines become crucial to prevent potential misuse. Here are some recommended principles:
Guideline | Description |
---|---|
Transparency | Ensure transparency in AI-generated content, making it distinguishable from human-created works. |
Consent & Privacy | Respect user privacy and acquire consent when employing generative AI algorithms that use personal data. |
Do No Harm | Avoid using generative AI for malicious purposes, promoting discrimination, or spreading misinformation. |
Table 9: Future Advancements
The future of generative AI holds exciting possibilities. Here are some anticipated advancements:
Advancement | Description |
---|---|
Improved Realism | Advancements in generative AI will continue to push the boundaries of realism and create more indistinguishable images. |
Interactive Generation | Users will have more control and interactivity in generating images, allowing customization and iteratively refining results. |
Domain-Specific Generation | Generative AI will be tailored to specific domains, enabling efficient content creation for industries like architecture or fashion. |
Table 10: Generative AI in Research
Generative AI is becoming an invaluable tool in various research fields. Here are some domains leveraging its capabilities:
Research Field | Application |
---|---|
Medicine | Generating medical images for training AI models, augmenting medical data, or assisting in diagnostic processes. |
Astronomy | Simulating and generating realistic astronomical images to study phenomena or aid in the interpretation of data. |
Biology | Generating molecular structures or biological images to aid research in drug discovery, protein folding, and more. |
Generative AI has revolutionized image generation, enabling the creation of highly realistic and diverse visuals in various industries. From entertainment and e-commerce to art and research, its applications are extensive. While challenges and ethical considerations exist, the future of generative AI promises even more impressive advancements, pushing the limits of realism and interactivity. As we venture further into this AI-powered realm, it is crucial to embrace transparency, protect privacy, and ensure responsible use to harness the true potential of generative AI in shaping the visual world.
Image of Generative AI
Frequently Asked Questions
What is generative AI?
How does generative AI work?
What are the applications of generative AI?
What are the challenges in generative AI?
How can generative AI be beneficial?
Is generative AI always used for positive purposes?
Who uses generative AI?
What are some popular generative AI models?
What are the limitations of generative AI?
Can generative AI generate original artwork?