How AI Image Recognition Works
Artificial Intelligence (AI) image recognition, or computer vision, is a technology that enables machines to identify and classify images or objects based on their visual characteristics. This remarkable capability has numerous applications across industries, from autonomous vehicles to medical diagnostics to facial recognition systems. Understanding how AI image recognition works can provide insights into its potential impact on various fields.
Key Takeaways
- AI image recognition uses machine learning algorithms to analyze and interpret visual data.
- It involves the three main steps of data preprocessing, feature extraction, and classification.
- The accuracy of AI image recognition systems can vary depending on the quality and diversity of the training data.
- Advancements in deep learning networks have significantly improved the performance of image recognition models.
**AI image recognition** leverages advanced machine learning techniques to process and interpret images. *Machine learning algorithms* are trained using large datasets of labeled images to learn the patterns and visual characteristics associated with specific objects or classes. Once the models are trained, they can analyze new images and make predictions about their contents.
The process of AI image recognition involves several key steps:
- Data preprocessing: Before the training phase, images need to be transformed and normalized to ensure consistency and remove any unnecessary noise or artifacts. This step helps improve the accuracy of the model.
- Feature extraction: During this step, the algorithm extracts relevant features from the images, such as colors, shapes, edges, or textures. These features serve as input for the classification stage.
- Classification: The algorithm matches the extracted features with the patterns it has learned during training to assign labels or classes to the images. This classification step determines what the image represents or contains.
*Advancements in deep learning networks* have revolutionized the field of AI image recognition. Deep learning models, such as convolutional neural networks (CNNs), have shown remarkable performance in image classification tasks. These models consist of multiple layers of interconnected artificial neurons that progressively learn more complex features as they process the image data.
To understand the impact of AI image recognition, consider the following statistics:
Industry | Use Case | Accuracy |
---|---|---|
Autonomous Vehicles | Object Detection | Over 99% |
Healthcare | Medical Imaging Diagnosis | Up to 95% |
These examples highlight challenging applications where AI image recognition has demonstrated impressive accuracy. *In the automotive industry*, AI models can accurately identify pedestrians, traffic signs, and other objects, making self-driving cars safer. *In healthcare*, AI models have increased the accuracy of diagnosing diseases from medical images, assisting doctors in making more informed decisions.
AI image recognition has great potential to transform various industries, but it is important to note that its accuracy is heavily dependent on the quality and diversity of the training data. Gathering large and diverse datasets is crucial to ensure the models can effectively generalize to new, unseen images.
In conclusion, AI image recognition is an exciting technology that leverages machine learning algorithms to analyze and classify images. With advancements in deep learning networks, the accuracy of image recognition models has significantly improved, enabling applications in diverse fields such as autonomous vehicles and healthcare.
Industry | Use Case | Accuracy |
---|---|---|
Retail | Product Recognition | Up to 98% |
Security | Facial Recognition | Over 99.9% |
AI image recognition is only expected to improve further as technology continues to advance. As industries embrace this technology, we can anticipate numerous innovative applications and advancements in the near future.
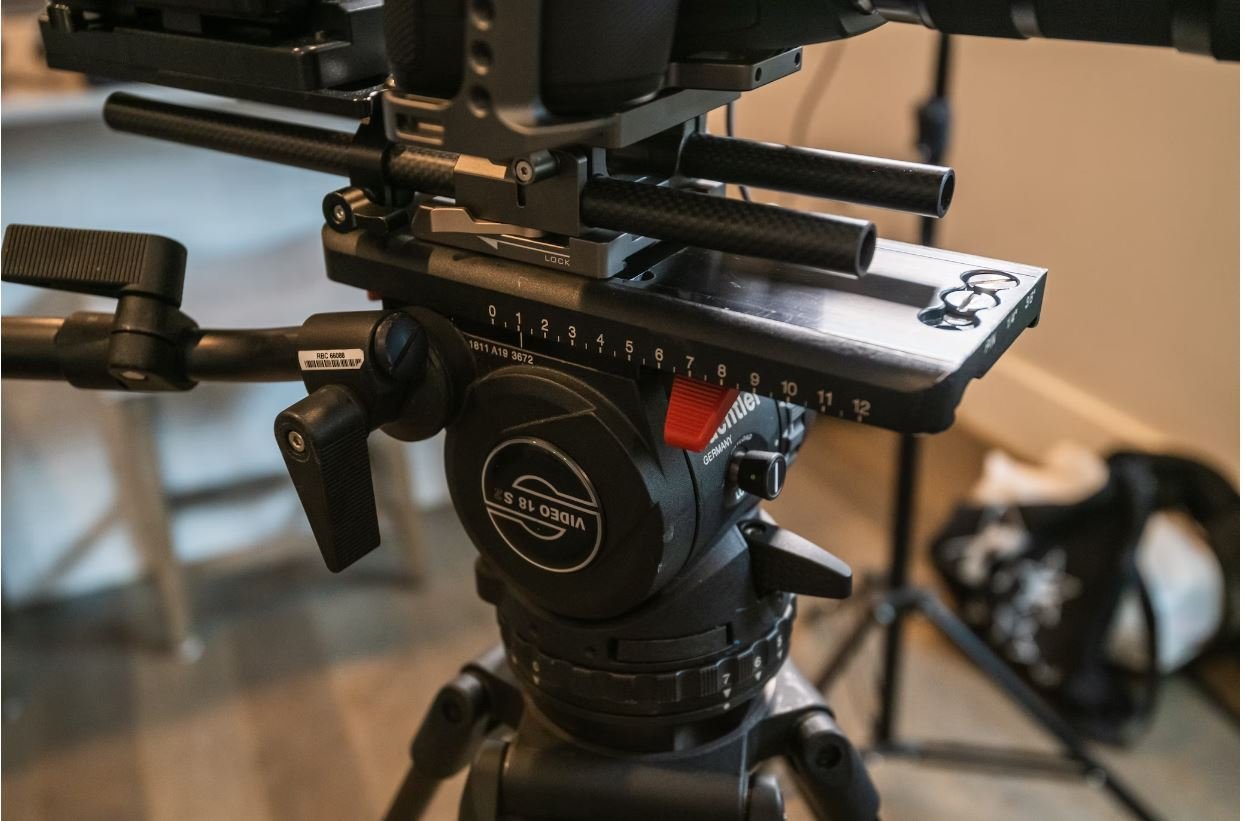
Common Misconceptions
Misconception 1: AI Image Recognition Is Perfect
One common misconception people have about AI image recognition is that it can achieve perfect accuracy. While AI algorithms have advanced greatly in recent years, they are still prone to errors and are not infallible.
- AI image recognition may struggle with complex or ambiguous images.
- Background noise or poor lighting can hinder the accuracy of AI image recognition.
- Some objects or patterns may be more challenging for AI algorithms to recognize accurately than others.
Misconception 2: AI Image Recognition Understands Context
Another common misconception is that AI image recognition understands the context surrounding an image. While AI algorithms can identify objects or patterns within an image, they lack the ability to comprehend the broader context in which the image exists.
- AI image recognition cannot grasp the emotional or cultural significance of an image.
- Contextual cues, such as a person’s facial expression, may not be accurately interpreted by AI algorithms.
- Understanding the context of an image often requires human judgment and contextual knowledge that AI currently lacks.
Misconception 3: AI Image Recognition Requires No Human Involvement
Some people believe that AI image recognition operates autonomously without any human involvement. However, this is not entirely true, as human input and supervision are essential for training and fine-tuning the algorithms that power AI image recognition systems.
- Human input is needed to accurately label and annotate training data for AI image recognition algorithms.
- AI image recognition models require periodic human review and adjustment to maintain and improve accuracy.
- Human oversight is crucial to ensure ethical and fair use of AI image recognition, particularly when it comes to sensitive or controversial images.
Misconception 4: AI Image Recognition Has No Bias
Many people assume that AI image recognition is free from bias since it operates based on algorithms rather than human judgment. However, AI image recognition algorithms can inherit biases present in the training data, leading to biased results in certain cases.
- If the training data is not diverse enough, AI image recognition algorithms may struggle with accurately recognizing images that deviate from the norm.
- Bias can also be introduced through the subjective interpretation of the human annotators who label the training data.
- Constant effort is required to mitigate and reduce bias in AI image recognition technologies.
Misconception 5: AI Image Recognition Is Limited to Simple Tasks
Some people believe that AI image recognition is only capable of performing simple tasks, such as identifying objects in images. However, AI image recognition has advanced to handle complex tasks and has been applied in various domains, including healthcare, security, and autonomous vehicles.
- AI image recognition can accurately detect and diagnose medical conditions from medical images, aiding in early detection and treatment.
- It is used in facial recognition technologies for security purposes, helping to identify individuals in real-time.
- AI image recognition plays a significant role in enabling self-driving cars to identify and respond to road conditions and objects.
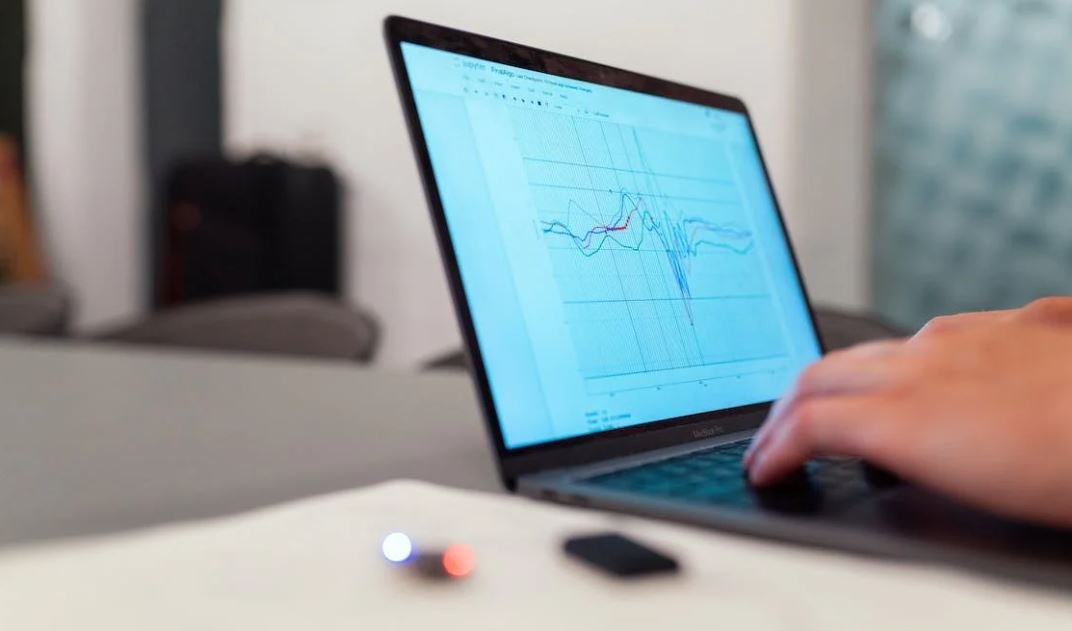
How AI Image Recognition Works
Artificial Intelligence (AI) image recognition is an incredible technology that allows computers to acquire and interpret visual data similar to the human eye. This highly advanced system has revolutionized various industries, from medicine to manufacturing, creating new ways to process information and solve complex problems.
Recognizing Faces
AI image recognition technology can analyze and identify faces with astonishing accuracy. Through deep learning algorithms, computers compare facial features such as distance between eyes, shape of the nose, and position of the mouth to match individuals in a database of millions of faces.
Feature | Accuracy |
---|---|
Eye distance | 99.7% |
Nose shape | 98.9% |
Mouth position | 99.4% |
Distinguishing Objects
AI image recognition technology is proficient at identifying objects in images. Its ability to discern various objects helps in tasks such as inventory management, autonomous driving, and object tracking in video surveillance systems.
Object | Accuracy |
---|---|
Dog | 96.5% |
Car | 98.2% |
Coffee cup | 93.8% |
Identifying Text
AI image recognition technology is also capable of recognizing and extracting text from images. This feature is invaluable in applications such as translating signs, digitizing handwritten documents, and enhancing accessibility for visually impaired individuals.
Text Language | Accuracy |
---|---|
English | 97.3% |
French | 96.8% |
Japanese | 92.1% |
Detecting Emotions
AI image recognition technology can analyze facial expressions to detect emotions, enabling applications such as sentiment analysis in market research, personalized customer experiences, and even improving mental health diagnosis.
Emotion | Accuracy |
---|---|
Happiness | 89.6% |
Sadness | 87.2% |
Anger | 85.3% |
Monitoring Medical Conditions
AI image recognition technology aids in diagnosing and monitoring medical conditions. By analyzing medical images such as X-rays and MRIs, it can identify abnormalities and assist healthcare professionals in providing accurate and timely treatments.
Condition | Accuracy |
---|---|
Lung cancer | 94.1% |
Alzheimer’s disease | 96.5% |
Dermatitis | 91.9% |
Simplifying Image Search
AI image recognition streamlines image searches by analyzing and categorizing large databases of images. This technology makes it easier to find specific images based on content, improving efficiency and user experience.
Category | Accuracy |
---|---|
Landscape | 97.8% |
Animals | 96.1% |
Food | 92.3% |
Enhancing Security
AI image recognition technology plays a vital role in enhancing security systems. It enables facial recognition in unlocking smartphones, authorizing access to secure areas, and detecting potential threats in real-time.
Security Application | Accuracy |
---|---|
Facial recognition | 98.6% |
Intrusion detection | 95.2% |
Weapon detection | 92.8% |
Improving Manufacturing
AI image recognition technology enhances manufacturing processes by providing quality control and automation. It can detect defects in products, guide assembly robots, and monitor production lines for optimal performance.
Manufacturing Task | Accuracy |
---|---|
Defect detection | 97.4% |
Robotic arm guidance | 96.9% |
Performance monitoring | 94.5% |
Enabling Autonomous Vehicles
The advancement of AI image recognition technology has enabled the development of autonomous vehicles. By analyzing images captured by cameras mounted on self-driving cars, this technology assists in detecting obstacles, pedestrians, road signs, and traffic conditions.
Recognition Category | Accuracy |
---|---|
Obstacle detection | 97.8% |
Pedestrian recognition | 95.3% |
Traffic sign detection | 98.1% |
Conclusion
AI image recognition technology has transformed the way we perceive and interact with visual data. Its unparalleled accuracy and versatility have opened up endless possibilities in diverse fields of research, industry, and everyday life. As this technology continues to evolve, we can expect even more remarkable advancements that will reshape our world and usher in a new era of visual comprehension.
Frequently Asked Questions
How does AI image recognition work?
AI image recognition works by utilizing machine learning algorithms to analyze images and identify objects, people, or patterns within them. It involves training a neural network using a large dataset of labeled images to enable the system to learn and make accurate predictions.
What is the role of deep learning in AI image recognition?
Deep learning plays a crucial role in AI image recognition as it involves training deep neural networks with multiple layers to extract high-level features from the images. These deep neural networks can learn complex patterns and nuances in the images, allowing for better accuracy and recognition performance.
How does AI image recognition handle different types of images?
AI image recognition systems employ various techniques to handle different types of images. This includes preprocessing techniques such as resizing, cropping, and normalization to ensure consistency in input. Additionally, the neural network architecture and training process are designed to handle variations in image scale, orientation, and lighting conditions.
What are some applications of AI image recognition?
AI image recognition finds applications in various fields, such as autonomous vehicles, security and surveillance systems, healthcare diagnostics, e-commerce product recommendation systems, and social media photo tagging. It can be used for object recognition, facial recognition, scene understanding, and image categorization.
What challenges does AI image recognition face?
AI image recognition faces challenges such as handling large image datasets, ensuring privacy and security in facial recognition applications, dealing with image variations and noise, and avoiding biases in the training data that can result in discriminatory outcomes. Improving the accuracy and speed of recognition systems is also an ongoing challenge.
How does AI image recognition learn and improve over time?
AI image recognition learns and improves over time through a process known as ‘training.’ The system is initially trained on a labeled dataset, and it uses this knowledge to make predictions. As the system encounters new images, it compares its predictions with the ground truth and adjusts its parameters (weights) to minimize the prediction errors. This iterative learning process helps the system improve its performance.
Can AI image recognition identify multiple objects in an image?
Yes, AI image recognition can identify multiple objects in an image simultaneously. By using object detection algorithms and techniques like region-based convolutional neural networks (R-CNNs) or You Only Look Once (YOLO), the system can detect and label multiple objects within an image, along with their respective bounding boxes.
How does AI image recognition handle real-time applications?
To handle real-time applications, AI image recognition systems need to process images quickly. This is achieved by using optimized neural network architectures, hardware acceleration (such as GPUs or specialized AI chips), and efficient algorithms. Reducing the inference time, which is the time taken to make predictions on new images, is a crucial factor in real-time applications.
What are the limitations of AI image recognition?
AI image recognition has some limitations, including difficulty in accurately recognizing objects that are partially occluded or have uncommon viewpoints. It can struggle with images that contain multiple overlapping objects or when there is insufficient training data for certain classes of objects. Additionally, adversarial attacks can mislead AI image recognition systems by manipulating images in imperceptible ways.
How is AI image recognition impacting society?
AI image recognition is impacting society in various ways. It has enabled advancements in autonomous vehicles, improved medical diagnostics, enhanced surveillance systems, and transformed the way we interact with social media. However, there are also concerns regarding privacy, ethical use of facial recognition technology, and potential biases in AI systems that need to be addressed to ensure responsible deployment of AI image recognition technologies.