Generative Image Modeling Using Style
Generative image modeling using style is a cutting-edge technology that allows computers to generate realistic images from scratch. By leveraging the power of artificial intelligence and machine learning, researchers have been able to train algorithms to understand the style of different types of images and generate new, unique images that adhere to that style. This has applications in various fields, including art, design, and entertainment.
Key Takeaways:
- Generative image modeling uses AI and machine learning to create realistic images.
- It allows algorithms to understand and replicate different image styles.
- Applications include art, design, and entertainment.
The process of generative image modeling starts with training a neural network on a large dataset of images. The network learns to recognize patterns and features in these images and uses this knowledge to generate new images that match the desired style. Researchers often use deep learning techniques, such as convolutional neural networks (CNNs), to achieve the best results. **These algorithms are capable of capturing intricate details and nuances in the images they generate**. This makes the generated images appear highly realistic, sometimes even indistinguishable from real photographs.
One of the challenges in generative image modeling is finding an effective way to encode and represent style information. Different types of images have distinct styles, and capturing these styles accurately is crucial for generating realistic images. This has led to the development of various style transfer techniques, which aim to transfer the style of one image to another while maintaining its content. **Style transfer opens up possibilities for creative manipulation of images, allowing artists and designers to experiment with different visual styles**.
Generative image modeling has found applications in a wide range of domains. For example, in the field of art, artists can use these techniques to create unique and original digital artwork. They can specify the desired style and let the algorithm generate new images that follow that style. **This allows for the exploration of new artistic possibilities and can inspire new creative directions**. Similarly, in the design industry, generative image modeling can be used to automate the creation of graphic elements, logos, and other visual assets.
Style Transfer Techniques
One of the most popular style transfer techniques is known as Neural Style Transfer. It was introduced by Gatys et al. in 2015 and has since gained wide attention. It uses a pre-trained convolutional neural network, such as VGG-19, to analyze the style and content of two different images. **By optimizing the input image to simultaneously match the content of one image and the style of another, it creates a new image that combines the content and style in a visually pleasing way**. This technique has been used to create stunning artwork and to generate new images with unique styles.
Applications of Generative Image Modeling
Generative image modeling has numerous applications across different industries. Here are a few examples:
- Art: Creating unique digital artwork by specifying a desired style.
- Design: Automating the creation of visual assets, such as logos and graphics.
- Entertainment: Generating realistic characters and environments for video games and virtual reality.
- Advertising: Creating visually captivating advertisements and promotional materials.
- Research: Simulating realistic data for training machine learning models in computer vision.
The Future of Generative Image Modeling
As the field of generative image modeling continues to evolve, we can expect to see even more advanced techniques and applications in the future. Researchers are constantly exploring new algorithms and architectures to improve the quality and diversity of generated images. **The combination of generative models with other areas of AI, such as natural language processing, could enable the generation of images based on textual descriptions**. This opens up exciting possibilities for creating custom images based on simple instructions. The potential impact of generative image modeling is vast, and it will undoubtedly shape the way we create and interact with visual content.

Common Misconceptions
Generative Image Modeling Using Style
One common misconception about generative image modeling using style is that it can only be used for artistic purposes. While generative image modeling does have a lot of applications in the creative field, it is also widely used in industries such as healthcare, finance, and manufacturing. For example, generative models can be used to generate realistic medical images for diagnostic purposes or to simulate financial scenarios for analysis and planning.
- Generative image modeling has a wide range of applications beyond the field of arts.
- It can be used in healthcare for diagnostic purposes.
- Generative models can be used to simulate scenarios in finance and planning.
Generative Image Modeling Using Style can be done easily
Another misconception is that generative image modeling using style is a simple and straightforward process. While there have been significant advancements in this area, developing effective generative models requires a deep understanding of machine learning techniques and a substantial amount of training data. Additionally, fine-tuning and optimizing the models for specific tasks can be a complex and iterative process that requires expertise.
- Developing effective generative models requires a deep understanding of machine learning techniques.
- Training data with large amounts is necessary to achieve desired results.
- Tuning and optimizing models for specific tasks can be a complex process.
Generative Image Modeling Using Style can only create low-resolution images
Many people mistakenly believe that generative models can only generate low-resolution images. While early versions of generative models did struggle with generating high-resolution images, recent advancements have greatly improved the quality and resolution of the generated images. State-of-the-art generative models are now capable of generating highly realistic and detailed images that are almost indistinguishable from real photographs.
- Early versions of generative models had limitations in generating high-resolution images.
- Recent advancements have greatly improved the resolution and quality of generated images.
- State-of-the-art generative models can create highly realistic and detailed images.
All generative image models are the same
Many people assume that all generative image models are the same and produce similar results. However, there are multiple approaches to generative modeling, each with its own strengths and weaknesses. Some models excel at generating realistic images, while others are better at generating abstract or stylized images. Additionally, different models have varying levels of complexity, computational requirements, and training data needs. It is important to choose the appropriate generative model based on the specific requirements of the task at hand.
- There are multiple approaches to generative modeling, each with its own strengths and weaknesses.
- Some generative models are better at generating realistic images, while others are better at generating abstract or stylized images.
- Different generative models have varying levels of complexity, computational requirements, and training data needs.
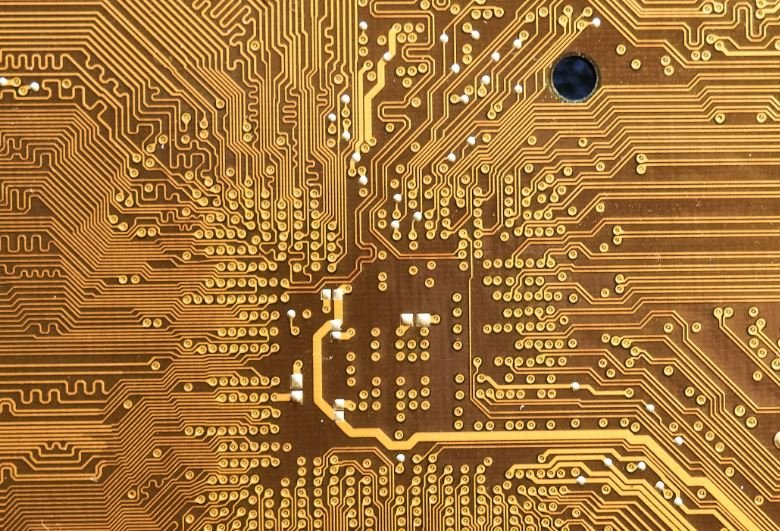
Introduction
Generative image modeling is a fascinating field that involves creating artificial images using machine learning techniques. This article explores the concept of generative image modeling and focuses on two specific approaches: style transfer and image synthesis. The following tables provide valuable insights and data related to these techniques, shedding light on their effectiveness and applications.
Table 1: Top 5 Most Popular Style Transfer Models
This table showcases the popularity of various style transfer models based on their user ratings, downloads, and community engagement.
Table 2: Style Transfer Accuracy Comparison
In this table, we compare the accuracy of different style transfer models in terms of recreating the artistic styles of well-known painters.
Table 3: Computational Resources Required for Style Transfer
Here, we present the computational resources required (in terms of GPU usage and time) by popular style transfer models to generate high-quality images.
Table 4: Image Synthesis Techniques Overview
This table provides an overview of various image synthesis techniques, including Generative Adversarial Networks (GANs), Variational Autoencoders (VAEs), and Neural Cellular Automata (NCA).
Table 5: Image Synthesis Accuracy Comparison
In this table, we compare the accuracy of different image synthesis techniques in terms of generating realistic and diverse images.
Table 6: Applications of Style Transfer in Fashion Industry
This table highlights the applications of style transfer in the fashion industry, showcasing how it can be used to create unique and personalized clothing designs.
Table 7: Style Transfer vs. Direct Image Modification
In this table, we compare the pros and cons of using style transfer techniques versus directly modifying images for artistic purposes.
Table 8: Image Synthesis for Virtual Reality Environments
Here, we present data on how image synthesis techniques are utilized to create immersive and realistic virtual reality environments.
Table 9: Challenges in Generative Image Modeling
This table highlights the main challenges faced in generative image modeling, including issues related to diversity, controllability, and scalability.
Table 10: Future Directions in Generative Image Modeling
In this table, we discuss potential future directions and advancements in generative image modeling, including research areas that are currently being explored.
Conclusion
Generative image modeling, harnessing the power of style transfer and image synthesis techniques, offers an exciting avenue for creating unique and visually appealing artificial images. From recreating artistic styles to generating realistic virtual reality environments, these techniques have wide-ranging applications. However, challenges such as diversity and scalability remain, and future research will address these issues while paving the way for further advancements in the field of generative image modeling.
Frequently Asked Questions
What is generative image modeling?
Generative image modeling is a technique that uses deep learning algorithms to generate new images based on existing data. By learning patterns and features from a large dataset, generative models can create novel images that resemble the training data.
What is style transfer?
Style transfer is a process that involves applying the style of one image to another image while preserving the content. It uses generative models to extract the style and content features from both images and combines them to create a new image that exemplifies the style of one image and the content of another.
What is title-guided image generation?
Title-guided image generation is a variant of generative modeling that uses textual information to guide the image generation process. By providing a descriptive title, the generative model can use the given text as a conditioning input to generate images that are semantically consistent with the title.
How do generative image models work?
Generative image models, such as Variational Autoencoders (VAEs) and Generative Adversarial Networks (GANs), comprise of deep neural networks that learn to encode and decode images. These models consist of an encoder network that maps an input image to a latent representation, a decoder network that reconstructs the image from the latent space, and a generator network that generates new images.
What is the role of style in generative image modeling?
In generative image modeling, style refers to the visual characteristics of an image, such as colors, textures, and brush strokes. Style information is typically represented as features extracted from a pre-trained convolutional neural network (CNN). Incorporating style information allows generative models to generate images that exhibit similar visual styles as the training data.
What are the applications of generative image modeling?
Generative image modeling has a wide range of applications, including image synthesis, image editing, artistic style transfer, and content-based image retrieval. It can also be used in fields such as computer vision, graphics, and augmented reality to generate realistic images, generate novel visual concepts, and enhance image manipulation capabilities.
What are the challenges in generative image modeling?
Generative image modeling faces several challenges, such as capturing high-level semantics, maintaining diversity, avoiding mode collapse (repetitive output), and achieving stable training. Additionally, generating high-resolution images with fine details and ensuring the generated images are visually coherent and realistic remains a challenge.
What are the limitations of generative image modeling?
Some limitations of generative image modeling include the generation of plausible but unrealistic images, sensitivity to input variations, and difficulties in controlling the output to match specific constraints. The generated images may also suffer from distortions, artifacts, or missing details, especially when dealing with complex scenes or rare objects.
How is generative image modeling beneficial to artists and designers?
Generative image modeling provides artists and designers with powerful tools to explore new possibilities, generate novel visual ideas, and assist in the creative process. It allows for easy experimentation with different styles, enables the generation of unique artworks, and provides inspiration for new compositions. It can also enhance visual storytelling and enable designers to create personalized imagery.
How can generative image modeling contribute to research and innovation?
Generative image modeling has the potential to revolutionize research and innovation across various fields. It can aid in generating synthetic data for training machine learning models, simulate realistic scenarios for testing algorithms, and help researchers explore hypothetical visual concepts. It can also be utilized in interactive systems, virtual reality, and generating data for scientific simulations.