Image Generative Data
Image generative data refers to a set of techniques and algorithms used to generate new images based on existing data. This technology has gained significant traction in recent years, with applications ranging from art and design to computer vision and artificial intelligence. By analyzing patterns and characteristics in existing images, generative models can create new content that closely resembles the original data, while also introducing novel elements and variations.
Key Takeaways
- Image generative data techniques create new images based on existing data.
- These techniques are used in various fields, including art, design, computer vision, and artificial intelligence.
- Generative models analyze patterns in images to create new content with both resemblance and novelty.
Applications of Image Generative Data
One of the major applications of image generative data is in art and design. Artists and designers can use generative techniques to create unique and innovative visuals, exploring new possibilities and pushing boundaries. With generative models, they can generate diverse images, patterns, and even entire scenes at the click of a button. This empowers designers by augmenting their creative process and expanding their creative output.
Another field where image generative data is making significant strides is computer vision. By training generative models on vast amounts of visual data, computer vision systems can better understand and interpret images. This enables more accurate object recognition, scene understanding, and image classification. With generative models, computer vision algorithms can generate new images to analyze and test the capabilities of these systems, improving their overall performance.
An interesting application of image generative data is in the field of artificial intelligence. Generative models are employed to create synthetic data for training AI systems. By generating large volumes of labeled images, these models can facilitate the training of deep learning models at scale. This is particularly useful when dealing with limited or imbalanced datasets, as the generative models can fill in gaps and create more diverse training samples.
Generative Models and Techniques
Generative models are at the core of image generative data. Two popular types of generative models are autoencoders and generative adversarial networks (GANs). Autoencoders learn to encode input images into a lower-dimensional representation and then generate new samples by decoding this representation. GANs consist of two networks, a generator and a discriminator, which compete against each other. The generator aims to create realistic images to fool the discriminator, while the discriminator learns to distinguish between real and fake samples. This adversarial setup encourages the generator to produce more realistic and diverse images over time.
An interesting technique used in image generative data is style transfer. Style transfer allows the combination of the content of one image with the artistic style of another. By applying the style of a famous painter, for example, to a photograph, style transfer can create unique and artistic interpretations of images. This technique has gained popularity in various creative fields, bridging the gap between art and technology.
Data and Computational Requirements
Training generative models for image generation requires large volumes of labeled datasets. The availability of high-quality, diverse, and accurately labeled images is crucial for training effective generative models. Access to powerful computational resources is also necessary, as training generative models can be computationally intensive, requiring significant processing power and memory.
Tables: Interesting Info and Data Points
Generative Model | Application |
---|---|
Autoencoders | Art and design |
GANs | Computer vision |
Style Transfer | Artistic interpretations |
Benefits of Image Generative Data |
---|
Expanded creativity and innovation |
Improved computer vision systems |
Efficient AI training with synthetic data |
Requirements for Image Generative Data |
---|
Labeled datasets for training |
Powerful computational resources |
Future Potential and Ethical Considerations
The future potential of image generative data is vast. As the technology advances, we can expect even more realistic and diverse generated images. This opens up new possibilities for content creation, artistic expression, and innovation across various industries. However, ethical considerations must also be taken into account. With the ability to generate realistic fake images, there is a risk of misuse, such as generating deepfakes or unauthorized content. Responsible use and regulation are essential to ensure image generative data benefits society without causing harm.
Unleashing Creativity with Image Generative Data
Image generative data has revolutionized the way we create, analyze, and interpret visual content. From art and design to computer vision and artificial intelligence, the applications are diverse and far-reaching. With generative models and techniques, we can unleash our creativity, improve our systems, and explore new frontiers. The potential of image generative data is immense, and as technology continues to advance, we are only scratching the surface of its capabilities.
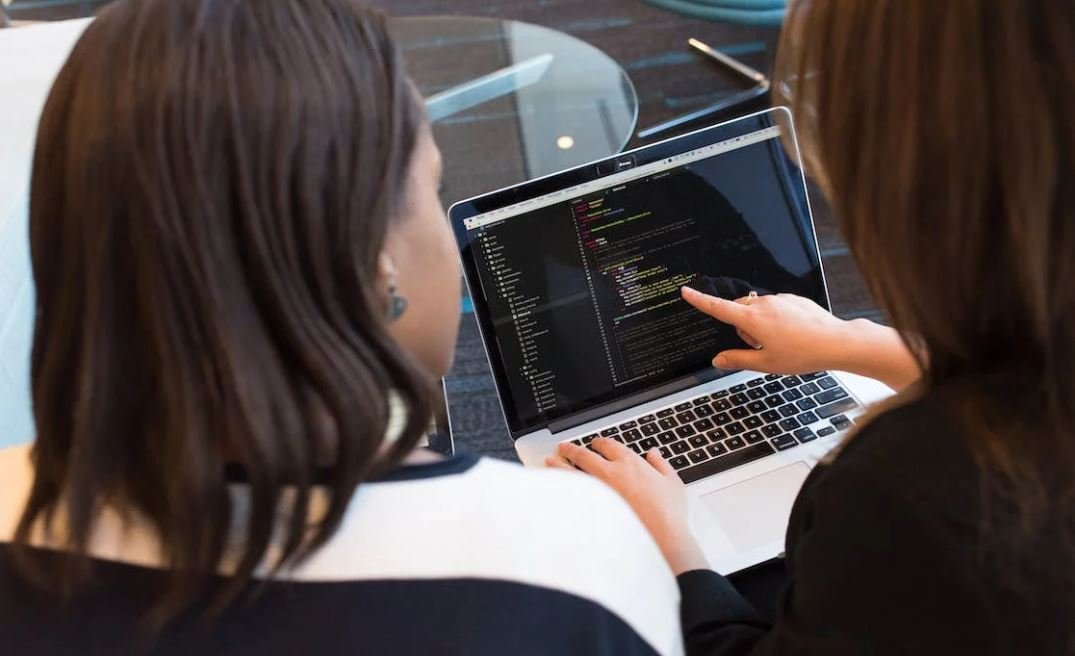
Common Misconceptions
Image Generative Data
There are several common misconceptions that people have about image generative data, which often leads to misunderstandings and misinterpretations about its purpose and applications. It is important to address these misconceptions in order to have a clearer understanding of this topic.
- Image generative data is only used for creating images
- Image generative data can replace traditional photography
- Image generative data is only relevant for artistic purposes
Firstly, one common misconception is that image generative data is solely used for the creation of computer-generated images or digital artwork. While it is true that this technology can be used for artistic purposes, it has far wider applications beyond that. Image generative data can be used in various industries such as fashion, interior design, and product visualization, enabling designers to generate realistic virtual models and prototypes.
- Image generative data can be used for realistic product visualization
- Image generative data is used in various industries, such as fashion and interior design
- Image generative data enhances the efficiency of product development processes
Secondly, there is a common misconception that image generative data can completely replace traditional photography. Although image generative data can produce realistic synthetic images, it cannot fully replicate the intricacies and nuances of real-world photography. Traditional photography continues to play a crucial role in capturing the essence of physical objects and environments, while image generative data complements this process by enabling the creation of 3D models and digital representations.
- Image generative data cannot replace traditional photography
- Traditional photography captures the essence of physical objects and environments
- Image generative data complements traditional photography
Lastly, image generative data is often mistakenly seen as relevant only for artistic purposes. However, its applications go beyond art. Image generative data has practical uses in scientific and medical fields, allowing researchers to generate computer-based models for better understanding anatomy, simulating experiments, and aiding in the development of new medical treatments and procedures.
- Image generative data has practical applications in scientific and medical fields
- Researchers use image generative data for better understanding anatomy
- Image generative data aids in the development of new medical treatments and procedures
In conclusion, it is important to dispel the common misconceptions surrounding image generative data. It has diverse applications beyond artistic purposes, including product visualization, scientific research, and medical innovations. Understanding the true potential of this technology can help us harness its capabilities to enhance various industries and fields.
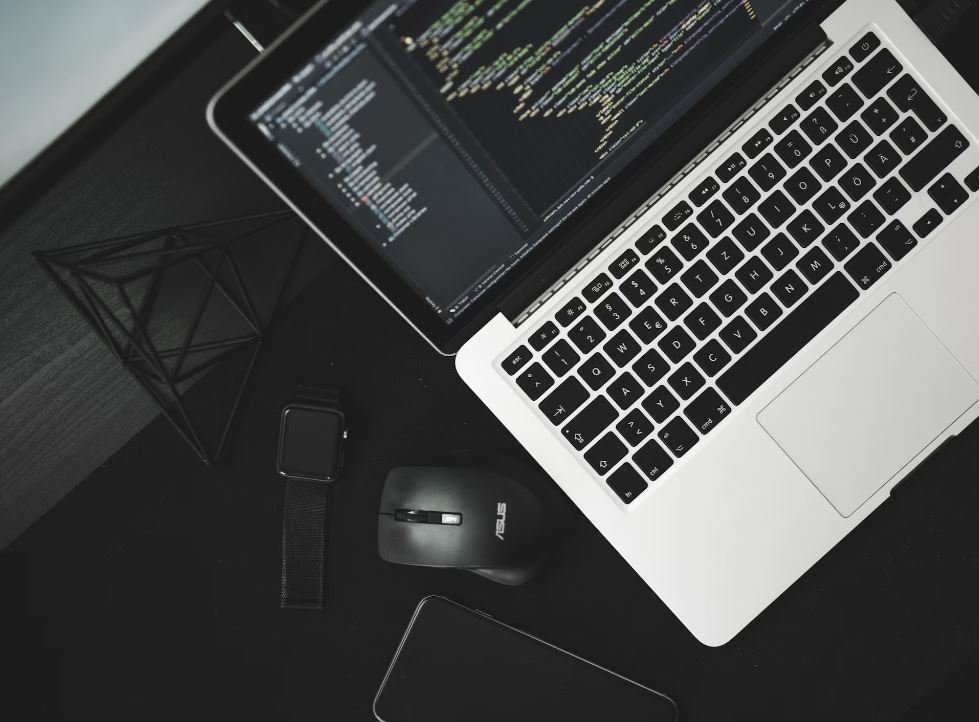
Generative Image Data of Famous Artwork
The following table showcases a comparison of famous artwork from various art movements and their corresponding generative image data. Each art movement represents a unique style and approach to art, while generative image data explores the potential of artificial intelligence and computer algorithms in creating visually captivating artwork.
| Art Movement | Famous Artwork | Generative Image Data |
|—————-|————————-|————————–|
| Renaissance | Mona Lisa |  |
| Impressionism | Water Lilies |  |
| Cubism | Les Demoiselles d’Avignon|  |
| Surrealism | The Persistence of Memory|  |
| Pop Art | Campbell’s Soup Cans |  |
Generative Image Data of Famous Landmarks
This table showcases generative image data of well-known landmarks around the world, highlighting the diverse architectural styles and cultural significance they embody. Generative image data allows us to appreciate the intricate details of these landmarks and explore alternative visual representations.
| Landmark | Location | Generative Image Data |
|——————-|——————-|————————–|
| Eiffel Tower | Paris, France |  |
| Taj Mahal | Agra, India |  |
| Great Wall | Beijing, China |  |
| Statue of Liberty | New York, USA | |
| Pyramids of Giza | Cairo, Egypt | |
Generative Image Data of Endangered Species
This table presents generative image data of endangered species, raising awareness about the importance of conservation and protecting their habitats. Generative image data encourages us to appreciate the beauty and diversity of these species, ultimately inspiring efforts to preserve their existence.
| Species | Common Name | Generative Image Data |
|—————-|———————–|————————–|
| Panthera pardus | Leopard |  |
| Ailuropoda melanoleuca | Giant Panda |  |
| Diceros bicornis | Black Rhinoceros | |
| Balaenoptera musculus | Blue Whale |  |
| Gorilla gorilla | Western Gorilla |  |
In this article, we explored the fascinating concept of generative image data, which transcends traditional boundaries and explores the intersection between art, architecture, and environmental conservation. By harnessing the power of artificial intelligence and computer algorithms, generative image data allows us to visualize and appreciate the beauty of various subjects in captivating and unique ways. Whether it’s famous artwork, iconic landmarks, or endangered species, generative image data offers a fresh perspective and encourages us to delve deeper into the world around us. Through this exploration, we can cultivate a greater sense of appreciation and understanding for the wonders that surround us.
Frequently Asked Questions
What is image generative data?
Image generative data refers to a type of data that is used to generate or create images through algorithms, machine learning models, or other computational techniques. It involves using a set of input data to generate new images that possess certain desired features, patterns, or characteristics.
How is image generative data different from traditional image data?
Traditional image data typically consists of pre-existing images captured or created by humans, while image generative data is created or generated by computers or algorithms. Image generative data allows for the creation of new, synthetic images based on desired specifications, enabling applications in various fields such as computer vision, art, and design.
What are the applications of image generative data?
Image generative data has a wide range of applications, including but not limited to:
- Art and creative expression
- Content creation for video games
- Computer vision tasks, such as object recognition and classification
- Data augmentation for training machine learning models
- Style transfer and image manipulation
What are some popular algorithms used for image generative data?
Several popular algorithms for image generative data include:
- Generative Adversarial Networks (GANs)
- Variational Autoencoders (VAEs)
- Deep Convolutional Generative Adversarial Networks (DCGANs)
- Restricted Boltzmann Machines (RBMs)
- PixelRNN and PixelCNN
What are the benefits of using image generative data?
Using image generative data offers several benefits, such as:
- Creation of realistic and novel images
- Ability to control and manipulate image characteristics
- Improved data augmentation for training models
- Reduced reliance on large pre-existing image datasets
- Exploration of artistic and creative possibilities
Are there any challenges associated with image generative data?
Yes, there are challenges involved in working with image generative data, including:
- Generating high-quality and visually appealing images
- Maintaining diversity and avoiding overfitting
- Ensuring computational efficiency
- Data privacy and ethical considerations
- Evaluating and measuring image generative models
How can image generative data be used in art and design?
Image generative data can be a powerful tool in art and design. It allows artists and designers to create unique and visually striking images by harnessing the capabilities of generative algorithms. Artists can manipulate various parameters, styles, and characteristics of the generated images to create pieces that push the boundaries of traditional art and design.
Can image generative data be used for facial recognition or image synthesis?
Yes, image generative data can be used for facial recognition and image synthesis. By training generative models on large datasets of facial images, it is possible to generate new faces or enhance existing ones. This has applications in areas such as virtual avatars, digital identity verification, and even forensic face reconstruction.
What ethical considerations should be taken into account when working with image generative data?
When working with image generative data, it is essential to consider ethical considerations, especially in areas such as:
- Privacy and consent of individuals whose images are used in datasets
- Potential misuse of generative models for malicious purposes
- Bias and fairness in image generation, particularly regarding race, gender, or other sensitive attributes
- Transparency and accountability in the development and use of generative models