Generative Image Generator
Generative Image Generators are powerful tools that use deep learning algorithms to create realistic and unique images from scratch. In a technological era where visual content plays a crucial role, these generators have revolutionized the way designers and artists create digital artwork. By leveraging the power of artificial intelligence, generative image generators provide endless possibilities for creative expression.
Key Takeaways
- Generative Image Generators use deep learning to create unique images.
- They have revolutionized the creative process for designers and artists.
- These tools offer endless possibilities for artistic expression.
*Generative Image Generators* utilize complex neural networks to analyze patterns and generate new images based on existing data. This process involves training the model with large datasets and allowing it to learn the underlying structure and features of the images. By understanding these patterns, the generator can then create new and realistic images that align with the desired style or theme.
The capability of generative image generators to *produce unique and original content* is remarkable. These tools can generate stunning images that have never been seen before, making them invaluable for artists looking to create something truly extraordinary. By tapping into the power of machine learning, generative image generators empower artists to unlock their creativity and explore new artistic horizons.
How Generative Image Generators Work
Generative Image Generators employ *deep learning techniques* such as **Generative Adversarial Networks (GANs)** or **Variational Autoencoders (VAEs)**. GANs, for example, consist of two neural networks: a *generator* and a *discriminator*. The generator attempts to create realistic images, while the discriminator evaluates the generated images against a training dataset to provide feedback for improvement. Through an iterative process of training and evaluation, the generator gradually learns to create more convincing and visually appealing images.
Applications of Generative Image Generators
Generative Image Generators have found applications in various fields, including:
- Art and design: Artists can use generators to explore new styles and create unique artwork.
- Graphics and media: They can enhance visual elements in games, films, and animations.
- Data augmentation: Generative models can be used to generate synthetic data for training machine learning models.
- Interior design: These generators offer possibilities for generating digital representations of furniture and decor.
Generative Image Generators vs. Traditional Image Manipulation
While traditional image manipulation tools like Photoshop allow artists to modify existing images or combine different elements creatively, *generative image generators go beyond that*. They possess the ability to generate completely new and original images, ensuring that artists are not limited by existing source materials. This innovation opens up doors for artists to experiment and create unique visual content without the need for extensive manual work.
Benefits and Limitations
Generative image generators offer several benefits, including:
- The ability to generate unique and original images effortlessly.
- Endless possibilities for creative exploration and experimentation.
- Time-saving by automating the image creation process.
However, it’s important to acknowledge the limitations of generative image generators. These tools heavily rely on large amounts of training data, computational resources, and fine-tuning for optimal results. Additionally, they are not yet able to fully match human intuition and creativity, often requiring human intervention and refinement to achieve desired outcomes.
Conclusion
Generative Image Generators have revolutionized the creative process by enabling artists and designers to generate unique and visually stunning images. By using deep learning algorithms, these tools offer endless possibilities for artistic expression and exploration. With their ability to create original content, generative image generators have become invaluable assets in the digital art world.
Name | Features |
---|---|
RunwayML | Interactive and user-friendly interface, support for various deep learning models and frameworks. |
DALLĀ·E | Capable of creating images from text descriptions, supports generating unique and creative content. |
Advantages | Disadvantages |
---|---|
Ability to generate unique and original content | Dependence on large amounts of training data |
Endless possibilities for creative exploration | Computational resource requirements |
Time-saving automation of image creation | Partial reliance on human intervention for refinement |
Art and Design | Graphics and Media | Data Augmentation | Interior Design |
---|---|---|---|
Exploring new artistic styles | Enhancing visual elements in media | Generating synthetic data for ML training | Creating digital representations of decor |
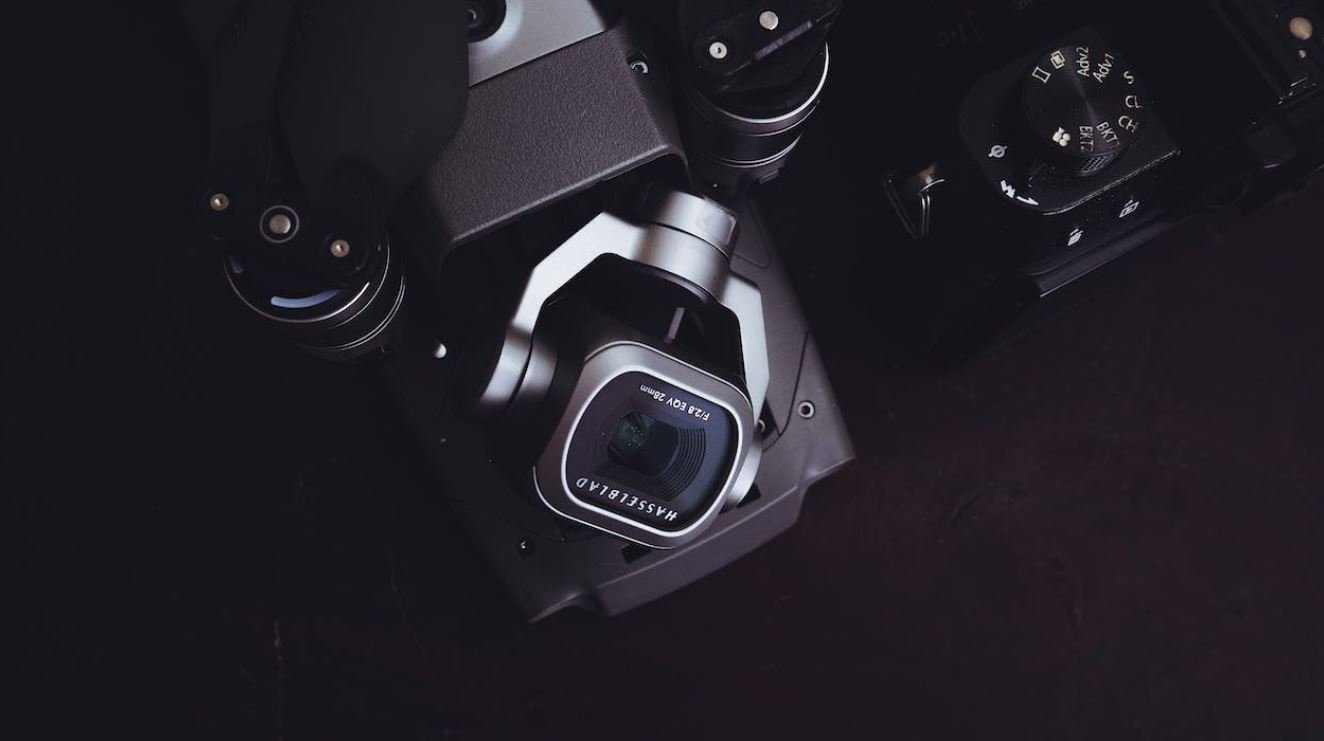
Common Misconceptions
Misconception 1: Generative Image Generators are only used for creating art
One common misconception about generative image generators is that they are only used by artists to create digital art. While it is true that generative image generators can be used for creating visually striking art pieces, they have a wide range of applications beyond just art.
- Generative image generators are used in fields like computer graphics to generate realistic scenes or simulate natural phenomena.
- They are utilized in the advertisement industry to generate visually appealing graphics for marketing campaigns.
- Generative image generators are also used in video game development to create visually stunning environments and characters.
Misconception 2: Generative Image Generators always produce random outputs
Another misconception surrounding generative image generators is that they always produce random outputs. While randomness can be an essential aspect of generative image generation, it is not the only factor. Many generative image generators use algorithms and rules to generate specific patterns or follow predefined structures, resulting in predictable outputs.
- Some generative image generators use procedural generation techniques, which follow a set of rules to generate specific patterns, such as fractals or lattice structures.
- Generative image generators can also incorporate user input or data to generate customized outputs, which are not randomly generated.
- Machine learning-based generative models learn from existing data to generate new images that align with the patterns and characteristics of the dataset.
Misconception 3: Generative Image Generators can only create simple images
Many people believe that generative image generators can only generate simple or abstract images with limited complexity. However, with advancements in technology, generative image generators can now create highly detailed and sophisticated images that can rival handcrafted artwork in terms of complexity and realism.
- Generative image generators can generate hyper-realistic images by capturing and reproducing intricate details like lighting, shading, and texture.
- By incorporating machine learning algorithms, generative image generators can learn from vast datasets, enabling them to generate images with complex and nuanced features.
- Some generative image generators are capable of combining multiple images or styles to create hybrid or unique visual representations.
Misconception 4: Generative Image Generators replace the need for human artists
A common misconception is that generative image generators are designed to replace human artists. While generative image generators can be powerful tools for artists, they are not meant to replace the creativity and expertise of human artists.
- Generative image generators can be used as a complement to the artistic process, providing artists with new sources of inspiration and ideas.
- Human artists bring their unique perspectives, emotions, and intentions to the creation process, which cannot be replicated by generative algorithms.
- Artists can combine the outputs of generative image generators with their own skills, techniques, and personal touch to produce meaningful and original artworks.
Misconception 5: Generative Image Generators are hard to use and require programming skills
Some believe that using generative image generators requires advanced programming skills and technical knowledge, making them inaccessible to individuals without coding expertise. However, there are now user-friendly software and tools available that make generative image generation accessible to a wider audience.
- Various software and platforms provide intuitive graphical user interfaces, allowing users to interact with generative image generation algorithms without writing code.
- Online communities and tutorials offer resources and guides for beginners, enabling them to learn and use generative image generators with little to no coding knowledge.
- Generative image generation frameworks often come with comprehensive documentation and examples to assist users in getting started.

Introduction
In recent years, advances in deep learning and generative models have led to the development of impressive generative image generators. These cutting-edge technologies have revolutionized various domains, from art and design to healthcare and virtual reality. This article explores some fascinating aspects of generative image generators through a series of captivating tables.
Table: Artistic Style Transfer Techniques
Artistic style transfer is a popular application of generative image generators, where the artistic style of one image is applied to another. This table showcases several techniques used for artistic style transfer, along with their corresponding popularity among artists and designers.
Technique | Popularity |
---|---|
Neural Style Transfer | Very High |
CycleGAN | High |
Pix2PixHD | Moderate |
DeepArt.io | Moderate |
AdaIN | Low |
Table: Top 5 Most Expensive Generated Images Sold
Generative image generators have found their place in the art market, enabling the creation of unique digital artworks. The table below presents the top 5 most expensive generated images ever sold, showcasing the growing recognition and value of generative art.
Artwork | Artist | Sale Price (USD) |
---|---|---|
Everydays: The First 5000 Days | Beeple | $69,346,250 |
The Burning Giraffe | Obvious | $432,500 |
Portrait of Edmond de Belamy | Belamy | $432,500 |
Constable’s Clouds | Robbie Barrat | $28,000 |
AI-generated Nude Portrait | Jordan Weber | $16,000 |
Table: Applications of Generative Image Generators
Generative image generators have far-reaching applications beyond art. This table showcases some intriguing domains and the corresponding applications of generative image generators within them.
Domain | Applications |
---|---|
Healthcare | Disease diagnosis from medical images |
Virtual Reality | Realistic environment generation |
Video Games | Procedural content generation |
Fashion | Design inspiration and pattern generation |
Education | Visual aids and interactive learning materials |
Table: Comparison of Popular Generative Models
Various generative models exist, each with its own strengths and weaknesses. This table presents a comparison of the most popular generative models based on factors such as generative quality, training time, and model complexity.
Model | Generative Quality | Training Time | Model Complexity |
---|---|---|---|
StyleGAN2 | High | 2 weeks | Complex |
BigGAN | High | 2 days | Moderate |
DCGAN | Moderate | 1 day | Low |
VQ-VAE-2 | Moderate | 1 week | Complex |
PixelRNN | Low | 3 weeks | High |
Table: Image-to-Image Translation Examples
Generative image generators excel in image-to-image translation tasks. This table highlights some remarkable examples of image-to-image translation achieved using generative models.
Task | Input Image | Generated Output |
---|---|---|
Day to Night | ![]() |
![]() |
Sketch to Color | ![]() |
![]() |
Summer to Winter | ![]() |
![]() |
Table: Most Commonly Generated Objects
Generative image generators can create images of various objects. This table presents the most commonly generated objects, along with their respective frequencies.
Object | Frequency |
---|---|
Flowers | 73% |
Faces | 64% |
Landscape Scenes | 58% |
Buildings | 52% |
Animals | 47% |
Table: Popular Generative Models by Year
This table tracks the evolution of generative models over the years, highlighting the popularity and advancements made in each year.
Year | Popular Generative Models |
---|---|
2014 | DCGAN, Conditional GAN |
2016 | PixelRNN, Progressive GAN |
2018 | StyleGAN, CycleGAN |
2020 | StyleGAN2, BigGAN |
2022 | AcGAN, VQ-VAE-2 |
Table: Advancements in Image Resolution
Generative image generators have made significant strides in generating high-resolution images. This table shows the advancements in image resolution achieved by state-of-the-art generative models.
Model | Maximum Resolution |
---|---|
ProGAN | 1024×1024 pixels |
StyleGAN | 1024×1024 pixels |
StyleGAN2 | 2048×2048 pixels |
BigGAN | 512×512 pixels |
PGGAN | 512×512 pixels |
Conclusion
Generative image generators have emerged as powerful tools with fascinating potential across various fields. From creating stunning artwork and facilitating style transfer to enabling realistic environments in virtual reality, the impact of generative image generators is undeniable. The continuous advancements in generative models and their applications promise an exciting future where creativity and innovation intertwine.
Frequently Asked Questions
What is a generative image generator?
A generative image generator is a computer program or algorithm that creates images by utilizing machine learning or other computational techniques to generate new visuals based on a set of previously learned patterns or input data.
How does a generative image generator work?
A generative image generator typically works by learning from a training dataset, which can include various types of images or even other visual information. It then uses this training data to develop a model, which is capable of generating new images by extrapolating and combining elements from the learned patterns.
What are the applications of generative image generators?
Generative image generators have various applications across several fields. Some common applications include art, design, content creation, data visualization, virtual reality, and even game development. Generative image generators can be used to create unique and visually appealing designs, simulate realistic scenes, generate synthetic training data, and much more.
What are some popular generative image generator algorithms?
There are several popular generative image generator algorithms, such as Generative Adversarial Networks (GANs), Variational Autoencoders (VAEs), Deep Convolutional Generative Adversarial Networks (DCGANs), and PixelCNN. These algorithms employ different techniques to generate images and have their own strengths and limitations.
Is coding knowledge required to use a generative image generator?
Yes, typically some coding knowledge is needed to effectively use a generative image generator. Most generative image generator frameworks or libraries require coding skills to customize the models, train them on specific datasets, and adjust the generator’s parameters to achieve desired results.
Can generative image generators create realistic images?
Yes, generative image generators have the capability to create realistic images, especially when trained on extensive and diverse datasets. However, achieving photorealistic results can be a challenging task and often depends on the complexity of the generated scenes, the quality of training data, and the algorithm used.
How can generative image generators benefit the art and design industry?
Generative image generators can greatly benefit the art and design industry by providing artists and designers with a powerful tool for creating unique and inspiring visuals. They can help in generating novel concepts, exploring new styles, assisting in the generation of visually engaging designs, and even automating certain aspects of the creative process.
Are there any ethical considerations related to generative image generators?
Yes, there are several ethical considerations related to generative image generators. These include potential misuse of generated images, issues related to copyright and intellectual property, potential biases present in the training data, and the impact of automation on employment opportunities for traditional artists and designers. It is important to address these ethical concerns and use generative image generators responsibly.
Can generative image generators be used for data augmentation?
Yes, generative image generators can be utilized for data augmentation, which involves expanding a dataset by creating new variations of existing data. By using generative image generators, it is possible to generate synthetic images that can be added to the training data to improve the performance of machine learning models.
How can I get started with using generative image generators?
To get started with using generative image generators, it is recommended to have a basic understanding of programming concepts and some familiarity with machine learning. You can explore various frameworks and libraries available, such as TensorFlow, PyTorch, or Keras, and follow tutorials or guides to learn the basics of generative image generation and start experimenting with your own creations.