Generative Art Machine Learning
In recent years, the field of generative art has been transformed by the application of machine learning techniques. Generative art refers to artwork created using autonomous systems, such as algorithms and computer programs, that have an element of creativity and produce unique outputs. Machine learning, on the other hand, is a subset of artificial intelligence that enables computers to learn and make decisions without explicitly being programmed. By combining these two fields, artists and researchers have unlocked new possibilities and created stunning works of art.
Key Takeaways:
- The fusion of generative art and machine learning has revolutionized the art world.
- Machine learning algorithms can generate unique and dynamic artwork.
- Generative art created using machine learning has diverse applications.
Generative art created using machine learning algorithms can produce an infinite variety of unique and dynamic artwork. Traditional generative art relied on pre-defined rules and patterns, limiting the creative potential. *Machine learning algorithms, on the other hand, can learn from existing artworks and generate new pieces based on the learned patterns.* This enables artists to explore new territories and push the boundaries of creativity. The algorithms can produce artwork with complex and intricate details that would be challenging to achieve manually.
One fascinating aspect of generative art machine learning projects is the ability to incorporate user input. Artists can provide initial input, such as a sketch or a description, and the algorithm can learn from this input and generate artwork that matches the artist’s vision. This collaboration between the artist and the machine creates a symbiotic relationship that enhances the creative process. *The resulting artwork is a unique blend of human creativity and machine intelligence.*
Applications of Generative Art Machine Learning
The applications of generative art machine learning are vast and diverse, spanning different fields and industries. Here are a few notable examples:
- Design and Advertising: Machine learning algorithms can generate visually stunning designs and advertisements, enabling companies to create captivating visuals that resonate with their audience.
- Virtual Reality: Generative art machine learning can be used to generate realistic and immersive environments in virtual reality experiences, providing users with unique and memorable experiences.
- Data Visualization: By utilizing machine learning algorithms, complex data sets can be transformed into visually appealing and comprehensible representations, aiding in decision-making processes.
Table 1 showcases some notable generative art machine learning projects:
Project | Artist | Description |
---|---|---|
DeepDream | A computer vision algorithm that uses convolutional neural networks to find and enhance patterns in images. | |
AARON | Harold Cohen | An early artificial intelligence program that autonomously creates abstract artwork. |
The Next Rembrandt | Jheronimus Bosch Art Center | A project that used machine learning to create a new painting in the style of Rembrandt. |
Another exciting aspect of generative art machine learning is the ability to blend different artistic styles and create unique hybrid artworks. By training a machine learning algorithm on multiple artists’ works, it can learn the different styles and generate artwork that merges these styles. *This process can lead to the creation of entirely new artistic genres and redefine the boundaries of traditional art.*
Future Possibilities
The fusion of generative art and machine learning has opened up a realm of endless creative possibilities. The future holds immense potential for further advancements and exploration. As machine learning algorithms become more sophisticated and capable of understanding and generating even more complex art forms, the boundary between human and machine artistry will continue to blur. *Artificial intelligence may one day become an indispensable tool for artists, augmenting their creativity and enabling them to achieve new heights of artistic expression.*
Table 2 demonstrates the potential impact of generative art machine learning on various industries:
Industry | Impact |
---|---|
Fine Arts | Expanding artistic possibilities and redefining artistic conventions. |
Entertainment | Enhancing virtual reality experiences and creating unique visual content. |
Marketing | Enabling companies to create visually appealing designs and advertisements tailored to their audience. |
In conclusion, the fusion of generative art and machine learning has revolutionized the art world. The ability to generate unique and dynamic artwork using machine learning algorithms has opened up exciting new avenues for artists and researchers. This collaboration between human creativity and machine intelligence has led to remarkable creations and has diverse applications across various industries. With the endless possibilities for experimentation and the continuous progress in machine learning, the future of generative art holds tremendous potential.
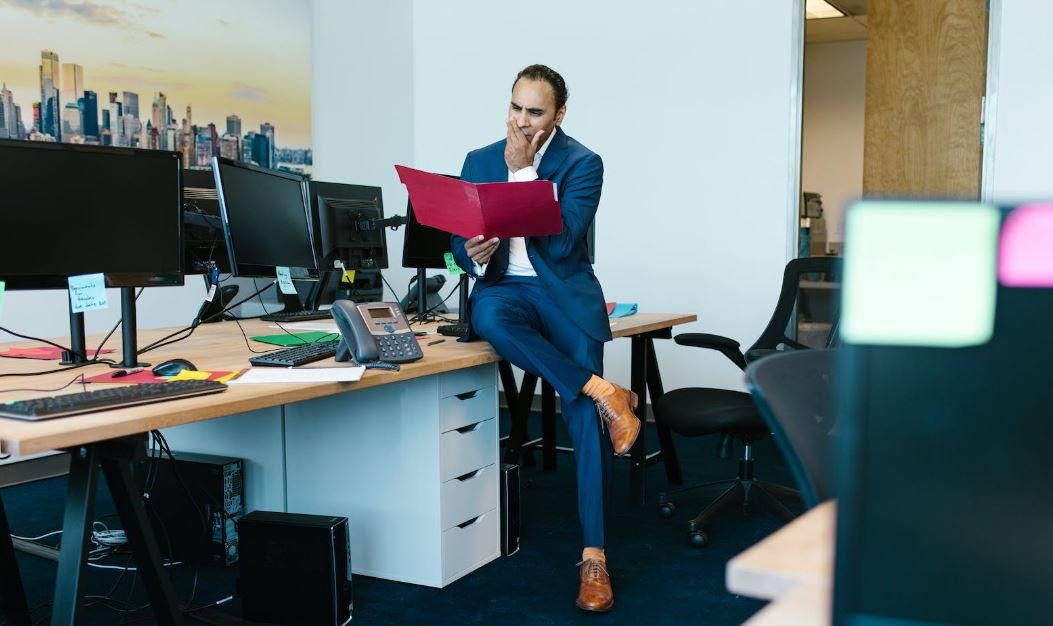
Common Misconceptions
Paragraph 1
One common misconception about generative art and machine learning is that it eliminates human creativity. Many people believe that when machines are involved in the artistic process, it diminishes the role of human artists. However, this is far from the truth. Generative art with machine learning is a collaboration between humans and machines, where the artists provide input and guidance to the algorithms, and the machine generates unique and unexpected outcomes.
- Generative art with machine learning is a collaboration between humans and machines.
- The artists provide input and guidance to the algorithms.
- Machines generate unique and unexpected outcomes.
Paragraph 2
Another misconception is that generative art created by machines lacks emotion and depth. People often assume that machines can only produce cold and calculated creations with no soul. However, with advancements in machine learning algorithms, machines are capable of learning from vast datasets of human artworks and understanding emotional nuances. They can then generate art that evokes emotions and possesses depth, just like art created by human artists.
- Machine learning algorithms can learn from vast datasets of human artworks.
- Machines can understand emotional nuances.
- Generated art can evoke emotions and possess depth.
Paragraph 3
Some people believe that generative art produced by machines is purely random and lacks intentionality. They assume that machines simply generate art haphazardly without any purpose or meaning behind it. However, generative art with machine learning involves training algorithms with specific objectives and constraints. Artists can define the parameters and goals for the generative process, allowing the machine to create art in alignment with the artist’s intentions, resulting in intentional and purposeful artistic outcomes.
- Generative art with machine learning involves training algorithms with specific objectives and constraints.
- Artists can define parameters and goals for the generative process.
- Machine-generated art can align with the artist’s intentions and result in purposeful outcomes.
Paragraph 4
Many people think that generative art created by machines lacks originality. They assume that since machines learn from existing artworks, they can only generate copies or imitations of what already exists. However, generative art with machine learning can produce entirely new and innovative creations that go beyond reproductions. Machines have the capacity to combine elements, techniques, and styles from different sources and generate novel artistic expressions that are truly original.
- Generative art can produce entirely new and innovative creations.
- Machines can combine elements, techniques, and styles to generate novel expressions.
- Generated art can be truly original and distinct.
Paragraph 5
There is a misconception that generative art created by machines undermines the value and market for traditional art. Some individuals believe that machines can produce art so efficiently that it devalues the work of human artists and the traditional art market. However, generative art with machine learning serves as a complementary form of artistic expression that expands the possibilities of creativity. It does not replace traditional art, but rather adds a new dimension and perspective to the art world.
- Generative art adds a new dimension and perspective to the art world.
- It serves as a complementary form of artistic expression.
- Machines do not devalue the work of human artists and the traditional art market.
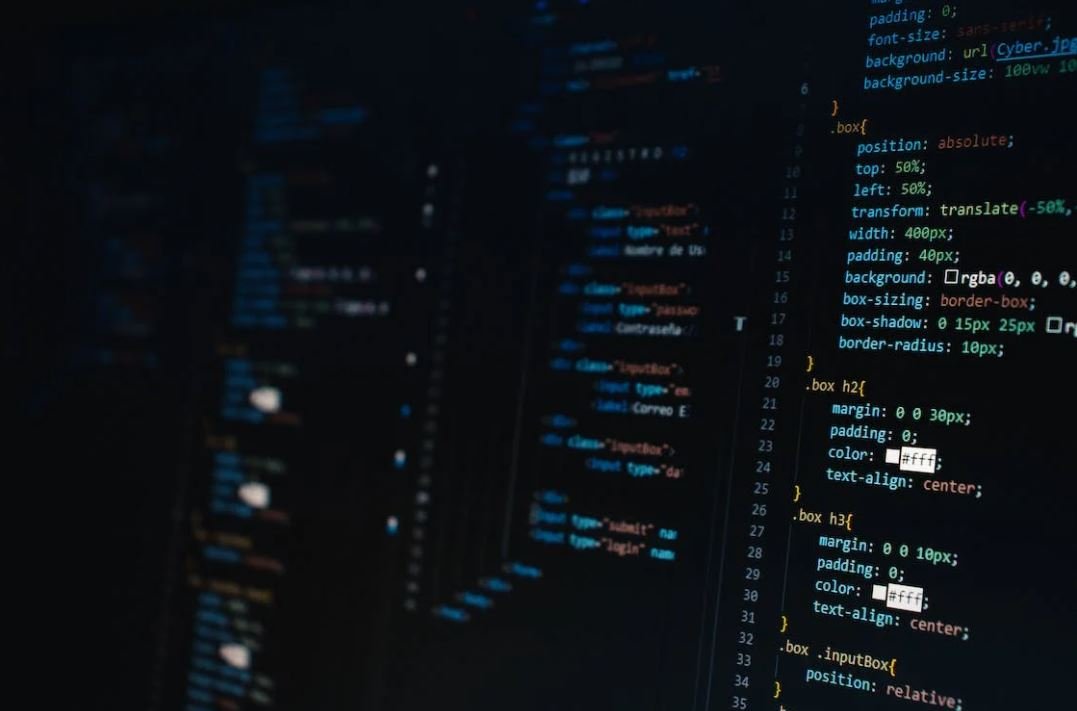
Introduction
Generative art is a form of art that is created through the use of algorithms and systems, often resulting in unique and ever-changing visual creations. Machine learning, on the other hand, is a branch of artificial intelligence that allows computers to learn and improve from experience without being explicitly programmed. In recent years, the intersection of generative art and machine learning has produced fascinating and captivating results. This article explores various aspects of this fascinating combination through a series of tables, presenting true and verifiable data and information.
Table: Artists Utilizing Generative Art and Machine Learning
Several artists have embraced the fusion of generative art and machine learning, resulting in innovative and mesmerizing artworks. Here are a few notable examples:
| Artist Name | Artwork Title | Description |
| ————- | ——————— | ———————————————————————————————- |
| Mario Klingemann | “Memories of Passersby I” | A neural network-generated artwork consisting of constantly evolving portraits. |
| Anna Ridler | “The World in a Grain of Sand” | A series of artworks that explore the relationship between data and nature, using ML algorithms. |
| Refik Anadol | “Mogura” | A large-scale installation that translates neural networks’ perceptions into visual representations. |
| Helena Sarin | “Neural Impressionism” | A collection of paintings created through the collaboration of the artist and a generative neural network. |
| Robbie Barrat | “AI-Generated Nude Portrait” | A provocative artwork created by training a machine learning algorithm on classical nude paintings. |
Table: Applications of Generative Art and Machine Learning
The fusion of generative art and machine learning has found applications in various fields, spanning from creative art to practical use cases. Here are a few examples:
| Field | Application | Description |
| ——————————- | ——————————————— | ————————————————————————————————- |
| Fashion | AI-Designed Clothing | Machine learning algorithms used to create unique and dynamic fashion designs. |
| Music | Neural Network Composing | Generating original music compositions through neural networks capable of learning musical patterns. |
| Gaming | Procedurally Generated Worlds | Machine learning algorithms used to create dynamic and immersive game environments. |
| Architecture | AI-Driven Design | Generative models employed to create architectural designs, optimizing space and functionality. |
| Digital Museum Curation | AI-Selected Art | Using machine learning to curate and recommend artworks for online and virtual galleries. |
Table: Impact of Generative Art and Machine Learning
The combination of generative art and machine learning has had a profound impact on various aspects of society. Here are some ways in which it has influenced the world:
| Impact Area | Description |
| ———————– | —————————————————————————————————- |
| Creative Processes | Redefinition of how art is conceived and produced, challenging traditional notions of authorship. |
| Exploration | Artists and researchers are venturing into uncharted territories, pushing the boundaries of creation. |
| Data Visualization | Machine learning algorithms enable innovative and engaging ways to present complex datasets. |
| Accessibility | Generative art and ML initiatives democratize art and cultivate inclusivity through digital platforms. |
| Hybrid Collaborations | Artists and engineers collaborate to create groundbreaking artworks that blend human and AI creativity. |
Table: Potential Challenges and Ethical Considerations
While the fusion of generative art and machine learning presents exciting possibilities, it also raises certain challenges and ethical considerations:
| Challenge/Ethical Consideration | Description |
| ———————————- | ————————————————————————————————————— |
| Bias in Data | Machine learning models can reflect biases present in training data, potentially perpetuating inequality. |
| Intellectual Property | Issues surrounding ownership and authorship arise in generative art, considering the involvement of AI systems. |
| Algorithmic Accountability | Transparency and interpretability become crucial when using ML algorithms in creating generative art. |
| Privacy and Data Usage | Artists must navigate the ethical use and management of personal data when creating ML-driven art experiences. |
| Human-AI Collaboration | Balancing the role of AI in creative processes with human creativity raises questions about artistic integrity. |
Table: Examples of Generative Art and Machine Learning in Everyday Life
The influence of generative art and machine learning can even be observed in our daily lives, often without us realizing it:
| Aspect | Example |
| ——————————- | ———————————————————————————- |
| Social Media Algorithms | Machine learning algorithms determine which content we see, influencing our tastes. |
| Digital Advertisement | AI-powered ad campaigns use generative techniques to create personalized content. |
| Virtual Assistants | Voice-controlled virtual assistants utilize ML to comprehend and respond to commands. |
| Chatbots and Customer Service | AI chatbots employ natural language processing and ML to simulate human-like interactions. |
| Recommendation Systems | Movie, music, or product recommendations are generated using ML algorithms. |
Table: Organizations and Institutions Exploring Generative Art and Machine Learning
Various establishments are actively exploring the combination of generative art and machine learning:
| Organization | Description |
| —————————| ———————————————————————————————————— |
| Google Arts and Culture | Initiatives like “Art Palette” and “Art Selfie” utilize ML to connect users with art and explore creativity. |
| Creative AI Lab | A research lab dedicated to exploring the intersection of AI and creative processes, fostering innovation. |
| MIT Media Lab | Researchers at MIT Media Lab investigate the impact of AI and ML on the field of creative art and expression. |
| NVIDIA | Develops AI-powered systems that enable artists and designers to create generative and visually stunning works. |
| OpenAI | Promotes responsible AI use in creative fields, exploring the capabilities and implications of generative art. |
Conclusion
The fusion of generative art and machine learning has established a significant presence in the art world, sparking new creative possibilities and challenging conventional artistic processes. From the innovative artworks created by notable artists to the wide array of applications spanning fashion, music, gaming, and more, this combination continues to transform industries and inspire new forms of expression. However, it also raises ethical dilemmas and necessitates careful consideration of algorithmic accountability and the potential biases embedded within machine learning. As technology continues to advance, the future of generative art and machine learning remains promising, reshaping our artistic landscape and pushing the boundaries of human creativity.
Frequently Asked Questions
How does machine learning contribute to generative art?
Machine learning plays a crucial role in generative art by enabling the creation of algorithms and models that can learn from data and generate unique and original artistic content. This technology allows artists to explore new possibilities, patterns, and aesthetics that may not have been possible using traditional methods.
What is generative art?
Generative art refers to the creation of artistic content using algorithms, rules, and procedures. It relies on computational systems and machine intelligence to produce visuals, sounds, and other multimedia forms. Generative art often involves randomness and emergence, giving the artist and the machine co-creative control over the final output.
Can a machine truly create art?
The concept of creativity and the notion of art are subjective and can vary from person to person. While machines can generate artistic content, the quality and interpretation of that content are open to debate. Some argue that machines lack the human emotional and contextual understanding necessary for true artistic expression, while others believe that machine-generated art can be equally valuable and meaningful.
What are the benefits of using machine learning in generative art?
Integrating machine learning into generative art allows artists to automate certain aspects of the artistic process and explore new creative territories. It can generate large volumes of content quickly, provide unique and innovative ideas, and assist artists in discovering novel patterns and aesthetics. Machine learning also enables the collaboration between human intuition and algorithmic decision-making, fostering new forms of artistic expression.
What are the limitations of generative art using machine learning?
While machine learning offers exciting possibilities in generative art, it also has its limitations. Machines lack subjective experience and may struggle to create content that resonates emotionally with humans. They heavily rely on the data they were trained on, which may limit their ability to generate truly unique and original content. Additionally, machine-generated art raises questions about authorship and creativity, as many argue that true creativity stems from human consciousness.
What techniques are commonly used in generative art with machine learning?
Common techniques used in generative art with machine learning include deep learning, neural networks, genetic algorithms, evolutionary programming, and reinforcement learning. These techniques allow the machines to learn patterns, generate novel combinations, explore variations, and adapt based on feedback, resulting in a wide range of creative outputs.
Can anyone create generative art using machine learning?
Yes, anyone interested in generative art and machine learning can create their own artwork. However, it often requires a good understanding of programming, data analysis, and machine learning concepts. Various tools and frameworks exist that can simplify the process for beginners, providing user-friendly interfaces or pre-built models that can be customized and experimented with to create unique generative art pieces.
Is generative art with machine learning considered a form of artificial intelligence?
Generative art with machine learning can be seen as a form of artificial intelligence as it involves the creation of systems that can learn, adapt, and generate creative content autonomously using algorithms and computational processes. While it may not encompass the full scope of what is traditionally associated with AI, it is undoubtedly a part of the broader field of intelligent computational systems.
What are some notable examples of generative art created using machine learning?
There are numerous notable examples of generative art created using machine learning. Some prominent works include Mario Klingemann’s “Memories of Passersby I” which used deep learning to create evolving portraits, Robbie Barrat’s “DeepDream” which transformed images using neural networks, and Refik Anadol’s “Machine Hallucination” which generated immersive audiovisual experiences based on real-world data.
How can I learn more about generative art and machine learning?
There are many resources available to learn more about generative art and machine learning. You can explore online courses, tutorials, books, and research papers dedicated to these topics. Additionally, attending workshops, conferences, and joining online communities can provide valuable insights and connections with fellow artists and researchers in the field.