How Does Generative Image AI Work?
Generative Image AI refers to the use of artificial intelligence algorithms to generate new images based on existing ones. It has become an increasingly popular field in recent years, with applications ranging from art and design to healthcare and entertainment.
Key Takeaways
- Generative Image AI uses artificial intelligence algorithms to create new images based on existing ones.
- It can be used across various industries, including art, design, healthcare, and entertainment.
- Generative Image AI works by utilizing deep learning models to understand patterns and generate new content.
- GANs (Generative Adversarial Networks) are commonly used for generative image tasks.
- Training data plays a crucial role in the success and quality of generative image AI models.
At the heart of generative image AI is the use of deep learning models, specifically **Convolutional Neural Networks (CNNs)**. These networks are designed to understand visual patterns and representations, allowing them to generate new content that aligns with the learned patterns. By leveraging CNNs, generative image AI algorithms can create realistic and visually pleasing images.
One of the key techniques used in generative image AI is the application of **Generative Adversarial Networks (GANs)**. GANs consist of two main components: a generator and a discriminator. The generator takes random noise as input and tries to generate convincing images, while the discriminator’s role is to differentiate between real and generated images. *The generator and discriminator engage in a competitive process, where the generator continually improves its ability to generate realistic images, fooling the discriminator.*
Generating high-quality images requires large amounts of training data. *The more diverse and representative the training data, the better the generative image AI model can generalize and produce quality outputs.* However, collecting and labeling large datasets can be a challenging and time-consuming task. To mitigate this, transfer learning techniques are often applied, where pre-trained models on similar tasks or domains can be fine-tuned to generate new images.
Generative Image AI in Practice
Generative Image AI has found numerous applications in various industries. It has been used for:
- Art and Design: Creating unique and artistic pieces based on existing artwork or styles.
- Healthcare: Generating synthetic medical images for training and research purposes.
- Entertainment: Enhancing video game graphics and creating visually stunning effects.
Examples of Generative Image AI Output
Table 1 showcases some examples of generative image AI output in different domains:
Application | Example Output |
---|---|
Art and Design | ![]() |
Healthcare | ![]() |
Entertainment | ![]() |
The Future of Generative Image AI
Generative Image AI has already showcased its potential in various domains and will continue to advance in the coming years. With improvements in deep learning models, data availability, and computational power, we can expect even more impressive and realistic generative image AI applications in the future.
*As generative image AI continues to evolve, it has the potential to revolutionize how we create, design, and interact with visual content.* Its impact will be felt across industries, from art and entertainment to healthcare and beyond.
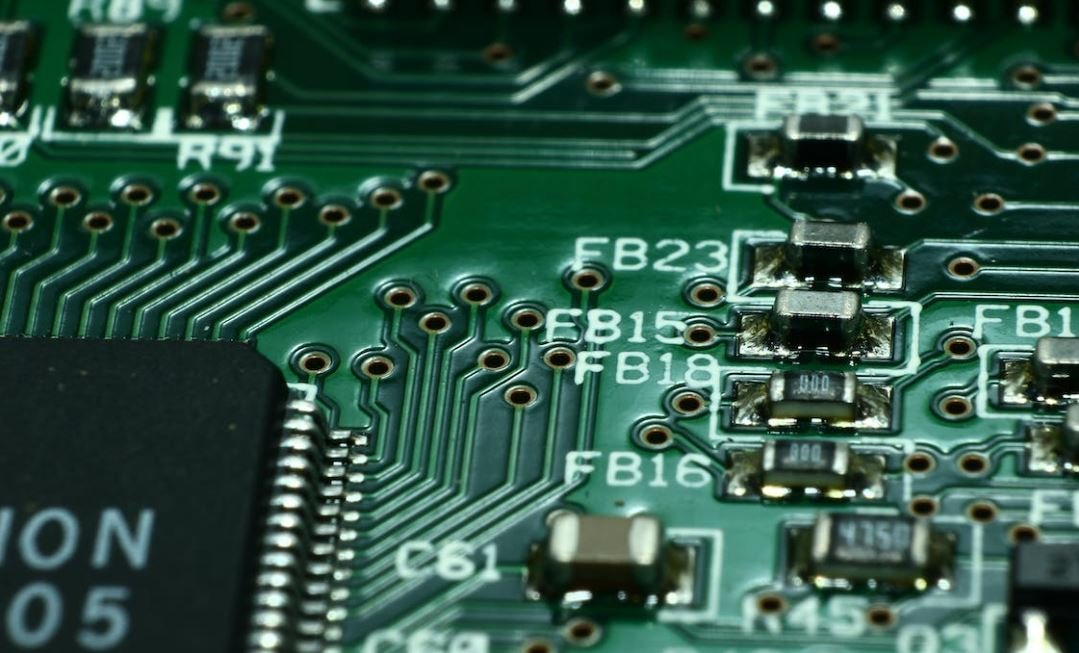
Common Misconceptions
Generative Image AI is Just Like Photoshop
One common misconception is that generative image AI works in a similar way to Photoshop. While both tools manipulate images, they function differently. Generative image AI uses deep learning algorithms to analyze and understand patterns in images, while Photoshop is a software that allows users to manually edit images.
- Generative image AI relies on artificial intelligence algorithms.
- Photoshop is a tool that requires user input.
- Generative image AI creates original images, while Photoshop primarily edits existing ones.
Generative Image AI Can Create Realistic Images
Many people believe that generative image AI can always generate highly realistic or indistinguishable images from real photographs. While generative image AI has made significant advancements, it is still not perfect at creating completely realistic images. The generated images may have certain flaws or imperfections that can give them away as machine-generated.
- Generative image AI can produce impressive results, but not always perfectly realistic.
- Generated images may have subtle clues that they are not real photographs.
- The AI’s ability to create realistic images depends on various factors, including the quality of training data.
Generative Image AI Can Only Generate Images
Another misconception is that generative image AI can only generate static images. In reality, generative image AI can generate much more than just images. It can create animations, generate textures, produce 3D models, and even generate music or text. Generative image AI is a versatile tool that can be applied to various creative applications.
- Generative image AI has a wider scope of capabilities beyond static image generation.
- It can create animations, textures, and 3D models.
- Generative image AI can also be used in generating music or text using similar principles.
Generative Image AI is Easy to Use and Requires No Expertise
Many people assume that generative image AI is easy to use and requires no specialized knowledge or expertise. However, working with generative image AI often requires a deep understanding of machine learning concepts and programming skills. It takes time and effort to train the AI models, optimize parameters, and ensure the results meet the desired objectives.
- Using generative image AI often requires expertise in machine learning.
- Training and optimizing AI models can be a complex process.
- Achieving the desired results with generative image AI requires expertise and experimentation.
Generative Image AI is a Threat to Human Creativity
Some people believe that generative image AI will replace human creatives and diminish the role of human imagination. While generative image AI can automate certain creative tasks, it does not eliminate human creativity. Instead, it can be seen as a tool that complements and enhances human creativity by assisting in the creative process and providing new avenues for exploration.
- Generative image AI is a tool that can assist human creatives.
- It can provide new possibilities for artistic expression and exploration.
- Human imagination and creativity remain crucial in leveraging generative image AI effectively.
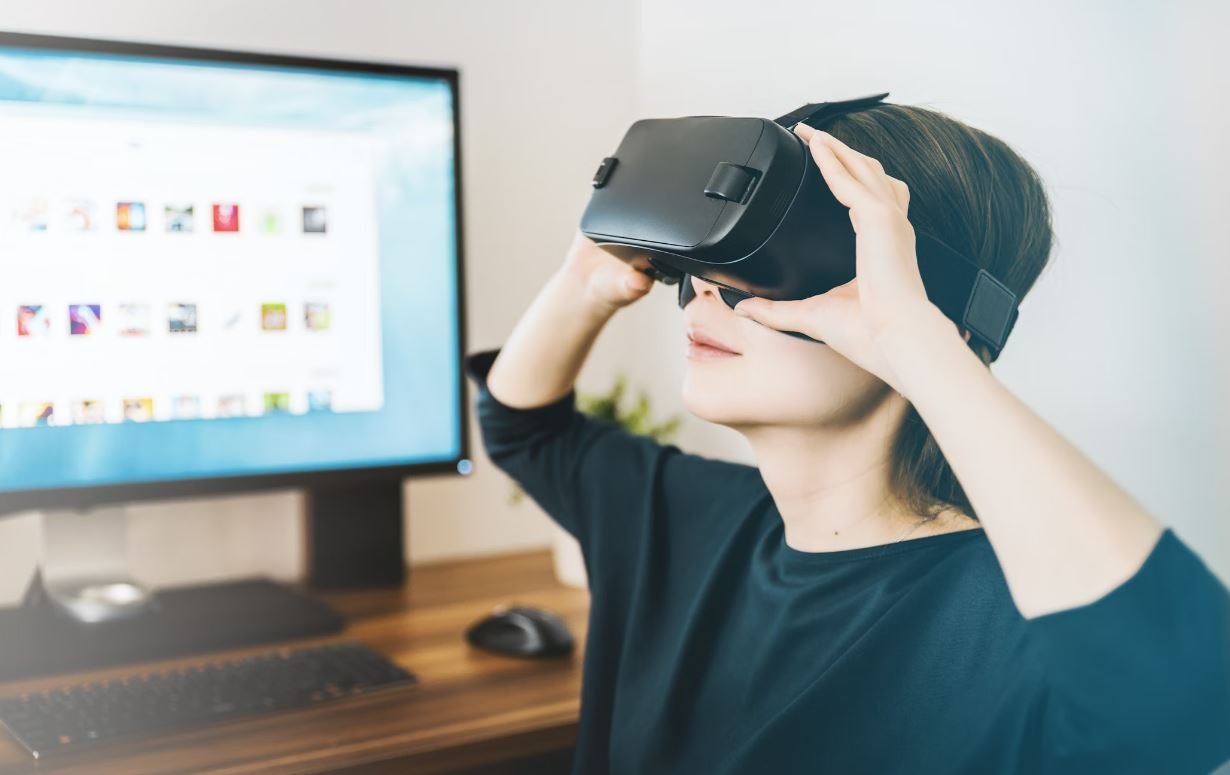
Defining Generative Image AI
Generative Image AI refers to the technology that uses machine learning algorithms to produce realistic and creative images. This innovative development has revolutionized various industries, including art, design, and advertising. Below are 10 fascinating examples showcasing the intriguing capabilities of Generative Image AI.
1. “Starry Night” – Van Gogh’s Famous Artwork Reimagined
This AI algorithm generates a unique interpretation of Van Gogh’s masterpiece “Starry Night,” transforming it into a mesmerizing blend of vibrant colors and abstract patterns. The dream-like ambiance created by this generative image AI captivates the viewer, revealing new dimensions of Van Gogh’s artistic vision.
2. Replicating Famous Faces
Imagine being able to witness how iconic personalities from history would look today. With generative image AI, this becomes possible. This table features side-by-side comparisons of historical figures such as Leonardo da Vinci, Cleopatra, and Albert Einstein as they might appear in the present day – a fascinating glimpse into the fusion of past and present.
3. “The Nature Symphony” – Multisensory Experience
This generative image AI project brings together visual and audio elements to create a captivating multisensory experience. By analyzing various aspects of nature, such as landscapes, flora, and fauna, the algorithm composes a synchronized symphony that complements the visual imagery, immersing the viewer in a whole new artistic dimension.
4. Unlocking the Mystery of Lost Cities
Generative image AI can recreate and visualize ancient lost cities based on historical records and archaeological findings. By processing available data, this technology constructs detailed digital reconstructions, shedding light on civilizations long forgotten. Explore the wonders of ancient wonders like Atlantis and Machu Picchu.
5. Fashion Forward – AI Fashion Design
Designers and fashion enthusiasts can leverage generative image AI to push the boundaries of creativity. This table showcases a series of AI-generated clothing designs that blend classic styles with futuristic elements, creating avant-garde fashion statements that inspire and challenge conventional concepts of style.
6. Dreamscapes – Surreal Landscapes from Imagination
Step into surreal dreamscapes with the help of generative image AI. This table presents a collection of otherworldly landscapes that seem plucked from the depths of imagination. Eerie settings, floating islands, and gravity-defying structures coalesce to create a stunning visual journey into the realm of the impossible.
7. Restoring Ancient Artifacts
Generative image AI can be invaluable in the restoration of ancient art pieces. This table demonstrates how the technology can repair and enhance faded, damaged, or incomplete artifacts, such as ancient sculptures and paintings. With AI assistance, the beauty of these historical treasures is revived, bringing them closer to their original splendor.
8. Visualizing Music – Sonic Melodies
This project explores the interaction between music and visual art. By analyzing sound frequencies and patterns, generative image AI creates captivating visual representations of various musical compositions. This table presents a visual journey into the harmonic realm, where melodies take form and rhythm becomes color.
9. Architectural Marvels Beyond Reality
Unleash architectural creativity with the aid of generative image AI. This table showcases architectural designs that surpass the boundaries of reality, combining futuristic aesthetics with functional elements. These imaginative structures redefine our perception of space, offering a glimpse into the bold possibilities of the future.
10. Virtual Passages – Time Travel through Photography
Generative image AI enables us to travel through time by reconstructing historical moments. This table features a series of AI-generated images that revive iconic events, such as the moon landing and the signing of historical treaties. Immerse yourself in these lifelike glimpses of the past, bridging the gap between generations.
Generative image AI has opened up remarkable creative avenues, transforming the fields of art, design, music, and archaeology. These tables offer a mere glimpse into the expansive capabilities of this technology, showcasing its ability to reimagine the past, envision the future, and push the boundaries of human creativity.
Frequently Asked Questions
How does generative image AI work?
What is generative image AI?
How does generative image AI learn?
What are the applications of generative image AI?
How does generative image AI handle style transfer?
What are the challenges in generative image AI?
Can generative image AI be used for data augmentation?
How does generative image AI assist in image restoration?
Can generative image AI generate entirely new images?
Why is generative image AI important?
Are there any limitations to generative image AI?