Generative Models for Image Processing
Image processing has made significant advancements in recent years, with generative models playing a crucial role in this progress. Generative models are algorithms that can generate new data samples similar to a given training dataset. In the field of image processing, generative models have enabled numerous applications, such as image super-resolution, image inpainting, and style transfer. This article dives into the world of generative models for image processing, exploring their benefits and applications.
Key Takeaways:
- Generative models are algorithms used in image processing to generate new data samples similar to a given training dataset.
- They enable various applications such as image super-resolution, image inpainting, and style transfer.
- Generative models have revolutionized the field of image processing and have opened up new possibilities for creative manipulation of images.
Understanding Generative Models
Generative models, such as autoencoders and generative adversarial networks (GANs), have gained popularity for their ability to learn complex patterns and generate realistic images. These models use statistical techniques to capture the underlying distribution of the training images and then generate new samples based on this learned distribution.
One interesting sentence: Generative models can generate a range of output images, allowing users to explore different possibilities and variations.
Applications of Generative Models in Image Processing
Generative models have found applications in various areas of image processing:
1. Image Super-Resolution
Generative models can enhance the resolution of low-resolution images, producing visually sharper and more detailed outputs. This is particularly useful in the field of medical imaging, where high-resolution images are crucial for accurate diagnosis and analysis.
2. Image Inpainting
Generative models are used to fill in missing or corrupted parts of an image based on the surrounding context. This technique is valuable for restoring damaged images or removing unwanted objects from photographs.
3. Style Transfer
Generative models enable the transfer of artistic styles from one image to another. By learning the style of a reference image, a generative model can apply similar artistic effects to other images, creating visually compelling results.
One interesting sentence: Generative models have the potential to revolutionize the creative industry by automating the process of generating unique and visually impressive images.
Advancements and Challenges
Generative models have undergone significant advancements, leading to impressive results in image processing. However, several challenges persist:
- The quality of generated images is highly dependent on the size and quality of the training dataset.
- Training generative models can be computationally intensive and time-consuming.
- Ensuring that generated images are diverse and cover the full range of variations present in the training dataset remains a challenge.
Data Augmentation Techniques in Image Processing
Data augmentation techniques are used to enhance the performance and robustness of generative models for image processing:
- Rotation: Rotating the input images at different angles to increase the diversity of training samples.
- Translation: Shifting the images horizontally or vertically to introduce positional variation.
- Scaling: Resizing the images to different scales, simulating different perspectives.
Data Augmentation Techniques for Image Processing
Technique | Description |
---|---|
Rotation | Rotating the input images at different angles to increase the diversity of training samples. |
Translation | Shifting the images horizontally or vertically to introduce positional variation. |
Scaling | Resizing the images to different scales, simulating different perspectives. |
Conclusion
Generative models have revolutionized image processing by enabling applications such as image super-resolution, image inpainting, and style transfer. These models have the potential to transform various industries, including healthcare, digital art, and entertainment. With ongoing advancements and research in generative models, the possibilities for creative image manipulation are endless.
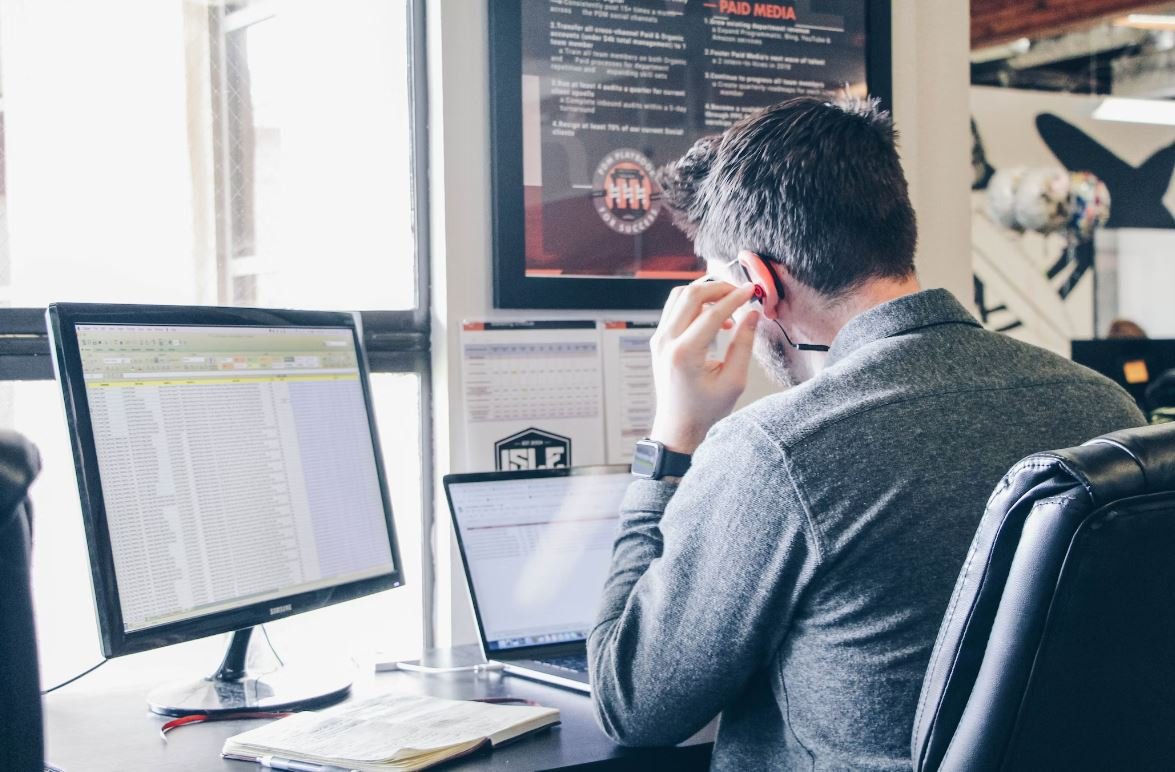
Generative Models for Image Processing
Common Misconceptions
Misconception 1: Generative models can perfectly replicate any image:
- Generative models can create realistic images, but not with 100% accuracy.
- Imperfections in the generated images are inevitable due to the complexity of real-world images.
- The level of detail and accuracy achieved by generative models depends on various factors like training data, model architecture, and hyperparameters.
Misconception 2: Generative models can only create new images:
- While generative models can generate new images, they can also modify existing images.
- Generative models can be used for image translation, style transfer, and other image manipulation tasks.
- These models have the ability to learn the underlying patterns and structures in images and apply them to generate new or modified images.
Misconception 3: Generative models are only applicable to artistic or creative domains:
- Generative models have applications beyond artistic and creative domains.
- They can be used in various fields like medical imaging, computer vision, data augmentation, and even in generating realistic synthetic data for training machine learning models.
- Generative models provide novel solutions in fields where generating or manipulating high-quality images is crucial.
Misconception 4: Generative models always generate accurate images that are indistinguishable from real ones:
- While generative models have made significant progress in generating realistic images, they are not foolproof in replicating real images without any observable differences.
- Subtle artifacts or imperfections can still be present even in high-quality generated images.
- Evaluating the realism and quality of generated images requires careful analysis and comparison to real images.
Misconception 5: Generative models will replace human artists or photographers:
- Generative models are tools that can assist artists and photographers in their creative process.
- They can potentially automate certain aspects of image creation or provide inspiration for artists.
- However, the subjective and unique aspects of human creativity and expression cannot be replicated solely by generative models.
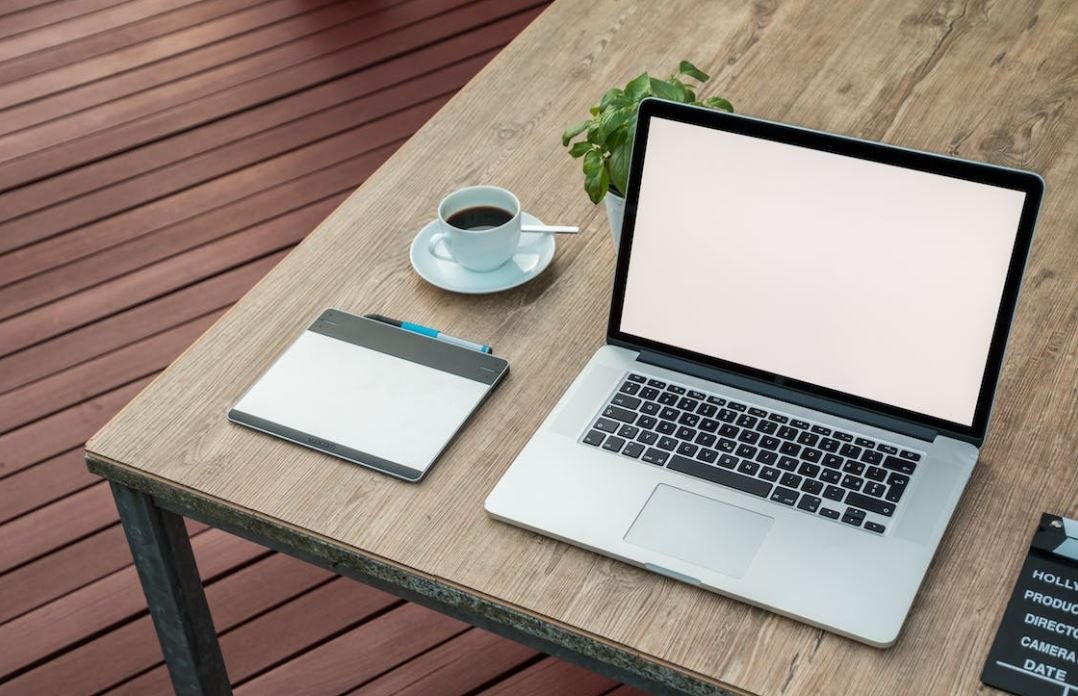
Introduction
In recent years, generative models have revolutionized the field of image processing. These models, fueled by advancements in deep learning and artificial intelligence, have made significant strides in generating high-quality images, enhancing existing ones, and even creating entirely new visual content. This article explores ten fascinating aspects and advancements within generative models for image processing.
Table 1: Applications of Generative Models in Image Processing
Generative models have found numerous applications in image processing, ranging from image super-resolution and style transfer to image inpainting and synthesis.
Table 2: Comparison of Generative Adversarial Networks (GANs) and Variational Autoencoders (VAEs)
Two popular types of generative models are GANs and VAEs. Each has its own strengths and weaknesses, making them the focus of extensive research and comparison within the image processing community.
Table 3: Image Quality Assessment Metrics for Generative Models
For evaluating the performance of generative models, various metrics have been developed to objectively measure the quality, diversity, and realism of generated images.
Table 4: Conditional Generation with Text Inputs
Generative models can be conditioned on text inputs, enabling the generation of images based on specific textual descriptions.
Table 5: Cross-Domain Image Translation with CycleGAN
CycleGAN is a generative model designed to facilitate the translation of images between different domains, such as transforming summer landscapes into winter scenes or horses into zebras.
Table 6: Generative Models for Image Inpainting
Image inpainting techniques utilizing generative models effectively fill in missing or corrupted parts of images, making them visually coherent and consistent with the surrounding context.
Table 7: Image Synthesis with Style Transfer Techniques
Style transfer methods based on generative models allow artists and creators to transfer the style of one image onto the content of another, resulting in unique and visually striking compositions.
Table 8: Generative Models for Image Super-Resolution
Generative models have made significant contributions to the field of image super-resolution, enabling the enhancement of low-resolution images to higher resolutions while preserving important details and textures.
Table 9: Deep Dream: Enhancing Images with Deep Learning
Deep Dream is a generative model-based technique that enhances images by amplifying and visualizing patterns detected by a pre-trained deep neural network, resulting in dream-like and trippy visual effects.
Table 10: Generative Models in Digital Art and Entertainment
Generative models have found a place in the world of digital art and entertainment, where they are utilized for creating unique visual experiences, generating realistic characters and environments, and even designing virtual worlds.
Conclusion
Generative models have revolutionized the field of image processing by providing innovative solutions to various challenges. From enhancing image quality and performing cross-domain translations to creating art and generating realistic images, these models continue to advance the boundaries of what is possible in the realm of digital visual content. With ongoing research and development, the future of generative models holds even greater potential for transforming the way we perceive and interact with images.
Frequently Asked Questions
What are generative models?
Generative models are a type of machine learning models that can learn the underlying distribution of a given dataset and generate new, synthetic data that follows a similar distribution.
Why are generative models important for image processing?
Generative models are important for image processing as they can be used to generate high-quality images, inpaint missing parts of an image, enhance low-resolution images, and create realistic visual data for various applications.
What is image inpainting?
Image inpainting is the process of filling in missing or corrupted parts of an image with plausible content. Generative models can be trained to inpaint missing parts and produce visually coherent results.
What are some popular generative models for image processing?
Some popular generative models for image processing include Variational Autoencoders (VAEs), Generative Adversarial Networks (GANs), and Autoregressive Models (e.g., PixelCNN).
How do Variational Autoencoders (VAEs) work?
VAEs consist of two main components, an encoder and a decoder. The encoder maps the input image to a lower-dimensional latent space, while the decoder maps the latent space back to the image space. VAEs are trained to minimize the reconstruction error and encourage the latent space to follow a desired distribution.
What is the concept behind Generative Adversarial Networks (GANs)?
GANs consist of two neural networks, a generator and a discriminator, which compete against each other. The generator generates synthetic images and tries to fool the discriminator, while the discriminator learns to distinguish real from fake images. Through this adversarial training, GANs can generate highly realistic images.
What is the difference between GANs and VAEs?
While both GANs and VAEs are generative models, they have different underlying principles. GANs aim to learn the true data distribution and generate realistic images, while VAEs focus on maximizing the likelihood of the observed data and generating new samples from a learned distribution. GANs often produce more visually appealing results, but VAEs provide better control over the generated samples.
How can generative models be used in medical image processing?
Generative models can assist in medical image processing tasks like denoising, super-resolution, and segmentation. They can learn from large datasets of medical images and generate synthetic data that helps improve the accuracy and efficiency of medical image analysis.
What are some challenges in training generative models for image processing?
Training generative models can be challenging due to issues like mode collapse (where the model generates limited diversity), unstable training dynamics, and the need for large amounts of training data. Overcoming these challenges often requires careful model selection, optimization techniques, and quality evaluation.
How can I get started with generative models for image processing?
To get started with generative models for image processing, you can begin by learning the basics of machine learning and deep learning. Familiarize yourself with popular generative models like VAEs and GANs and explore available libraries and frameworks (such as TensorFlow or PyTorch) that offer comprehensive resources and tutorials to experiment with generative models.