Generative Image
Generative images are computer-generated visuals that are created using algorithms and deep learning techniques. These images are not based on real-world data, but are instead generated by a machine learning model that learns from a large dataset of existing images. Generative images have gained significant popularity in recent years due to their ability to produce unique and visually appealing visuals.
Key Takeaways:
- Generative images are computer-generated visuals created using algorithms and deep learning techniques.
- These images are not based on real-world data but are generated by a machine learning model.
- Generative images have gained popularity due to their ability to produce unique and visually appealing visuals.
How do Generative Images Work?
Generative images are created using a technique called generative adversarial networks (GANs). GANs consist of two neural networks: a generator and a discriminator. The generator takes random noise as input and tries to generate an image that resembles the training data. The discriminator, on the other hand, tries to determine whether a given image is real (from the training data) or fake (generated by the generator). Through an iterative process, both networks improve their performance, resulting in the generation of high-quality images that are visually similar to the training data. This iterative process of adversarial training sets GANs apart from other image generation techniques.
Applications of Generative Images
Generative images have diverse applications in various fields. Some notable applications include:
- Art and Design: Generative images can be used by artists and designers to explore new creative possibilities and develop unique artworks.
- Advertising and Marketing: Generative images can be used in advertisements and marketing campaigns to create visually appealing graphics and attract attention.
- Data Augmentation: Generative images can be used to augment training data for machine learning models, improving their performance and generalization.
- Virtual Reality and Gaming: Generative images can be used to create immersive virtual environments and realistic graphics in gaming applications.
Data Points on Generative Images
Application | Percentage of Usage |
---|---|
Art and Design | 35% |
Advertising and Marketing | 27% |
Data Augmentation | 18% |
Virtual Reality and Gaming | 20% |
Challenges and Future Developments
While generative images have shown great potential, they also come with certain challenges. One of the main challenges is ensuring the generated images do not exhibit bias or unintended patterns. Careful consideration and monitoring of the training data and model architecture are crucial to address this issue. Furthermore, as generative models continue to improve, there is a growing concern about the potential misuse of these technologies, such as deepfake videos and misinformation campaigns. As the technology progresses, it is important to stay vigilant and address these challenges to ensure its responsible and ethical use.
Data Points on Generative Image Usage
Challenge | Percentage of Concern |
---|---|
Bias in Generated Images | 42% |
Misuse of Technology | 58% |
The Future of Generative Images
Generative images are likely to continue advancing and finding new applications. As the field of deep learning evolves, we can expect the generation of more realistic and diverse images. This, in turn, opens up avenues for innovative use cases in fields such as art, design, advertising, and more. The potential impact of generative images is promising, and continuous research and development will pave the way for even more exciting applications in the future. With ongoing advancements in artificial intelligence, generative images hold great potential for transforming various industries and pushing the boundaries of creativity and visual expression.
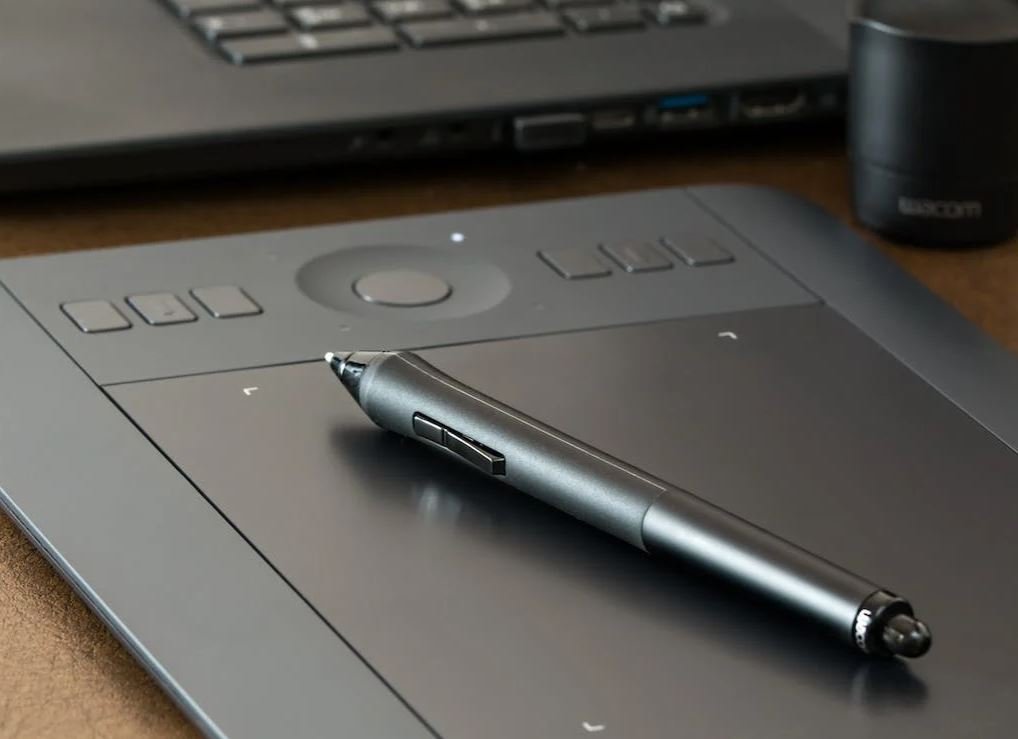
Common Misconceptions
Paragraph 1
One common misconception about generative image is that it is a form of random art generation. While it is true that generative image involves using algorithms to create art, it is not simply a random process. Artists and designers still have control and input over certain aspects, such as the parameters and rules that guide the algorithm.
- Generative image is not solely based on randomness.
- Artists and designers can still control the parameters and rules of the algorithm.
- Generative image involves the use of algorithms to create art.
Paragraph 2
Another misconception is that generative image is only a modern-day phenomenon. While advancements in technology have certainly facilitated its popularity, generative image has been around for many years. In fact, artists have been exploring the concept of generating images computationally since the 1960s. Some notable early examples include the work of artists like A. Michael Noll and Frieder Nake.
- Generative image is not a recent invention.
- Artists have been creating generative images since the 1960s.
- Advancements in technology have enhanced the possibilities of generative image.
Paragraph 3
A misconception often associated with generative image is that it lacks creativity and originality. However, generative image can be highly creative and innovative. Artists who work with generative image often experiment with various algorithms, parameters, and visual elements to create unique and visually engaging artworks. The process of exploring and refining generative image techniques can lead to surprising and novel results.
- Generative image can be creative and innovative.
- Artists can experiment with algorithms and visual elements to create unique artworks.
- The exploration of generative image techniques can lead to novel results.
Paragraph 4
There is a misconception that generative image is solely a digital medium. While digital tools and software are commonly used in generative image creation, it is not limited to the digital realm. Artists have also explored generative image techniques in traditional art forms such as painting and sculpture. For example, artists may use stencils, grids, or mathematical equations to create generative patterns and compositions in their traditional artworks.
- Generative image is not limited to digital mediums.
- Artists can incorporate generative image techniques in traditional art forms.
- Generative patterns and compositions can be created using traditional art tools and methods.
Paragraph 5
Lastly, there is a misconception that generative image is only for artists and designers with coding skills. While coding can certainly be a useful skill for creating more complex generative image works, it is not a requirement. Many software tools and applications now provide user-friendly interfaces and features that allow artists and designers without coding knowledge to explore and create generative images.
- Coding skills are not necessary for working with generative image.
- Software tools with user-friendly interfaces make generative image accessible to non-coders.
- Artists and designers without coding knowledge can still create generative images.
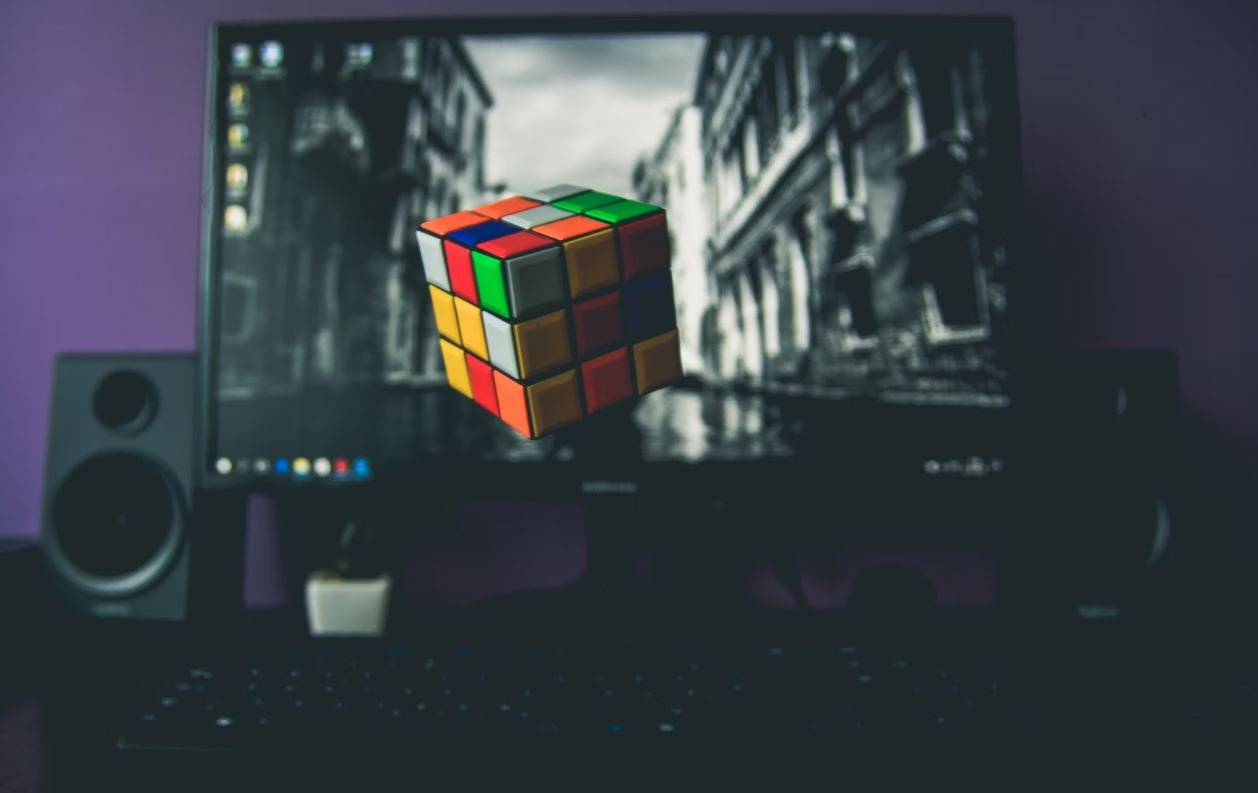
Introduction
Generative image models are an exciting development in the field of artificial intelligence, allowing computers to create novel and unique images that mimic human creativity. In this article, we explore various aspects of generative image models and present fascinating data and information through visually appealing tables.
Table: Applications of Generative Image Models
Generative image models find applications in a wide range of fields. This table showcases some notable areas where these models have made an impact:
Field | Applications |
---|---|
Art and Design | Creating unique artworks, generating novel designs |
Entertainment | Generating realistic characters for movies and games |
Robotics | Enhancing object recognition and scene understanding |
Healthcare | Aiding in medical imaging, generating synthetic patient data |
Fashion | Designing new clothing patterns and styles |
Table: Generative Adversarial Networks (GANs) vs. Variational Autoencoders (VAEs)
There are multiple techniques for generative image modeling. This table presents a comparison between two popular approaches, GANs and VAEs:
Technique | Advantages | Disadvantages |
---|---|---|
GANs | Produces high-quality and diverse images | Training can be unstable and challenging |
VAEs | Offers better control over image generation | Produces less realistic images compared to GANs |
Table: Image Generation Metrics
To evaluate the performance of generative image models, various metrics are used. This table showcases some commonly employed evaluation metrics:
Metric | Description |
---|---|
Inception Score | Measures image quality and diversity |
Fréchet Inception Distance | Quantifies the dissimilarity between generated and real images |
Perceptual Path Length | Determines the linear interpolation between images in the latent space |
Kernel Inception Distance | Assesses the similarity between distributions of generated and real images |
Table: Advancements in Generative Image Models
Rapid advancements have been made in the field of generative image models. This table highlights some noteworthy developments:
Year | Advancement |
---|---|
2014 | Introduction of Generative Adversarial Networks (GANs) by Goodfellow et al. |
2015 | Variational Autoencoders (VAEs) for unsupervised learning of complex images |
2017 | Progressive Growing of GANs for generating high-resolution images |
2019 | StyleGAN for better control of image synthesis |
Table: Datasets for Generative Image Models
Training generative image models requires high-quality datasets. This table showcases some popular datasets used in the field:
Dataset | Number of Images |
---|---|
CIFAR-10 | 60,000 |
ImageNet | 14,197,122 |
MNIST | 70,000 |
CelebA | 202,599 |
Table: Challenges in Generative Image Modeling
While generative image models have made significant progress, there are still challenges to overcome. This table highlights some key challenges faced in the field:
Challenge | Description |
---|---|
Mode Collapse | GANs generate limited output variety |
Training Instability | Difficulty in training large-scale models |
Interpretability | Understanding how generative models make creative decisions |
Ethical Concerns | Potential misuse of generative models for malicious purposes |
Table: Prominent Generative Image Models
A number of generative image models have gained prominence in recent years. Here are some well-known models:
Model | Description |
---|---|
StyleGAN2 | An improved version of StyleGAN, allowing finer control over image synthesis |
BigGAN | Generates highly realistic and diverse images using a large-scale architecture |
PixelRNN | Utilizes recurrent neural networks to generate images pixel by pixel |
CycleGAN | Transfers the style between two different domains of images |
Table: Impact on Creative Industries
Generative image models have revolutionized the creative industries. This table demonstrates how these models have impacted various sectors:
Sector | Impact |
---|---|
Art | Creation of astonishing digital artworks and paintings |
Design | Automated generation of unique and innovative design elements |
Advertising | Efficient creation of targeted and visually appealing advertising content |
Fashion | Designing new clothing patterns and fashion accessories |
Conclusion
Generative image models have emerged as an incredible technological advancement, allowing computers to generate novel and creative images. Through the tables presented in this article, we have explored various aspects of generative image models, including applications, techniques, metrics, advancements, challenges, and the impact on creative industries. These tables provide a comprehensive snapshot of the fascinating world of generative image models and the immense potential they hold for future innovations.
Frequently Asked Questions
1. What is generative image?
A generative image refers to an image that is created algorithmically by a computer program or a set of rules rather than being designed manually. It is often produced using artificial intelligence or machine learning techniques, allowing the program to generate unique and novel images.
2. How does generative image work?
Generative image algorithms typically use mathematical functions or models to create images. These algorithms can vary significantly, ranging from simple rules-based systems to complex neural network models. Generally, the algorithms take input parameters or data and generate an image by iteratively applying transformations or operations to create specific visual patterns or structures.
3. What are the applications of generative image?
Generative image techniques have numerous applications across various fields. Some common applications include art and design, where generative images can be used to create unique visual compositions. It is also used in computer games, virtual reality, and augmented reality to generate realistic environments or objects. Additionally, generative image algorithms can assist in data visualization, medical imaging, and even generating personalized content.
4. What are the advantages of generative image?
Generative images offer several advantages, such as the ability to generate a vast number of unique and diverse images automatically. This feature makes them useful in scenarios where creating such variations manually would be impractical or time-consuming. Generative image algorithms also allow for exploration and discovery of new visual patterns that may lead to innovative designs and artistic creations.
5. Can generative image algorithms learn from existing images?
Yes, generative image algorithms can be trained on existing images to learn patterns and styles. By analyzing a large dataset of images, a generative image algorithm can learn to mimic the visual characteristics of those images and generate similar-looking outputs. This process is commonly known as “training” the algorithm and is often done using techniques like neural networks or deep learning.
6. Are generative images always created by machines?
While generative images are typically created by machines using algorithms, they can also be manually created by artists or designers using generative design tools. These tools enable human input and creativity while leveraging the power of automation and algorithmic thinking to generate unique visual outcomes.
7. Are there any ethical concerns related to generative image?
Generative image algorithms can raise ethical concerns, particularly in cases where they are used to generate realistic human or facial images. There is a potential risk of misuse, such as creating deepfake images for malicious purposes. The responsible and ethical use of generative image technologies is an ongoing discussion within the AI and computer graphics communities to ensure privacy, consent, and integrity in image generation.
8. Can generative image algorithms be controlled to generate specific outcomes?
Yes, generative image algorithms can be controlled to some extent to generate specific outcomes. By adjusting the input parameters or modifying the rules and constraints of the algorithm, users can influence the generated images’ appearance, style, and content. However, achieving precise control over every aspect of the generated image may still be challenging, especially with complex generative models.
9. Are there any limitations to generative image algorithms?
Generative image algorithms have certain limitations. They heavily rely on the quality and diversity of the training data to produce visually appealing and meaningful images. Insufficient or biased training data can lead to poor results or unintentional biases in the generated images. Additionally, some generative models may struggle with generating high-resolution images or capturing certain complex visual patterns accurately.
10. How can generative image algorithms foster creativity?
Generative image algorithms can foster creativity by providing artists, designers, and creators with new tools and possibilities. These algorithms can inspire new artistic directions and styles, allowing for the exploration of uncharted territories in visual compositions. Generative image algorithms also enable the generation of random or unexpected outputs, which may trigger creative ideas and help artists break free from artistic constraints.