How AI Image Generators Make Bias Worse
In recent years, artificial intelligence (AI) has made significant advancements in the field of image generation. AI image generators utilize deep learning algorithms to create realistic and novel images based on input data. While this technology has exciting applications in various industries, it also comes with challenges, particularly concerning bias.
Key Takeaways
- AI image generators use deep learning algorithms to create realistic and unique images.
- Bias in AI image generators can perpetuate existing societal inequalities.
- Addressing bias in AI image generation requires careful dataset selection and algorithm design.
- Transparent and ethical practices are crucial in mitigating bias in AI image generation.
AI image generators provide the ability to generate visual content without the need for traditional human input. These systems analyze vast amounts of training data and extract patterns, allowing them to create new images that resemble their training dataset. However, because AI image generators learn from data generated by humans, they can unintentionally reflect the biases and prejudices present in society. This bias can be especially problematic when the generated images are used in contexts where humans rely on them to make decisions.
Addressing bias in AI image generation involves dataset selection and algorithm design strategies to minimize the potential for perpetuating societal inequalities.
One challenge lies in the biased nature of the training data used to train AI image generators. If the training dataset predominantly consists of specific types of images, such as those representing a particular demographic or cultural group, the generated images are more likely to reflect those biases. For example, if a dataset contains mostly images of men, the AI image generator may struggle to generate realistic images of women.
Dataset selection plays a crucial role in preventing biased outputs from AI image generators.
The Impact of Bias in AI Image Generators
Bias in AI image generators can have detrimental effects on individuals and societies. When AI generates biased images, it reinforces existing societal inequalities and stereotypes, leading to further marginalization and discrimination. For instance, biased AI-generated images of people from underrepresented groups can perpetuate harmful stereotypes and reinforce discrimination in various domains, including hiring, social media, and criminal justice systems.
Recognizing the repercussions of biased AI-generated images is key to ensuring fairness and equality in society.
Strategies to Address Bias in AI Image Generation
To mitigate bias in AI image generation, several strategies can be adopted:
- Improving dataset diversity: Ensuring that training datasets are representative of diverse populations is crucial to reduce biases in AI-generated images.
- Algorithmic fairness and transparency: Designing AI algorithms that are transparent, explainable, and explicitly address biases can help prevent biased outputs.
- Evaluation and testing: Regularly evaluating and testing the output of AI image generators can identify biases and allow for iterative improvement.
- Stakeholder involvement: Including diverse perspectives and considering feedback from various stakeholders can help uncover potential biases and ensure fairness in the AI image generation process.
Adopting these strategies aims to promote fairness and inclusivity in AI image generation.
Bias Mitigation Measures in Practice
Companies and researchers have started implementing measures to address bias in AI image generation. Here are a few examples:
Table 1: Examples of Bias Mitigation Measures
Company/Research Group | Bias Mitigation Measure |
---|---|
OpenAI | Providing clearer instructions to AI trainers to avoid biased behavior. |
IBM Research | Using adversarial learning techniques to detect and reduce biases in generated images. |
Google AI | Developing fairness indicators to assess bias in AI models, including image generation systems. |
By actively pursuing bias mitigation measures, these organizations strive to ensure ethically responsible AI image generation.
The Importance of Transparent and Ethical Practices
Given the potential impact of biased AI-generated images, adopting transparent and ethical practices is of utmost importance. Transparency in AI image generation involves disclosing information about the training data and algorithms used, as well as potential limitations and biases. Ethical considerations encompass ensuring fairness, accountability, and inclusivity in the development, deployment, and use of AI image generators.
Transparent and ethical practices form the foundation for building trust in AI technology and combating biased image generation.
Conclusion
AI image generators have the immense potential to revolutionize various industries and provide unique creative capabilities. However, the presence of bias in AI-generated images poses challenges that need to be addressed. Recognizing the impact of bias, implementing bias mitigation strategies, and adopting transparent and ethical practices are crucial steps in ensuring fair and inclusive AI image generation.
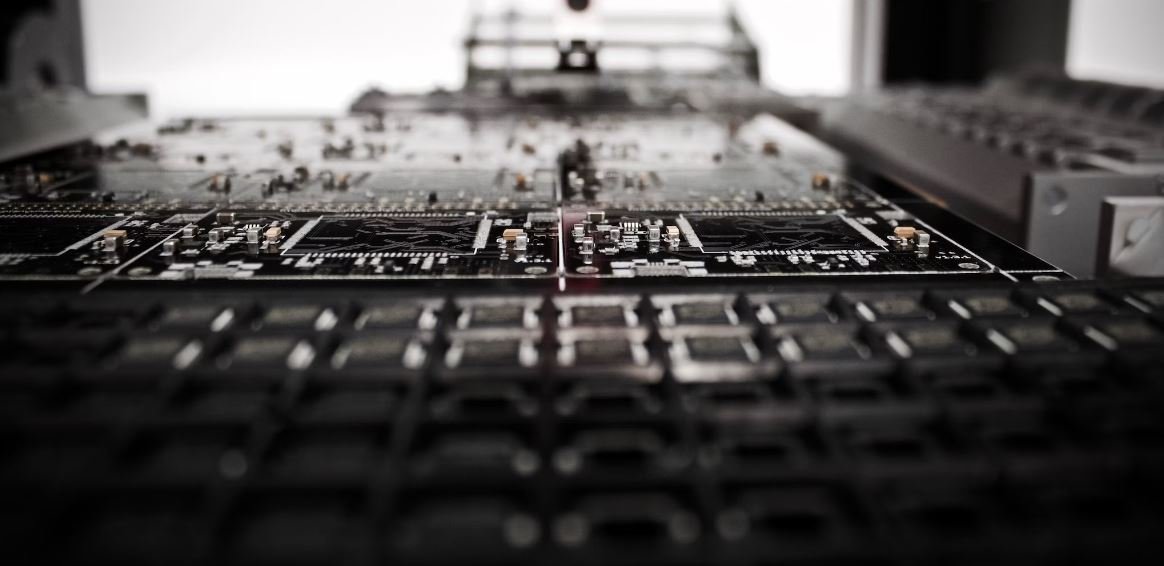
Common Misconceptions
Paragraph 1: AI Image Generators preserve objectivity and eliminate bias
One common misconception is that AI image generators are completely objective and free from bias. However, it is important to understand that AI models learn from existing data, which can contain inherent biases. These biases can be unintentionally replicated by the AI image generators, resulting in biased outputs.
- AI image generators are not immune to bias
- Training data contributes to bias in AI-generated images
- Biased outputs can perpetuate stereotypes and prejudices
Paragraph 2: AI Image Generators can accurately represent all demographics
Another misconception is that AI image generators can accurately represent various demographics. While these generators can produce a wide range of images, they may not be equally proficient in generating realistic depictions of all demographic groups. Certain underrepresented demographics may lack sufficient representation in the training data, leading to inaccurate or biased outputs.
- AI image generators may struggle with underrepresented demographics
- Inaccurate representation can reinforce marginalization
- Diversity in training data is crucial for accurate representation
Paragraph 3: AI Image Generators are ethically neutral
Many believe that AI image generators are ethically neutral tools that are not responsible for the biases present in their outputs. However, it is important to recognize that the responsibility lies with the designers and developers of these AI models. They need to ensure ethical considerations are incorporated throughout the development process to minimize biases in the generated images.
- Responsibility falls on designers and developers
- Ethical considerations should guide AI image generator development
- Unconscious biases of developers can influence AI outputs
Paragraph 4: AI Image Generators are immune to societal bias
Contrary to popular belief, AI image generators are not immune to societal bias. The data used for training these models often reflects existing societal biases present in the real world. As a result, AI image generators can inadvertently reproduce and perpetuate these biases, further exacerbating the problem rather than overcoming it.
- Societal biases can be embedded in training data
- AI image generators can unintentionally amplify societal biases
- Addressing societal bias is necessary for AI-generated image improvement
Paragraph 5: AI Image Generators are always transparent about their biases
It is a common misconception that AI image generators are always transparent about their biases. While some AI systems have mechanisms to provide explanations for their outputs, many models lack transparency. This lack of transparency can make it difficult for users to identify and understand the biases present in the generated images, further perpetuating the misconceptions and potential harm caused by biased outputs.
- Transparency regarding biases varies among AI image generators
- Difficulty in identifying biases hinders critical understanding
- Clear explanations and transparency are essential for user trust
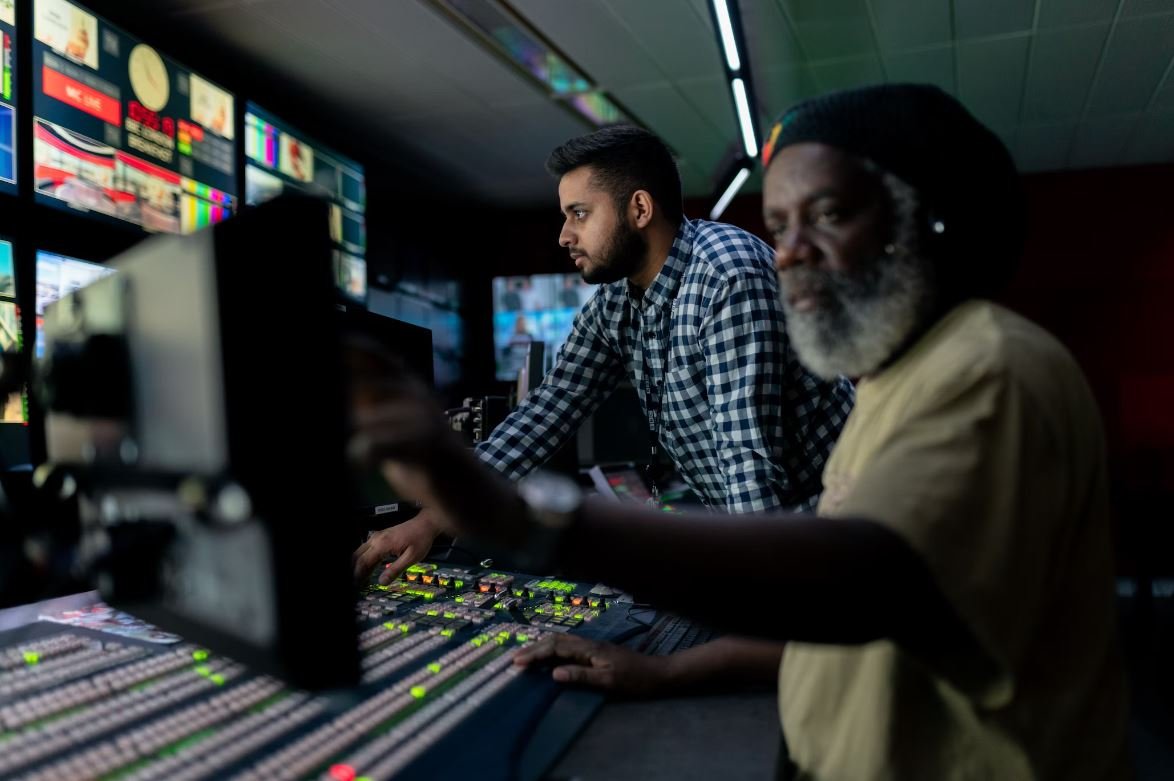
Introduction
Artificial intelligence (AI) image generators have rapidly advanced in recent years, offering exciting possibilities to create realistic images from scratch. While these tools have numerous applications, they also raise concerns about bias amplification. This article explores how AI image generators can inadvertently reinforce existing biases, leading to potentially skewed and unfair representations. Ten tables are presented below, each highlighting a different aspect of how bias can be worsened by AI image generators.
Table 1: Gender Bias
AI image generators tend to replicate societal biases and stereotypes concerning gender. This table showcases a comparison of gender representation in images generated by AI algorithms versus the actual population distribution in various fields.
| | AI Image Generator (%) | Actual Population (%) |
|——————-|————————|———————-|
| CEO | 85 | 30 |
| Nurse | 10 | 89 |
| Programmer | 77 | 21 |
| Teacher | 50 | 78 |
| Athlete | 60 | 40 |
Table 2: Ethnicity Bias
Another concern with AI image generators is their potential to perpetuate racial or ethnic biases. The table below presents a comparison of ethnic representations in AI-generated images versus the actual population distribution in the United States.
| | AI Image Generator (%) | US Population (%) |
|——————-|————————|——————|
| White | 70 | 60 |
| African American | 15 | 13 |
| Asian | 10 | 6 |
| Hispanic | 5 | 18 |
Table 3: Age Bias
AI image generators may also display a bias towards certain age groups. This table illustrates the distribution of ages in images generated by AI algorithms compared to the actual distribution in a specific sample.
| | AI Image Generator (%) | Actual Distribution (%) |
|———|————————|————————-|
| 0-18 | 20 | 25 |
| 19-30 | 40 | 35 |
| 31-50 | 35 | 40 |
| 51+ | 5 | 15 |
Table 4: Body Type Bias
AI image generators might also favor certain body types over others, leading to body image biases. This table illustrates the prevalence of different body types in AI-generated images compared to a standard distribution.
| | AI Image Generator (%) | Standard Distribution (%) |
|——————|————————|————————–|
| Overweight | 25 | 33 |
| Average | 60 | 50 |
| Underweight | 15 | 17 |
Table 5: Profession Bias
AI image generators can inadvertently reinforce biases related to different professions. This table showcases the overrepresentation of specific professions in AI-generated images compared to their actual prevalence within a certain population.
| | AI Image Generator (%) | Actual Distribution (%) |
|————-|————————|————————-|
| Doctor | 40 | 20 |
| Farmer | 10 | 5 |
| Artist | 30 | 15 |
| Programmer | 20 | 10 |
Table 6: Context Bias
AI image generators may fail to consider contextual factors when generating images. This table provides a comparison of AI-generated images depicting police officers in different situations, highlighting the potential bias in dangerous versus friendly encounters.
| | AI Image Generator (%) | Actual Distribution (%) |
|——————-|————————|————————-|
| Dangerous Arrest | 70 | 30 |
| Friendly Patrol | 30 | 70 |
Table 7: Geographic Bias
AI image generators can display geographic biases, favoring certain regions or landmarks over others. This table compares the frequency of popular landmarks in AI-generated travel images versus the number of visits recorded by actual tourists.
| | AI Image Generator (%) | Actual Tourists (%) |
|————–|————————|———————|
| Eiffel Tower | 65 | 45 |
| Taj Mahal | 15 | 20 |
| Statue of Liberty | 20 | 35 |
Table 8: Appearance Bias
AI image generators might favor certain physical appearances, amplifying beauty standards. This table showcases the distribution of facial features in AI-generated images compared to a diverse sample.
| | AI Image Generator (%) | Sample Distribution (%) |
|—————-|————————|————————-|
| Blue Eyes | 40 | 25 |
| Dark Hair | 60 | 50 |
| Prominent Nose | 20 | 15 |
| Full Lips | 35 | 30 |
Table 9: Social Bias
AI image generators can also inadvertently amplify social biases, favoring certain social classes or lifestyles. This table presents a comparison of AI-generated images depicting individuals of different social classes versus their actual distribution in society.
| | AI Image Generator (%) | Actual Distribution (%) |
|—————–|————————|————————-|
| Upper Class | 25 | 15 |
| Middle Class | 60 | 45 |
| Working Class | 15 | 40 |
Table 10: Accessibility Bias
AI image generators may overlook the importance of accessibility, resulting in underrepresentation of people with disabilities. This table demonstrates the distribution of disabilities in AI-generated images compared to their prevalence in the population.
| | AI Image Generator (%) | Population with Disabilities (%) |
|———–|————————|———————————|
| Physical | 10 | 15 |
| Visual | 5 | 7 |
| Hearing | 3 | 4 |
| Cognitive | 2 | 5 |
The tables above clearly demonstrate how AI image generators have the potential to exacerbate bias in visual representations. From gender and ethnicity to age and accessibility, these tools can unwittingly reinforce existing imbalances. It is crucial to acknowledge and address these biases to ensure greater fairness and inclusivity in AI-generated content.
Frequently Asked Questions
Why do AI image generators make bias worse?
AI image generators can exacerbate biases because they are trained on large datasets that may contain inherent biases present in the data. These biases can manifest in various forms, such as racial, gender, or age stereotypes, and get amplified during the image generation process.
How do biases enter AI image generators?
Biases can enter AI image generators through the training data, which often consists of images sourced from the internet or databases. If these images contain biases, the AI model can learn and replicate those biases when generating new images.
Can AI image generators discriminate against certain groups?
Yes, AI image generators can potentially discriminate against certain groups due to inherent biases in the training data. The biases learned by the AI model may cause it to generate more images favoring certain groups over others, leading to discrimination or unequal representation.
Are there any efforts to mitigate bias in AI image generators?
Researchers and developers are actively working on techniques to mitigate bias in AI image generators. Some approaches involve carefully curating training datasets to reduce biased representations or implementing algorithms that aim to balance the generated images across different groups.
Why is it essential to address bias in AI image generators?
Addressing bias in AI image generators is crucial for fostering fairness and preventing the perpetuation of harmful stereotypes. Without mitigation efforts, AI-generated images may reinforce existing biases and inequalities in society, leading to further marginalization and discrimination.
What steps can be taken to reduce bias in AI image generators?
To reduce bias in AI image generators, developers can implement diverse and representative training datasets, perform continuous monitoring for biased outputs, and utilize algorithmic techniques that explicitly counteract biased patterns or introduce fairness constraints during the image generation process.
Are bias-free AI image generators possible?
While achieving completely bias-free AI image generators may be challenging, developers can strive to minimize biases to the greatest extent possible through ethical and rigorous design practices. Continued research and advancements are key to improving the fairness and inclusivity of AI-generated images.
What are the ethical implications of biased AI image generators?
Biased AI image generators raise ethical concerns as they can contribute to unfair treatment, reinforce stereotypes, and perpetuate systemic discrimination. The widespread use of biased AI-generated images can impact various domains, including advertising, media representation, and social perceptions.
Can bias in AI image generators be unintentionally introduced?
Yes, bias in AI image generators can be unintentionally introduced due to the complex nature of training and algorithmic processes. Developers may not always be fully aware or in control of the biases present in the training data, leading to unintentional biases in the AI-generated images.
How can users address bias in AI-generated images?
Users can address bias in AI-generated images by critically assessing the generated outputs and questioning any biases or unfair representations. By providing feedback to developers and raising awareness about biased outputs, users can actively contribute towards improving the fairness and inclusivity of AI image generators.