How Does Generative AI Work?
Generative AI, a branch of artificial intelligence, focuses on creating new content by training models on large amounts of existing data. This technology has gained significant attention in recent years due to its potential to create human-like and creative outputs. Understanding how generative AI works can provide insights into the future of AI-generated content and its applications.
Key Takeaways
- Generative AI uses large datasets to learn patterns and generate new content.
- It relies on algorithms such as Generative Adversarial Networks (GAN) and Variational Autoencoders (VAE).
- Generative AI can create various forms of content, including images, music, and text.
- Ethical and legal concerns surround the use of generative AI, particularly in deepfake technology.
- The future potential of generative AI extends to fields like art, entertainment, and design.
In generative AI, models are trained on vast amounts of data to recognize and understand patterns. By analyzing the features and structures present in the data, **the models are able to generate new content that is similar to the examples provided during training**. This process allows AI to produce original content that resembles the style, composition, and characteristics of the training dataset.
Understanding Generative Models
Generative models are the core of generative AI. These models employ various algorithms to generate new content based on the patterns and features learned from the training data. Two commonly used algorithms in generative AI are:
- Generative Adversarial Networks (GAN): GANs consist of two main components – the generator and the discriminator. The generator generates new content, while the discriminator evaluates the generated content by comparing it with the real data. Through an iterative process, both components learn and improve their performance, leading to the creation of more realistic and high-quality outputs.
- Variational Autoencoders (VAE): VAEs are another widely used algorithm in generative AI. They work by encoding input data into a lower-dimensional representation called a latent space. This latent space can then be used to generate new content by decoding it back into the original data format. VAEs enable the generation of diverse and novel outputs by manipulating the latent space.
With generative AI, the possibilities are endless. It can create a wide range of content types, including:
- Artistic images and paintings
- Music compositions and melodies
- Texts, stories, and articles
- Video game characters and environments
- Product designs and architectural concepts
Generative AI has the potential to revolutionize various industries by assisting human creators or even functioning autonomously in certain cases. However, it also poses ethical and legal challenges, particularly in the area of **deepfake technology**. Deepfakes refer to manipulated or fabricated media, such as videos or images, that appear real. This raises concerns about the spread of misinformation and the potential use of deepfakes for malicious purposes.
Applications of Generative AI
Generative AI is being actively explored and utilized in numerous fields:
Field | Applications |
Art | Creating unique and original artwork, assisting artists in the creative process. |
Entertainment | Generating realistic characters, special effects, and immersive gaming experiences. |
Furthermore, **the potential of generative AI extends to fields like design and architecture**, where it can assist in generating novel concepts and prototypes. The ability to quickly generate multiple design options based on specified criteria can significantly speed up the creative process and increase innovation.
Challenges and Future Perspectives
- Regulating the use of generative AI to address concerns regarding deepfakes and intellectual property rights.
- Ensuring transparency and explainability of generative AI systems to build trust with users and stakeholders.
- Advancing research and development to improve the quality and diversity of generative AI outputs.
Generative AI is an exciting field with vast potential, but it also comes with challenges that need to be addressed. By responsibly harnessing its capabilities and overcoming these obstacles, we can unlock new frontiers in creative technology and shape a future where generative AI drives innovation across various industries.
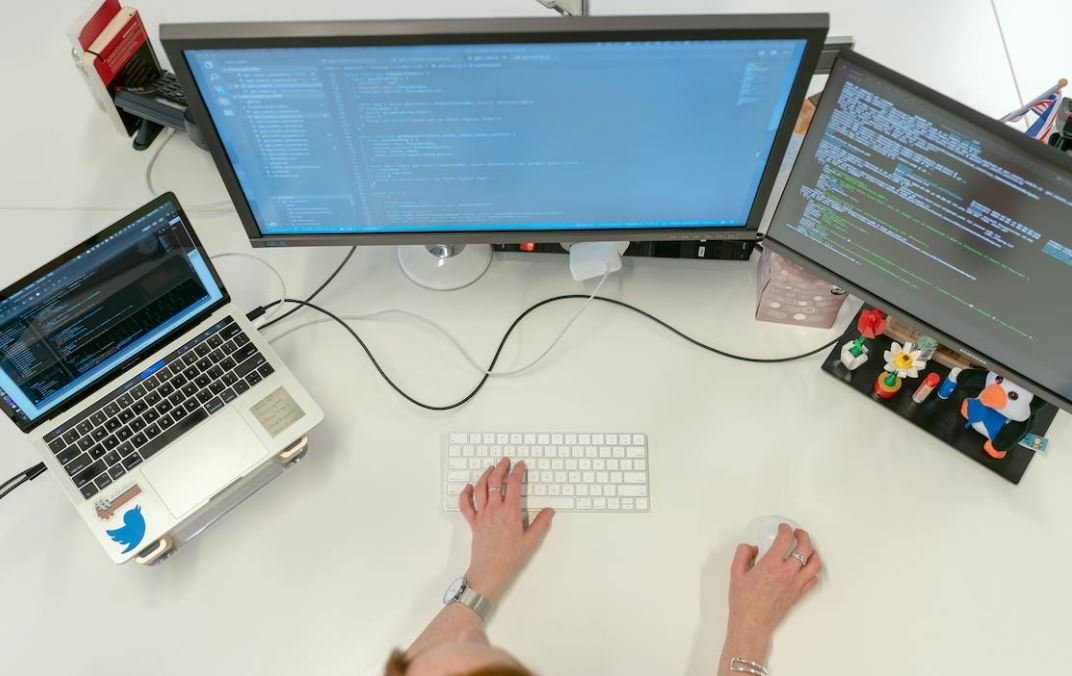
Common Misconceptions
Misconception 1: Generative AI Can Think Like Humans
One common misconception about generative AI is that it can think and reason like humans. However, this is not true. Generative AI algorithms, such as GANs (Generative Adversarial Networks), are designed to learn patterns and generate new data based on those patterns. They don’t possess consciousness or intelligence. Here are three relevant bullet points:
- Generative AI doesn’t have emotions or subjective experiences.
- It cannot understand context or interpret meaning in the same way humans do.
- Generative AI works by manipulating mathematical representations of data, rather than having thoughts or intentions.
Misconception 2: Generative AI Is Always Perfect
Another misconception is that generative AI always produces perfect and flawless output. While generative AI can create impressive and realistic content, it is not infallible. Here are three relevant bullet points:
- Generative AI relies on the quality and quantity of training data it has been fed.
- It can produce errors or inconsistencies in the generated output.
- Generative AI might also generate content that is biased or offensive if it has been trained on biased or offensive data.
Misconception 3: Generative AI Can Fully Replace Human Creativity
Some people believe that generative AI has the potential to replace human creativity entirely. While generative AI can assist and enhance creative processes, it is not a substitute for human creativity. Here are three relevant bullet points:
- Generative AI is limited to what it has been trained on and cannot create entirely new concepts or ideas.
- It lacks the ability to imagine, dream, or have original thoughts.
- Human creativity involves emotions, intuition, and personal experiences that cannot be replicated by generative AI.
Misconception 4: Generative AI Poses No Ethical Concerns
There is a misconception that generative AI has no ethical concerns associated with its use. However, there are several ethical dilemmas and considerations when working with generative AI technology. Here are three relevant bullet points:
- Generative AI can be used for malicious purposes, such as generating fake news or deepfake videos.
- It can perpetuate biases present in the training data, leading to biased outputs.
- Using generative AI without transparency or responsible data practices can raise concerns around privacy and consent.
Misconception 5: Generative AI Will Replace Human Jobs
Many people fear that generative AI will result in widespread job loss and unemployment. While it can automate certain tasks, generative AI is unlikely to fully replace human jobs. Here are three relevant bullet points:
- Generative AI is more likely to be used as a tool to augment human capabilities rather than replace human workers.
- Human skills such as critical thinking, judgment, and creativity are still essential in many fields.
- Generative AI can create new job opportunities, such as AI trainers and explainability experts.
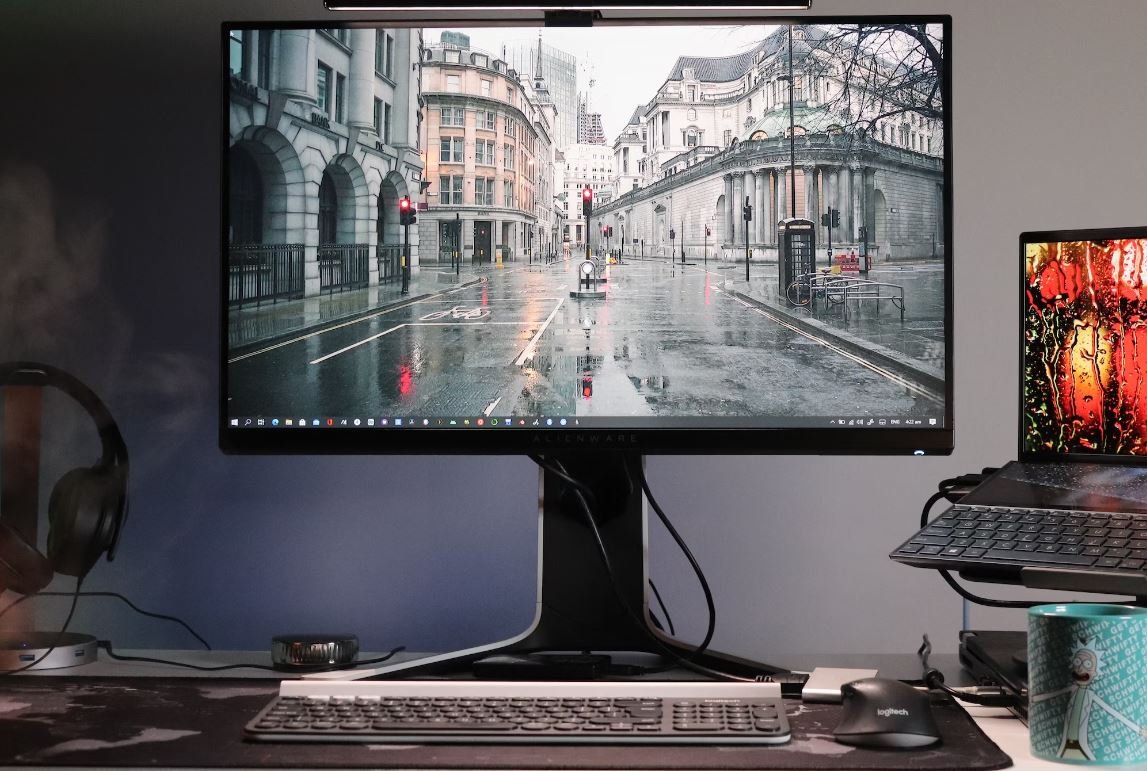
Introduction
In recent years, there has been a surge in the development and use of generative AI, a field of artificial intelligence that aims to create computer systems capable of producing new content or information. This article delves into the inner workings of generative AI and provides a glimpse into the fascinating world of data creation, manipulation, and generation.
1. AI-Generated Paintings
Generative AI has revolutionized the art world by creating stunning and unique paintings. From abstract masterpieces to surreal landscapes, these AI-generated artworks are captivating art enthusiasts worldwide.
2. Generated Music Compositions
With generative AI, computers can compose original music compositions that span various genres. From classical symphonies to electronic beats, these AI-generated melodies showcase the endless creative potential of artificial intelligence.
3. AI-Written Poetry
Generative AI has also ventured into the realm of literature by composing beautiful poetry. From sonnets to haikus, these poetic creations demonstrate the emotion and creativity that AI can bring to written language.
4. Generated Movie Scripts
AI is now capable of creating movie scripts, unveiling unique and imaginative storylines. The scripts leverage patterns and themes from existing films to create entirely new cinematic experiences.
5. AI-Created Recipes
Generative AI can curate innovative and delicious recipes by combining ingredients and flavors in unconventional ways. These AI-generated recipes push the boundaries of culinary arts.
6. Generated Fashion Designs
From avant-garde garments to futuristic outfits, generative AI has revolutionized the world of fashion by designing unique and eye-catching clothing pieces. These AI-generated designs push the boundaries of creativity and style.
7. AI-Generated Old Masters’ Paintings
Generative AI can create paintings that emulate the styles of renowned artists from the past, replicating their brushstrokes, color palettes, and themes. These AI-generated pieces pay homage to art history’s great masters.
8. Generated Architectural Designs
Generative AI has brought innovation to architecture by creating remarkable building designs. From awe-inspiring skyscrapers to sustainable eco-friendly structures, these AI-generated designs redefine urban landscapes.
9. AI-Written News Articles
AI is now capable of writing news articles on various topics, using real-time data and information sources. These AI-generated articles demonstrate the potential of AI in delivering timely and accurate news.
10. Generated Virtual Reality Worlds
Generative AI has transformed the virtual reality (VR) experience by creating immersive and lifelike worlds. These AI-generated virtual environments offer users new and exciting adventures without limits.
Conclusion
Generative AI has made incredible strides in various creative disciplines, from art and music to literature and design. These AI-powered systems have proven their ability to generate unique and captivating content, pushing the boundaries of human creativity. As generative AI continues to advance, we can only anticipate the limitless possibilities it will bring to the realm of creation and innovation.
Frequently Asked Questions
What is generative AI?
Generative AI refers to the use of algorithms and machine learning techniques to generate data or content that is similar to the input it has been trained on. It involves creating models that can generate new examples based on patterns and structures present in the existing data.
How does generative AI work?
Generative AI works by leveraging deep learning techniques, particularly through the use of generative models such as generative adversarial networks (GANs) or variational autoencoders (VAEs). These models are trained on large datasets and learn to generate new data by capturing the underlying patterns and structures present in the training data.
What are generative models?
Generative models are a type of machine learning model that aims to generate new data samples that are similar to the training data provided to them. These models learn the statistical properties, patterns, and correlations present in the training data and use that knowledge to create new instances that resemble the original dataset.
What are generative adversarial networks (GANs)?
Generative adversarial networks (GANs) are a type of generative model that consists of two neural networks competing against each other. The generator network learns to generate new data samples, while the discriminator network learns to distinguish between real and generated data. Both networks improve their performance through iterative training until the generated samples become indistinguishable from real data.
What are variational autoencoders (VAEs)?
Variational autoencoders (VAEs) are another type of generative model that aims to learn a low-dimensional representation of the original data in an unsupervised manner. VAEs consist of an encoder network that maps the input data to latent variables, and a decoder network that reconstructs the input data from the latent space. By sampling from the learned latent space, VAEs can generate new data points.
What are the applications of generative AI?
Generative AI has a wide range of applications across various fields. It can be used for generating realistic images, synthesizing speech, creating music, designing new products, generating text or code, and even for simulating realistic environments in video games. It has also found applications in healthcare, drug discovery, and data augmentation for training machine learning models.
What are the challenges of generative AI?
Generative AI still faces several challenges. One of the main challenges is generating truly high-quality and coherent outputs consistently. Modes of failure such as mode collapse or generating unrealistic samples can hinder the effectiveness of generative models. Another challenge is the amount of training data required, as well as the computational resources needed to train and fine-tune such models.
How is generative AI different from traditional AI?
Traditional AI is focused on solving specific tasks using explicit rules and predefined algorithms. Generative AI, on the other hand, leverages machine learning to learn from data and generate new content or samples. While traditional AI is rule-based and relies on human-crafted features, generative AI is data-driven and can learn to generate new content without explicit instructions.
Can generative AI create completely original content?
Generative AI can create new content that resembles the training data it was provided with, but it cannot create completely original content that has never existed before. However, it can generate novel combinations or variations of existing content, which can still be valuable in various domains and creative applications.
What are some popular generative AI projects?
There are many popular generative AI projects that have gained attention in recent years. Some examples include DeepArt, which generates artwork in the style of famous artists, OpenAI’s GPT-3, which can generate human-like text, and DeepDream, which creates psychedelic images by modifying input images using deep neural networks. These are just a few examples of the many exciting generative AI projects being developed.