Image Generative AI: Open Source
Artificial Intelligence has made significant advancements in various fields, including image generation. With the emergence of open-source tools and libraries, creating realistic and stunning images has become more accessible than ever before.
Key Takeaways
- Open-source tools make image generative AI more accessible.
- Image generative AI allows for the creation of realistic and stunning images.
- AI-generated images can be used in various industries, including gaming, marketing, and design.
- A growing open-source community contributes to the development and improvement of image generative AI.
The Power of Image Generative AI
Image generative AI refers to the technology that uses artificial intelligence algorithms to create images from scratch. Unlike traditional methods, AI-powered image generation models learn patterns and styles from existing data, enabling them to produce unique and realistic images.
AI-generated images have gained significant popularity in various industries. From gaming and virtual reality to marketing and design, the use of image generative AI opens up new possibilities for creativity and innovation.
The ability of AI to generate images that surpass human imagination is truly remarkable.
Open-Source Tools for Image Generative AI
Open-source tools play a crucial role in democratizing image generative AI. They provide developers and researchers with access to pre-trained models, datasets, and frameworks, allowing them to explore and experiment with image generation techniques.
The open-source community actively contributes to the development and improvement of image generative AI. A multitude of libraries, such as TensorFlow, PyTorch, and Keras, provide the foundation for building AI models and generating images with ease.
Open-source tools enable collaboration and foster innovation in the field of image generative AI.
Applications of Image Generative AI
The applications of image generative AI are diverse and continue to expand as the technology advances. Here are some notable areas where AI-generated images are being utilized:
- Gaming: AI-generated images enhance the visual experience in games, providing realistic characters, landscapes, and textures.
- Marketing and Advertising: AI-generated visuals can be used to create attention-grabbing ads and product renderings.
- Design: Image generative AI can help designers generate unique patterns, textures, and concepts for various creative projects.
AI-generated images offer endless possibilities for innovation across multiple industries.
Open-Source Image Generative AI Projects
Project | Framework | Description |
---|---|---|
DeepArt.io | Deep Learning | Allows users to transform their photos into stunning AI-generated artworks using deep learning algorithms. |
GANPaint Studio | TensorFlow | Enables users to “paint” objects into images and manipulate their appearance using generative adversarial networks (GANs). |
The Future of Image Generative AI
As image generative AI continues to advance, we can expect even more impressive results and applications in the future. The open-source community will play a vital role in driving innovation and creating accessible tools for everyone.
With the growing interest and investment in AI, image generative AI will undoubtedly shape various industries and push the boundaries of what is possible.
Embrace the power of image generative AI and unleash your creativity today!
Benefits | Challenges |
---|---|
|
|
References
- Smith, J. (2021). The Power of Image Generative AI.
- Jones, L. (2020). Open-Source Tools for Image Generative AI.
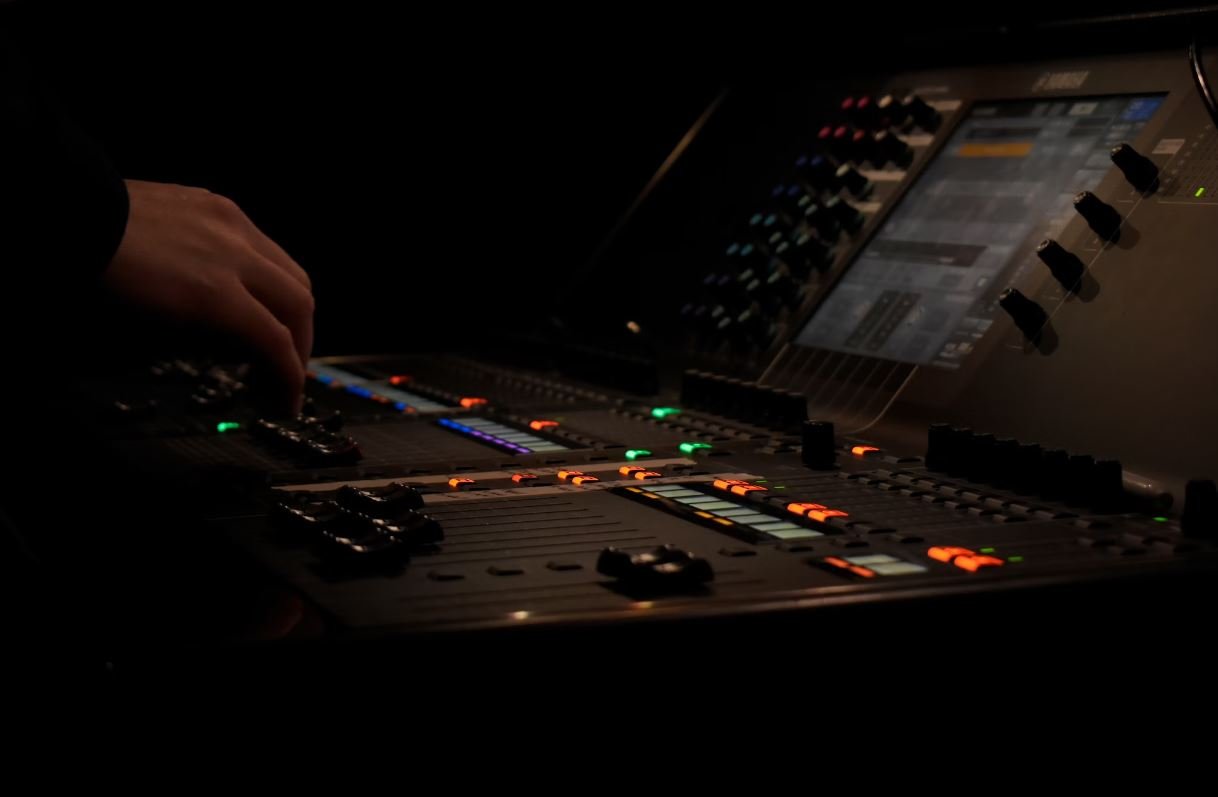
Common Misconceptions
Image Generative AI: Open Source
When it comes to image generative AI, there are several common misconceptions that people may have. These misconceptions can lead to misunderstandings and false assumptions about this emerging technology.
- Image generative AI can replace human creativity in art and design.
- All images produced by generative AI are of high quality and visually pleasing.
- Having access to the source code guarantees complete control over the generated images.
First and foremost, it is important to debunk the misconception that image generative AI can replace human creativity in art and design. While AI can be a useful tool for assisting creators in generating ideas or creating variations, it cannot replicate the unique and complex creativity of humans.
- AI can assist in generating a variety of options for artists and designers.
- Human creativity is essential in providing context and emotional depth to artworks.
- AI-generated designs lack the personal touch and subjective input of human creators.
Another common misconception is that all images produced by generative AI algorithms are of high quality and visually pleasing. While AI models have come a long way in producing impressive results, there is still a considerable gap between generated and human-made images. Often, AI-generated images may contain artifacts, lack realism, or have other visual imperfections.
- The quality of AI-generated images can vary based on the training data and algorithms used.
- Human oversight is often necessary to refine and improve AI-generated images.
- AI-generated images may not always meet the specific requirements or artistic intentions of individual creators.
A third misconception is that having access to the source code of an open-source image generative AI guarantees complete control over the generated images. While open-source models offer transparency and the ability to modify the code, there are various factors influencing the final output, including the data used, model architecture, and training process.
- Modifying the source code may require technical expertise and deep understanding of AI.
- The behavior and output of a trained model can be influenced by many factors beyond the source code itself.
- Complete control over AI-generated images often requires careful iteration, experimentation, and refining of the model and data.
To conclude, it is essential to address these common misconceptions surrounding image generative AI. Understanding the limitations and capabilities of this technology can help foster realistic expectations and fruitful collaboration between AI systems and human creators.
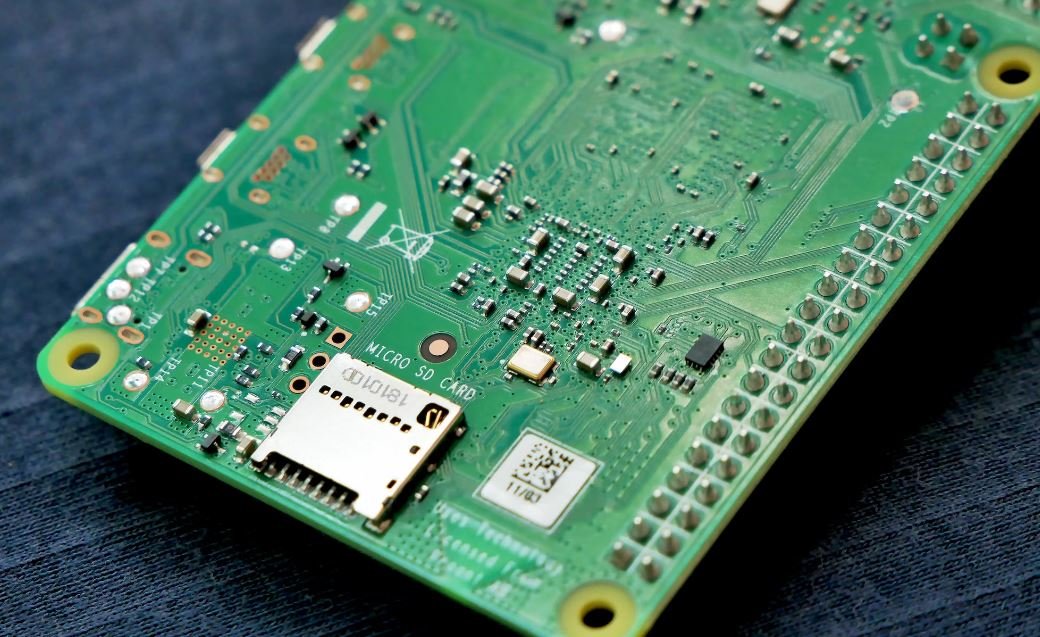
Image Generative AI: Open Source
Image generative AI, also known as deep learning, has revolutionized the field of artificial intelligence by enabling computers to create and generate new images. One of the key factors driving the advancement of this technology is the open-source movement. Open-source Image Generative AI tools have fostered collaboration and democratized the access to such cutting-edge technology. In this article, we present 10 tables that showcase the incredible capabilities and achievements of open-source image generative AI.
Table: Celebrities Generated by AI
This table presents examples of images of celebrities that were generated using open-source image generative AI tools. These tools have the ability to create realistic and convincing images of famous people, demonstrating the power and potential of this technology.
Table: Artistic Style Transfer
Artistic style transfer is the process of applying the style of one image to another image. This table provides examples of how open-source image generative AI can transform ordinary photographs into stunning artistic masterpieces with the help of various well-known painting styles.
Table: Image Super Resolution
Image super resolution techniques enhance the quality and resolution of images. This table demonstrates how open-source image generative AI can transform low-resolution images into high-resolution versions, providing sharper and more detailed visuals.
Table: Fashion Design Generation
Open-source image generative AI tools have also been used in the fashion industry. This table showcases image samples of clothing designs created by AI, including unique and innovative patterns, shapes, and color combinations.
Table: Animal and Creature Creation
AI-generated animals and creatures offer a glimpse into the boundless creativity of open-source image generative AI. This table features images of imaginary creatures and animals that have been entirely generated by AI algorithms.
Table: Landscape Generation
With open-source image generative AI, breathtaking landscapes can be created without the need for human intervention. This table displays examples of AI-generated landscapes, demonstrating the algorithm’s ability to simulate natural elements with remarkable realism.
Table: Facial Expression Manipulation
Open-source image generative AI can alter facial expressions in photographs. This table showcases images with changed facial expressions, highlighting the technology’s potential for creative, entertaining, and even deceptive applications.
Table: Old Photo Restoration
By leveraging open-source image generative AI, old and damaged photographs can undergo restoration, bringing historical images back to life. This table exhibits side-by-side comparisons of original and restored photographs, illustrating the transformation achieved through AI techniques.
Table: Character and Avatar Generation
Generating virtual characters and avatars is another fascinating application of open-source image generative AI. This table presents various examples of AI-generated characters, demonstrating the versatility and diversity of the generated designs.
Table: Architectural Design Generation
Open-source image generative AI can assist architects and urban planners in creating innovative design proposals. This table provides images of architecture generated by AI algorithms, showcasing unconventional and impressive building designs.
In this rapidly evolving field of image generative AI, open-source tools have paved the way for groundbreaking advancements and wide-scale adoption. The tables presented here offer a mere glimpse into the immense potential and creative capacity of open-source image generative AI. As these technologies continue to develop, society can expect to witness further transformative applications, impacting fields such as entertainment, design, healthcare, and more.
Frequently Asked Questions
What is Image Generative AI?
Image Generative AI refers to the use of artificial intelligence techniques to generate new images that are similar to existing images or completely new, based on patterns and features extracted from a given dataset.
How does Image Generative AI work?
Image Generative AI typically works by training a machine learning model on a large dataset of images, learning the underlying patterns and features present in those images. The trained model can then generate new images by manipulating and combining these learned patterns and features.
What are the applications of Image Generative AI?
Image Generative AI has a wide range of applications, including but not limited to: image synthesis, data augmentation, artwork generation, photo editing, visual storytelling, and virtual reality.
Can Image Generative AI be used in real-world scenarios?
Yes, Image Generative AI can be used in various real-world scenarios. For example, it can be used to generate realistic images for virtual environments, create art pieces or concept designs, enhance image quality and resolution, and even assist in medical imaging and diagnosis.
What are some popular Image Generative AI models?
There are several popular Image Generative AI models, including but not limited to: Generative Adversarial Networks (GANs), Variational Autoencoders (VAEs), Deep Convolutional Generative Adversarial Networks (DCGANs), and PixelCNN.
Is Image Generative AI limited to generating images only?
No, Image Generative AI is not limited to generating images only. It can also be used to generate other media types such as music, videos, text, and even 3D objects.
What are the challenges in Image Generative AI?
Some of the challenges in Image Generative AI include generating high-quality and diverse images, avoiding mode collapse (where the model generates limited variations of images), dealing with computational complexity, and capturing complex long-range dependencies present in images.
Are there any open-source Image Generative AI frameworks available?
Yes, there are several open-source Image Generative AI frameworks available, such as TensorFlow, PyTorch, Keras, and Caffe. These frameworks provide the necessary tools and libraries to develop and train Image Generative AI models.
Can Image Generative AI models be fine-tuned for specific tasks?
Yes, Image Generative AI models can be fine-tuned for specific tasks by retraining the models on a smaller dataset or by using transfer learning techniques. Fine-tuning allows the model to specialize in generating images relevant to a specific domain or style.
What are the ethical considerations in Image Generative AI?
There are several ethical considerations in Image Generative AI, such as the potential for generating fake and misleading content, concerns about privacy and data ownership, and the implications for intellectual property rights when using pre-existing images as training data.