Generative Image AI Models
Introduction: Generative Image AI Models are powerful tools that use algorithms to produce new images based on existing data. These models have become increasingly popular in various fields, such as art, design, and entertainment, enabling users to create unique and realistic images that were previously unimaginable. This article explores the capabilities and applications of generative image AI models, shedding light on their potential impact on various industries.
Key Takeaways:
- Generative Image AI models use algorithms to create new images based on existing data.
- These models have numerous applications in art, design, and entertainment.
- The technology allows users to generate unique and realistic images.
Generative Image AI Models Explained: Generative Image AI Models are deep learning algorithms that utilize complex neural networks to generate new images. They leverage large datasets to learn patterns and features from existing images, enabling them to create original and high-quality visuals. By analyzing vast amounts of data, these models can understand and replicate unique styles, structures, and details, allowing users to produce images that possess the characteristics of specific artist styles or artistic eras.
Generative Image AI Models can generate new images based on existing data, mimicking the style and characteristics of artists or artistic eras.
Applications of Generative Image AI Models: The applications of generative image AI models are vast and diverse. Let’s explore a few notable use cases:
- Art Creation: Artists can use generative image AI models to spark creativity and generate unique ideas. These models assist in generating new artwork inspired by their preferred styles or incorporating elements from various artistic movements.
- Design and Fashion: Designers and fashion industry professionals can leverage generative image AI models to create new patterns, prints, and textures for their designs. This allows for more innovative and customizable creations.
- Entertainment and Gaming: Generative image AI models have vast applications in the entertainment and gaming industry. They can be used to generate realistic characters, landscapes, and special effects, enhancing the overall visual experience for users.
Generative Image AI Models have a wide range of applications in various industries, including art, design, fashion, entertainment, and gaming.
The Impact of Generative Image AI Models:
Generative image AI models have revolutionized the creative process and opened up new possibilities for artists, designers, and creators across industries. Their impact can be summarized as follows:
- Creative Enhancement: These AI models serve as valuable tools for enhancing creativity and enabling artists to explore new territories.
- Efficiency and Productivity: Generative image AI models can significantly speed up the design process, unlock new design options, and increase overall productivity.
- Customizability: With these models, users are empowered to create highly customizable and unique designs tailored to their specific preferences and needs.
Industry | Applications |
---|---|
Art | Generate artwork inspired by different styles and movements. |
Design | Create innovative patterns, prints, and textures for fashion and product design. |
Entertainment | Generate realistic characters, landscapes, and special effects. |
Generative image AI models have significantly impacted the creative process, providing artists with new tools for enhancing creativity, productivity, and customizability.
Conclusion:
Generative Image AI models have revolutionized industries such as art, design, and entertainment by enabling users to generate unique and realistic images based on existing data. Their wide range of applications and impact on creativity, productivity, and customizability make them invaluable tools for artists, designers, and creators. As the technology continues to evolve, we can expect even more remarkable developments in image generation and visual arts.
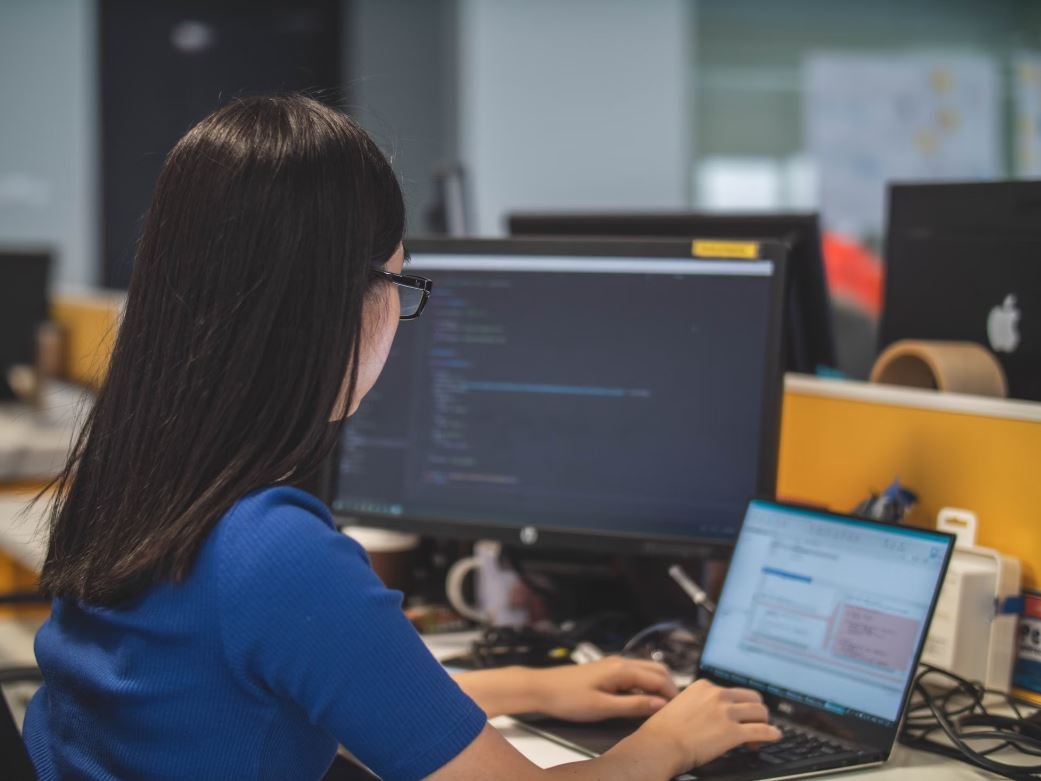
Common Misconceptions
Misconception 1: Generative Image AI models can perfectly replicate any image
One common misconception about generative image AI models is that they have the ability to perfectly replicate any given image. However, this is not true as these models work by creating new images based on existing data and patterns they have learned. They are not capable of reproducing every detail of an image with 100% accuracy.
- Generative image AI models rely on patterns and data they have been trained on.
- They may struggle with reproducing complex textures and fine details.
- Their output will always have some degree of variation and interpretation.
Misconception 2: Generative image AI models always produce high-quality images
Another misconception is that generative image AI models always produce high-quality images. While these models have made significant advancements in generating realistic images, there are still limitations to the quality of the output. Factors such as the complexity of the image, the training data, and the parameters set for the model can all affect the quality of the generated images.
- The quality of the training data impacts the output image quality.
- Complex images may result in lower-quality generated images.
- Parameters set for the model can be adjusted to enhance the image quality.
Misconception 3: Generative image AI models always produce original and unique images
Many people assume that generative image AI models always produce original and unique images. While these models are indeed capable of creating new images, they often rely on existing data and patterns in the training set. As a result, there is a degree of similarity and repetition in the generated images.
- Generative image AI models often combine and remix existing patterns to create new images.
- There is a likelihood of repetition and similarities in the generated images.
- The uniqueness of the generated images depends on the diversity of the training data.
Misconception 4: Generative image AI models always understand and interpret the content of an image accurately
One misconception is that generative image AI models can understand and interpret the content of an image accurately. While these models can learn patterns and generate images, they do not possess true understanding or semantic comprehension of the images they generate. They operate based on statistical patterns rather than semantic meaning.
- Generative image AI models do not possess true semantic understanding of images.
- Their output is based on learned patterns rather than true comprehension.
- They are incapable of interpreting the complex meaning behind an image.
Misconception 5: Generative image AI models only generate realistic images
It is a common misconception that generative image AI models can only generate realistic images. While realism is often the goal, these models can also produce abstract or surrealistic images depending on the training data and parameters set. This versatility allows for a range of creative possibilities and aesthetic variations.
- Generative image AI models can generate abstract or surreal images as well.
- Their output depends on the given training data and set parameters.
- They can be used to explore creative and artistic possibilities beyond realism.
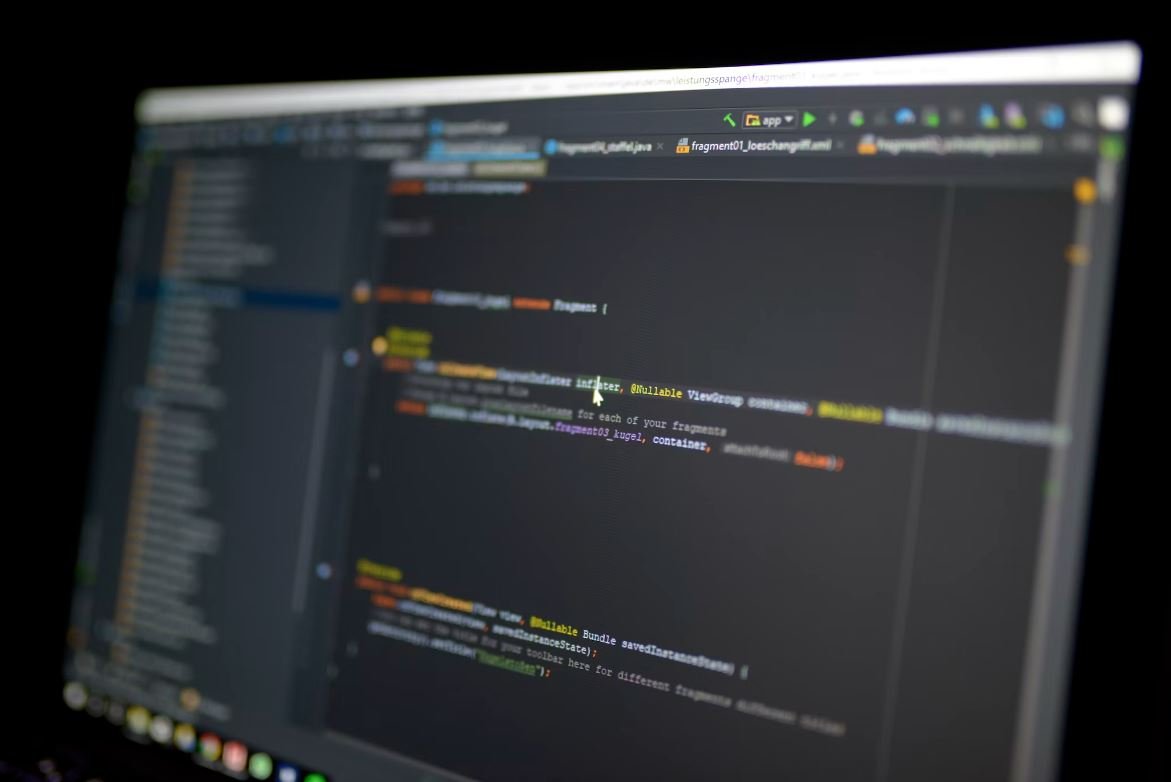
Table: Popular Generative Image AI Models
Below is a list of some popular generative image AI models used in various applications:
Model Name | Description | Applications |
---|---|---|
DeepArt | A neural network that applies artistic styles to images. | Artistic rendering, style transfer |
DeepDream | Produces surreal and trippy images by enhancing patterns. | Creative art, visualizing neural networks |
StackGAN | Generates high-resolution images from textual descriptions. | Fine art synthesis, text-to-image synthesis |
DCGAN | Creates realistic images using unsupervised learning. | Data augmentation, image generation |
Pix2Pix | Translates images from one domain to another. | Image-to-image translation, image colorization |
Table: Comparison of Image Quality Metrics
Various metrics can be used to assess the quality of generative images. The following table compares some commonly used metrics:
Metric | Description | Range | Advantages |
---|---|---|---|
SSIM | Structural similarity index | 0-1 (higher is better) | Considers perception; works well with human eyes |
PSNR | Peak signal-to-noise ratio | 0-infinity (higher is better) | Easy to compute; widely used in image compression |
FID | Fréchet inception distance | 0-infinity (lower is better) | Compares generated images to real images |
Inception Score | Measures the quality and diversity of generated images | 0-infinity (higher is better) | Considers both image quality and diversity |
Table: Pretrained Generative Models
In the field of generative image AI, pretrained models offer a convenient starting point. Here are some widely used pretrained models:
Model | Architecture | Dataset | Applications |
---|---|---|---|
StyleGAN | Generative Adversarial Network (GAN) | CelebA-HQ | Portrait generation, character design |
BigGAN | Conditional GAN (cGAN) | ImageNet | Large-scale image synthesis, fine-grained control |
CycleGAN | Unpaired image-to-image translation GAN | – | Style transfer, domain adaptation |
Table: Generative Models in Industry
Generative AI models find practical applications across different industries:
Industry | Use Cases | Generative Models Used |
---|---|---|
Advertising | Personalized image ads, dynamic content generation | BigGAN, StackGAN |
Fashion | Virtual try-on, product design | CycleGAN, StyleGAN |
Entertainment | Movie special effects, CGI, virtual worlds | DeepArt, DeepDream |
Table: Key Challenges in Generative AI
Developing generative image AI models comes with its own set of challenges:
Challenge | Description |
---|---|
Training Complexity | Models may require significant computational resources and time. |
Evaluation Metrics | Deciding on appropriate metrics for image quality and diversity assessment is non-trivial. |
Mode Collapse | Generated images can lack diversity, appearing similar or limited in variation. |
Table: Generative Models by Year
Generative image AI has evolved over time, with new models emerging each year:
Year | Models |
---|---|
2014 | DCGAN |
2015 | Pixel RNN, Image-to-Image Translation Networks |
2016 | Neural Style Transfer, Progressive GAN |
2017 | CycleGAN, InfoGAN |
2018 | BigGAN, StackGAN |
Table: Generative Models and Art
The intersection of generative image AI and art has created exciting possibilities:
Art Movement | Description | Artists/Artworks |
---|---|---|
Algorithmic Art | Artistic creations generated using algorithms and AI. | Robbie Barrat’s AI-generated artworks |
AI-Driven Installation Art | Art installations that incorporate generative AI technologies. | Refik Anadol’s “Archive Dreaming” |
Interactive AI Art | Artworks that engage viewers through AI-based interactivity. | TeamLab’s “Borderless” digital art museum |
Table: Generative AI in Healthcare
Generative image AI has shown promising potential in the healthcare domain:
Application | Description | Generative Models Used |
---|---|---|
Medical Imaging | Generating synthetic medical images for training and testing algorithms. | DCGAN, CycleGAN |
Disease Diagnosis | AI systems that can diagnose diseases from medical images. | StackGAN, StyleGAN |
Radiation Therapy Planning | Generating patient-specific simulated images for treatment planning. | Pix2Pix, BigGAN |
In conclusion, generative image AI models have proven to be incredibly powerful tools, revolutionizing various industries such as advertising, fashion, entertainment, and healthcare. With a wide range of applications and advancements, these models have changed the way we create, visualize, and interact with images. However, challenges still remain in training complexity, evaluation metrics, and ensuring diversity in generated imagery. As the field progresses, we can expect even greater innovation and exciting artistic creations through the fusion of AI and human creativity.
Frequently Asked Questions
Question 1: What are generative image AI models?
Generative image AI models are computer algorithms designed to generate new images based on existing data. These models use deep learning techniques to learn the patterns and features of a given dataset and produce new, realistic images that resemble the original data.
Question 2: How do generative image AI models work?
Generative image AI models usually consist of two components: a generator network and a discriminator network. The generator network takes random noise as input and generates an image, while the discriminator network tries to distinguish between real and generated images. Through an adversarial training process, both networks improve their performance, resulting in increasingly realistic images generated by the generator network.
Question 3: What are some applications of generative image AI models?
Generative image AI models have various applications, including but not limited to:
- Creating realistic video game environments
- Generating novel images for artistic purposes
- Enhancing image quality and resolution
- Facilitating data augmentation for training other neural networks
- Creating realistic virtual characters and avatars
Question 4: How are generative image AI models trained?
Generative image AI models are typically trained on large datasets containing real images. During training, the models attempt to generate images that are indistinguishable from the real ones. The training process involves iterating through the dataset multiple times, adjusting the model’s parameters, and fine-tuning the network until satisfactory results are achieved.
Question 5: What are some popular generative image AI models?
Some popular generative image AI models include:
- Deep Convolutional Generative Adversarial Networks (DCGANs)
- Progressive Growing of GANs (PGGANs)
- StyleGAN
- CycleGAN
- Variational Autoencoders (VAEs)
Question 6: Are there any limitations or challenges with generative image AI models?
Yes, there are several limitations and challenges with generative image AI models, such as:
- Generating images that lack fine details or exhibit artifacts
- Difficulty in controlling the specific attributes of the generated images
- Generating biased or culturally inappropriate content based on biased training data
- Generating images that may infringe on copyright or intellectual property rights
- High computational and memory requirements for training and inference
Question 7: Can generative image AI models be used for malicious purposes?
Generative image AI models have the potential to be misused for malicious purposes, such as creating deepfakes or generating fake images for misinformation campaigns. It is important to have ethical guidelines and regulations in place to mitigate these risks and prevent misuse.
Question 8: Are there any ethical considerations when using generative image AI models?
Yes, there are several ethical considerations when using generative image AI models. These include ensuring proper attribution and respect for intellectual property rights, addressing biases in training data, and being transparent about the use of AI-generated content to prevent potential deception or misleading information.
Question 9: Can generative image AI models be fine-tuned or customized for specific tasks?
Yes, generative image AI models can be fine-tuned or customized for specific tasks by training them on domain-specific datasets or adjusting the training process. Fine-tuning allows the models to generate images that align with the requirements and constraints of the specific task or application.
Question 10: How can I get started with generative image AI models?
To get started with generative image AI models, you can explore open-source implementations and libraries such as TensorFlow or PyTorch. Online tutorials, courses, and research papers can also provide valuable resources to learn about different models and techniques used in generative image AI.