Generative AI Landscape Image
Generative Artificial Intelligence (AI) capable of creating landscape images is an emerging technology that has gained significant attention in recent years. Leveraging deep learning algorithms and massive amounts of data, generative AI can generate visually stunning and realistic landscapes, enhancing various applications such as video games, virtual reality, and architectural design.
Key Takeaways
- Generative AI is an emerging technology that produces realistic landscape images.
- Deep learning algorithms and vast datasets enable generative AI to create visually stunning landscapes.
- Applications of generative AI in gaming, virtual reality, and architecture have significant potential.
The rapid advancement of generative AI is transforming the way landscapes are generated digitally. *With the ability to learn patterns and features from vast datasets, generative AI models can generate landscapes that rival photographs.* This technology has opened up exciting possibilities for industries such as video game development, where realistic and immersive environments are crucial for engaging gameplay.
Generative AI has also found applications in virtual reality (VR), where visually appealing environments are essential for providing a realistic and immersive experience. *By using generative AI, developers can create lifelike VR environments without the need for extensive manual design.* This not only saves time but also allows for more diverse and imaginative landscapes that can enhance the user’s experience.
Applications of Generative AI in Landscape Design
Generative AI has significant potential in landscape design and architecture. By leveraging generative models, architects and designers can explore various design options and visualize them in lifelike representations. This technology allows for quick iterations and experimentation, enabling professionals to create unique and innovative landscapes that meet specific project requirements. *Generative AI can also help architects incorporate natural elements and environmental considerations into their designs, promoting sustainable practices.*
To further highlight the capabilities of generative AI in landscape image generation, let’s explore some interesting data points and examples:
Application | Example |
---|---|
Video Game Development | A generative AI-powered game that dynamically generates vast and visually impressive landscapes for players to explore. |
Virtual Reality | A generative AI-driven virtual reality experience that transports users to breathtaking landscapes, making them feel present in diverse environments. |
- Generative AI enables fast and efficient creation of diverse landscape designs for architecture and urban planning.
- It allows for the integration of natural elements and sustainable practices in landscape design.
- Generative AI can produce realistic and immersive environments for video game development and virtual reality experiences.
Advantages | Challenges |
---|---|
|
|
As generative AI continues to advance, its potential impact on landscape image generation across various industries becomes increasingly evident. From video games to virtual reality and architecture, *this technology is revolutionizing the way realistic landscapes are created and experienced.* The future holds tremendous possibilities for generative AI, and we can only imagine the incredible landscapes it will generate.
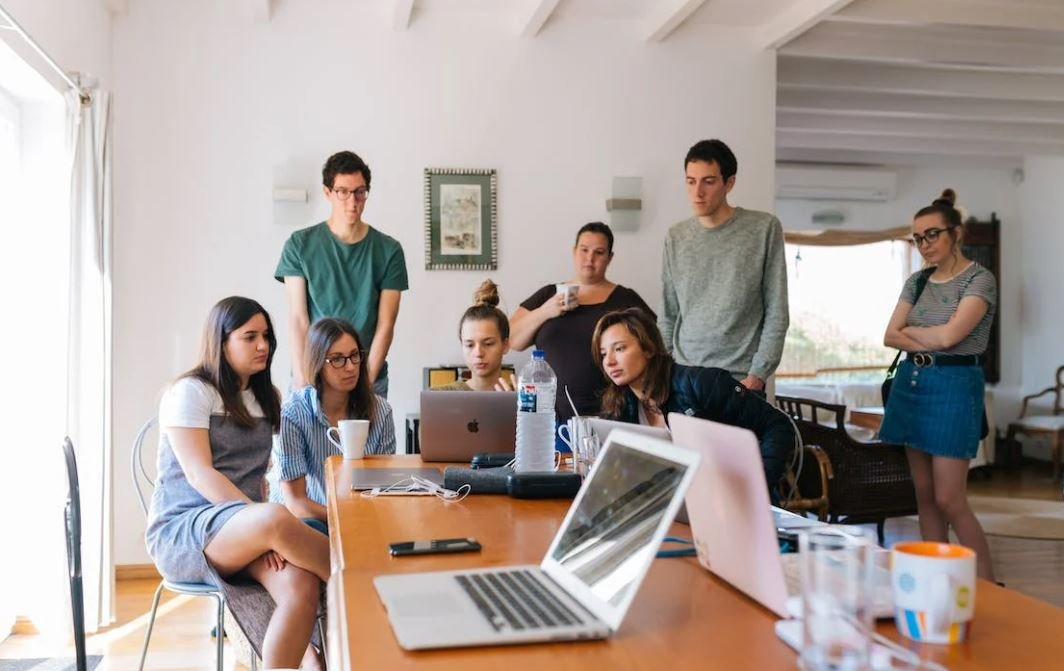
Common Misconceptions
Generative AI Landscape Image
There are several common misconceptions surrounding the topic of generative AI landscape images. These misconceptions often lead to misinformation and a lack of understanding of the capabilities and limitations of this technology. It’s important to clear up these misconceptions to provide a more accurate understanding of generative AI landscape images.
- Generative AI landscape images are 100% computer-generated and do not involve any real photographs.
- Generative AI landscape images cannot accurately represent real-world scenes and are solely creative outputs.
- Generative AI landscape images are only useful for artistic purposes and have no practical applications.
Firstly, one common misconception is that generative AI landscape images are 100% computer-generated and do not involve any real photographs. In reality, many generative AI models utilize a combination of real photographs as training data to create realistic landscapes. These models learn from real-world data and generate new images based on patterns and features extracted from the training dataset.
- Generative AI landscape images often incorporate elements from various real photographs to enhance their realism.
- Real photos are used in a generative AI model’s training process to capture realistic details.
- The use of real photographs helps generative AI landscape images maintain a closer resemblance to real-world scenes.
Secondly, generative AI landscape images cannot accurately represent real-world scenes and are solely creative outputs. Although generative AI images are inherently creative outputs, modern models have advanced to the point where they can generate highly realistic landscapes that closely resemble actual scenes. With the utilization of real photograph datasets and sophisticated algorithms, generative AI models can create landscape images that possess intricate details and nuanced characteristics found in real-world environments.
- Generative AI models are capable of capturing and incorporating realistic details such as textures, lighting, and weather patterns.
- The generated landscapes can resemble real-world scenes in terms of composition and overall appearance.
- Generative AI landscape images can serve as reference material for artists, designers, and architects looking to visualize concepts or inspire creativity.
Lastly, a common misconception is that generative AI landscape images are only useful for artistic purposes and have no practical applications. While these images do hold significant value in creative fields, they also have practical applications in various industries. For instance, generative AI landscape images can help urban planners or architects visualize potential urban development projects or assist in environmental studies by simulating the effects of climate change on landscapes.
- Generative AI landscape images can be utilized in movies, video games, and virtual reality experiences to create immersive and realistic virtual worlds.
- These images can aid in the visualization and planning of real-world projects, such as urban development or landscaping designs.
- Generative AI landscape images can contribute to research and scientific studies in areas like geographic mapping, climate simulations, and environmental impact assessments.
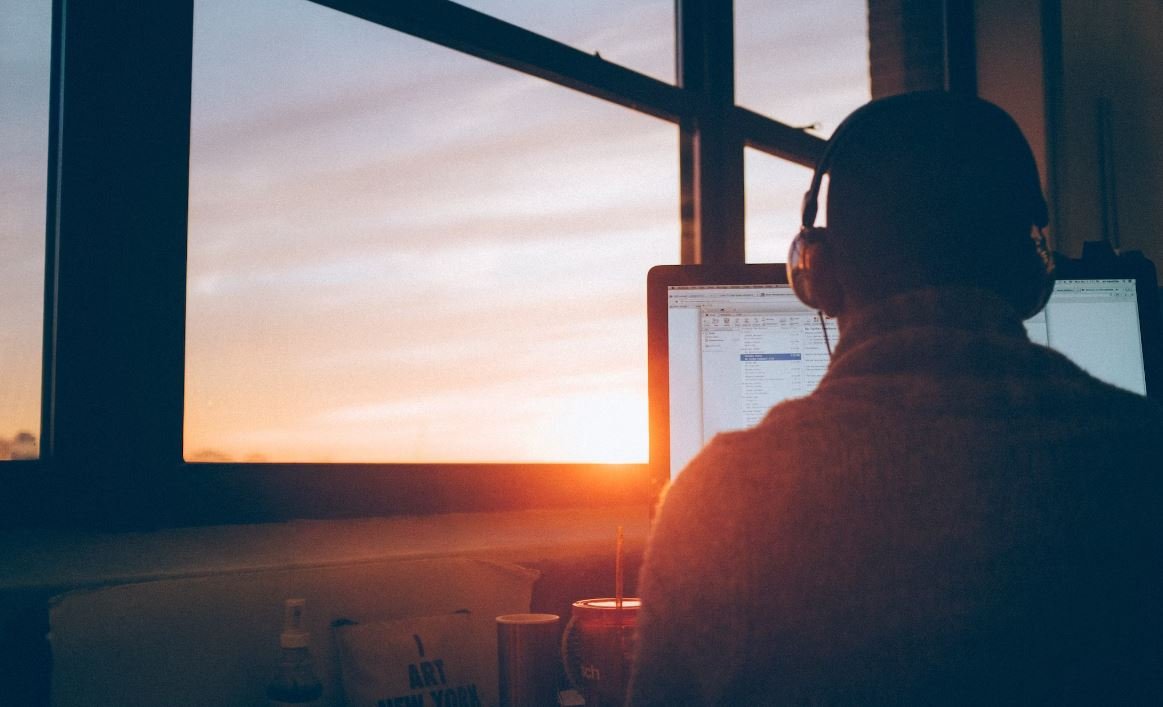
Introduction
In this article, we will explore the fascinating world of generative AI and its implications for landscape image generation. Generative AI refers to the use of artificial intelligence algorithms to create new and original content, such as images, music, or text. Specifically, we will dive into the landscape image domain and examine various aspects of this emerging field. Here are ten interesting tables that showcase different elements and facts related to the generative AI landscape image.
Table: Different Types of Landscape Images Generated by AI
A comprehensive overview of the various types of landscape images that can be generated using AI algorithms. Each image type showcases the unique capabilities of generative AI in producing diverse and realistic landscapes.
Landscape Type | Description |
---|---|
Mountainous Terrain | Images depicting majestic mountain ranges with towering peaks and lush valleys. |
Coastal Sceneries | Seascapes featuring serene beaches, crashing waves, and stunning sunsets. |
Enchanting Forests | Captivating woodland scenes with towering trees, dappled sunlight, and vibrant foliage. |
Desert Landscapes | Empty dunes, arid canyons, and rocky outcrops that transport you to the desert. |
Urban Cityscapes | Artificially generated landscapes boasting futuristic city skylines and technological marvels. |
Table: Top Generative AI Models Used for Landscape Generation
A compilation of the most influential and widely adopted generative AI models employed to generate stunning landscape images.
Model | Year Released | Description | Notable Features |
---|---|---|---|
DeepArt | 2015 | Uses deep learning techniques to create intricate and realistic landscape artworks. | High-resolution output, fine-grained details |
GANPaint Studio | 2018 | Enables users to interactively modify and render generative landscape images. | Real-time editing, customizable elements |
BigGAN | 2019 | A state-of-the-art generative model for high-resolution landscape synthesis. | Sharp and realistic image quality, diverse landscapes |
StyleGAN | 2019 | Produces highly realistic landscape images with configurable styles and characteristics. | Control over image appearance, diverse output |
DeepDream | 2015 | Alters existing images to generate dreamlike landscapes with surreal elements. | Trippy artistic effects, unexpected imagery |
Table: Impact of Generative AI in Landscape Photography
An exploration of how generative AI has revolutionized landscape photography, providing photographers with new tools and opportunities.
Impact | Description |
---|---|
Augmented Creativity | Gives photographers the ability to expand their creative horizons by generating novel landscapes. |
Exploration of Possibilities | Allows for the exploration of uncharted territories and non-existent locations. |
Time and Cost Savings | Reduces the need for extensive travel and expensive equipment, making landscape photography more accessible. |
Training Tool | Provides a valuable learning resource for aspiring landscape photographers. |
Artistic Collaboration | Fosters collaborative efforts between photographers and AI systems to create unique visual masterpieces. |
Table: Ethical Considerations in AI-Generated Landscape Images
An overview of the ethical considerations surrounding the use of AI-generated landscape images.
Consideration | Description |
---|---|
Intellectual Property | Ownership of AI-generated images and the rights of the original creator. |
Misrepresentation | Addressing the potential for AI-generated landscapes being portrayed as real places. |
Cultural Sensitivity | Ensuring that AI-generated landscapes respect cultural and historical contexts. |
Environmental Impact | Considering the ecological implications of generating landscapes that may not exist or altering perceptions of real environments. |
Data Privacy | Protecting the privacy of individuals or locations captured in AI-generated landscape images. |
Table: Applications of AI-Generated Landscape Images
A glimpse into the diverse applications of AI-generated landscape images beyond traditional photography.
Application | Description |
---|---|
Virtual Reality Experiences | Enhancing virtual reality environments by rendering realistic landscapes. |
Video Game Development | Creating immersive virtual worlds by generating expansive landscapes. |
Movie and Animation Industry | Augmenting visual effects by generating realistic landscapes for films and animated features. |
Architectural Visualization | Helping architects and designers by generating visualizations of landscapes for building projects. |
Tourism and Advertising | Generating captivating landscapes to entice travelers and promote destinations. |
Table: Challenges in Generative AI Landscape Image Synthesis
Identifying the key challenges faced when generating landscape images using generative AI techniques.
Challenge | Description |
---|---|
Realism | Ensuring generated landscapes are visually convincing and indistinguishable from real photographs. |
Diversity | Generating a wide range of unique landscapes rather than producing similar results. |
Consistency | Maintaining coherence and consistency across different elements in the landscape image. |
Training Data Bias | Avoiding biases and limitations present in the training dataset that could influence the generated landscapes. |
User Control | Providing users with sufficient control over the generated image to match their preferences or requirements. |
Table: Future Perspectives in Generative AI Landscape Image
Highlighting some exciting future prospects and possibilities within the field of generative AI for landscape image generation.
Perspective | Description |
---|---|
Photorealistic Landscapes | Advancing AI algorithms to generate landscapes that are virtually indistinguishable from real photographs. |
Creative Collaboration | Enabling AI systems to co-create landscapes alongside human artists, pushing the boundaries of creativity. |
Real-Time Augmentation | Implementing AI algorithms that can instantly modify and enhance landscape images captured by photographers. |
Artistic Style Transfer | Allowing users to apply various artistic styles to AI-generated landscape images, mimicking famous painters or art movements. |
Landscape Animation | Expanding the capabilities of generative AI to create dynamic and animated landscapes. |
Conclusion
Generative AI has rapidly transformed the landscape image generation domain, empowering photographers, and fostering collaboration between humans and algorithms. The tables presented throughout this article depict a variety of facets in the generative AI landscape, from the types of landscapes that can be synthesized to the associated ethical considerations. As the field progresses, it holds the potential to revolutionize not only artistic endeavors but also various industries, offering exciting applications and pushing the boundaries of what is possible with AI-generated landscapes.
Frequently Asked Questions
What is generative AI?
Generative AI refers to the technology that enables machines to create or generate new content, such as images, music, or text, similar to human-like behavior. It uses algorithms and datasets to learn patterns and generate output that is not explicitly programmed.
How does generative AI work?
Generative AI works by using neural networks, specifically generative models, which learn from training data to create new content. These models are trained on large datasets and utilize various techniques like deep learning, reinforcement learning, or adversarial training to generate output.
What are the applications of generative AI?
Generative AI has a wide range of applications across various domains. It can be used for content creation, image or music synthesis, data augmentation, chatbots, virtual reality, and even in scientific research, medicine, and design.
What is the difference between generative AI and traditional AI?
Traditional AI focuses on solving specific tasks or problems using rule-based systems or pre-defined algorithms. Generative AI, on the other hand, focuses on learning patterns from training data and creating new content or output that is not explicitly programmed. It relies on neural networks and deep learning techniques.
Can generative AI be used for malicious purposes?
While generative AI has great potential for positive applications, it can also be misused. For example, it can be used to create deepfake images or videos, generate fake news, or spread disinformation. Therefore, proper ethical considerations and regulations are necessary to prevent misuse.
What are some popular generative AI models?
There are several popular generative AI models, such as Generative Adversarial Networks (GANs), Variational Autoencoders (VAEs), and Transformer-based models like OpenAI’s GPT (Generative Pre-trained Transformer). These models have shown impressive results in generating realistic images, text, and music.
What are the challenges in generative AI?
Generative AI still faces challenges, such as generating high-quality and diverse output, addressing biases learned from training data, and interpreting the generated output. It also requires substantial computational resources and data to train the models effectively.
What are some future possibilities of generative AI?
Generative AI has the potential to revolutionize various industries. It could be used for personalized content creation, artistic expression, virtual world generation, improving healthcare outcomes, or even facilitating creativity in robotics and automation.
How can generative AI benefit businesses?
Generative AI can benefit businesses in multiple ways. It can automate content creation processes, provide personalized recommendations or ads to customers, enhance data augmentation, and contribute to innovative product designs. It can also assist in generating synthetic data for research or testing purposes.
Can generative AI replace human creativity?
No, generative AI is not intended to replace human creativity. Instead, it can be seen as a tool to augment and support human creativity. It can assist in generating ideas, inspiration, and providing a starting point for creative processes, but the human touch and judgment are still essential for truly creative outcomes.
“`