Generative AI Image Processing
Generative AI algorithms are revolutionizing image processing and taking it to new heights. Using deep learning techniques, these algorithms have the ability to generate and manipulate images with astonishing realism and creativity. Whether it’s enhancing the quality of images, creating original artwork, or transforming images into different styles, generative AI image processing has endless possibilities.
Key Takeaways
- Generative AI algorithms use deep learning techniques to generate and manipulate images.
- They can enhance image quality, create original artwork, and transform images into different styles.
- Generative AI image processing has various applications in fields like art, entertainment, and advertising.
- It requires large datasets and significant computational resources for training.
Generative AI image processing relies on powerful deep learning models, such as GANs (Generative Adversarial Networks) and VAEs (Variational Autoencoders). These models are trained on vast datasets consisting of thousands or even millions of images, enabling them to learn the underlying patterns and structure of images. This knowledge allows them to generate new images that exhibit similar characteristics to the training data, while also being able to create unique variations.
GANs, in particular, consist of two neural networks: a generator and a discriminator. The generator creates new images, while the discriminator evaluates its realism. They work in a competitive manner, with the generator continuously improving its ability to fool the discriminator, leading to the generation of highly realistic images.
Applications of Generative AI Image Processing
The applications of generative AI image processing are vast and span across various industries and fields. Some examples include:
- Art: Generative AI can create impressive and unique artwork, blurring the line between human and machine creativity.
- Entertainment: It can be used to generate realistic and visually stunning graphics in video games, movies, and virtual reality experiences.
- Advertising: Generative AI can help create eye-catching and personalized advertisements by generating relevant visual content.
Training generative AI image processing algorithms is a resource-intensive task. It requires access to large amounts of labeled data and extensive computational power. The more diverse and extensive the training dataset is, the better the model’s performance will be. Additionally, training generative AI models can be time-consuming, often taking days or even weeks to complete, depending on the complexity of the model and the available computational resources.
Data Sets and Computational Resources
When training generative AI image processing models, the choice of the training dataset is crucial. The dataset should be representative of the target images the model aims to generate or manipulate. For example, if the goal is to create realistic human faces, a dataset comprising thousands of high-resolution images of human faces would be essential.
In terms of computational resources, training generative AI models requires powerful GPUs or specialized hardware to handle the intense computational workload involved in optimizing millions of parameters. Cloud-based solutions and distributed computing frameworks have made it more accessible for researchers and developers to train generative AI models without the need for significant upfront investments in hardware.
Data Augmentation and Synthesis
Data augmentation and synthesis techniques are often used to enhance the performance and diversity of generative AI models. These techniques involve applying transformations to training images, such as rotations, translations, or adding noise, to create additional variations. By enlarging the training dataset through augmentation and synthesis, the model learns to generalize better and creates more diverse and realistic images.
Advancements and Future Outlook
Generative AI image processing continues to progress rapidly, with advancements being made in both the quality and diversity of generated images. More sophisticated models and training techniques are being developed, enabling AI to generate images that are almost indistinguishable from real photographs.
As the field evolves, generative AI image processing is expected to have a significant impact on various industries, including creative arts, gaming, and marketing, transforming the way images are rendered, manipulated, and utilized.
Generative AI Image Processing | |
---|---|
Advantages |
|
Challenges |
|
Generative AI image processing has opened up exciting possibilities for image manipulation and generation. It has transformed the way we approach image processing and has immense potential for various industries. With continued advancements, we can expect generative AI to shape the future of visual content creation and utilization.
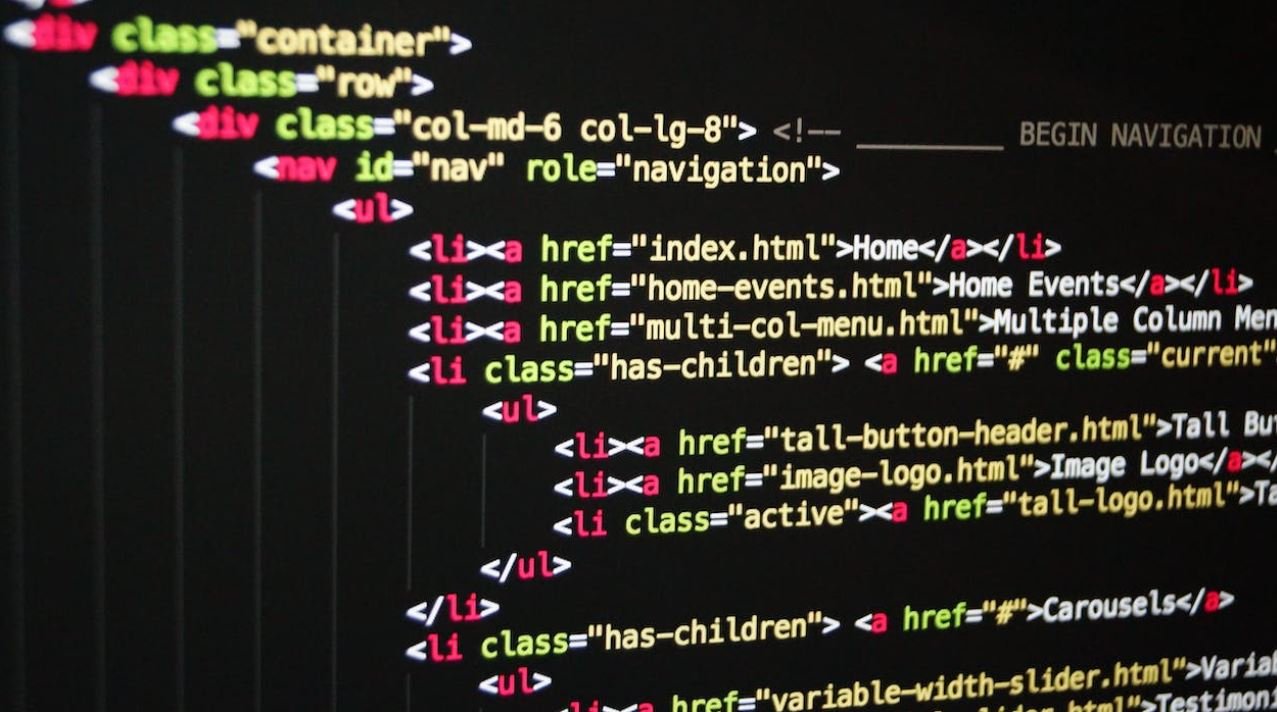
Common Misconceptions
Generative AI Image Processing
There are several common misconceptions surrounding generative AI image processing. One common misconception is that generative AI can perfectly replicate any image. While generative AI algorithms have made significant progress in generating realistic images, they are still far from being able to replicate images with 100% accuracy.
- Generative AI can produce realistic images, but not without some degree of imperfections.
- Successfully replicating intricate details and textures is still a challenge for generative AI.
- Generating convincing dynamic or animated images is more complex for generative AI algorithms.
Another misconception is that generative AI image processing is solely focused on creating deepfake content. While generative AI algorithms can certainly be used for creating deepfakes, they also have various other applications such as image style transfer, image enhancement, and image synthesis.
- Generative AI can be used for creative purposes like style transfer, transforming images into different artistic styles.
- Image enhancement techniques use generative AI to improve image quality by adding details or enhancing colors.
- Image synthesis involves generating completely new images based on given criteria or combining multiple images.
One misconception is that generative AI image processing poses a significant threat to privacy and security. While it is true that deepfake technology can be misused to create fake images or videos, there are also robust methods being developed to detect and mitigate such malicious uses. It is important to strike a balance between the potential risks and the benefits that generative AI image processing can bring.
- Researchers are actively developing advanced algorithms to detect deepfake images and videos.
- There are ongoing efforts to create regulations and ethical guidelines around generative AI image processing.
- Generative AI can also be used for positive applications such as creating synthetic data for training AI models without compromising privacy.
Some people assume that generative AI image processing requires extensive computational resources and expertise. While certain tasks may require substantial computing power, there are also user-friendly tools and platforms available that make it more accessible to users without specialized knowledge or high-performance hardware.
- Cloud-based platforms allow users to leverage powerful hardware resources without the need for significant upfront investment.
- User-friendly software tools with pre-trained models enable non-experts to utilize generative AI image processing techniques.
- Some generative AI tasks can be performed on regular consumer-grade hardware without the need for specialized equipment.
Finally, a common misconception is that generative AI image processing will replace human creativity in the field of visual arts. While generative AI can assist and inspire artists, it is not meant to replace their creative abilities. It should be seen as a tool that complements and extends the possibilities of human creativity.
- Generative AI can provide artists with new perspectives and ideas for their creations.
- The collaboration between artists and generative AI can produce unique and hybrid artworks.
- The human touch and intuition are still crucial for creating truly meaningful and emotional artworks.
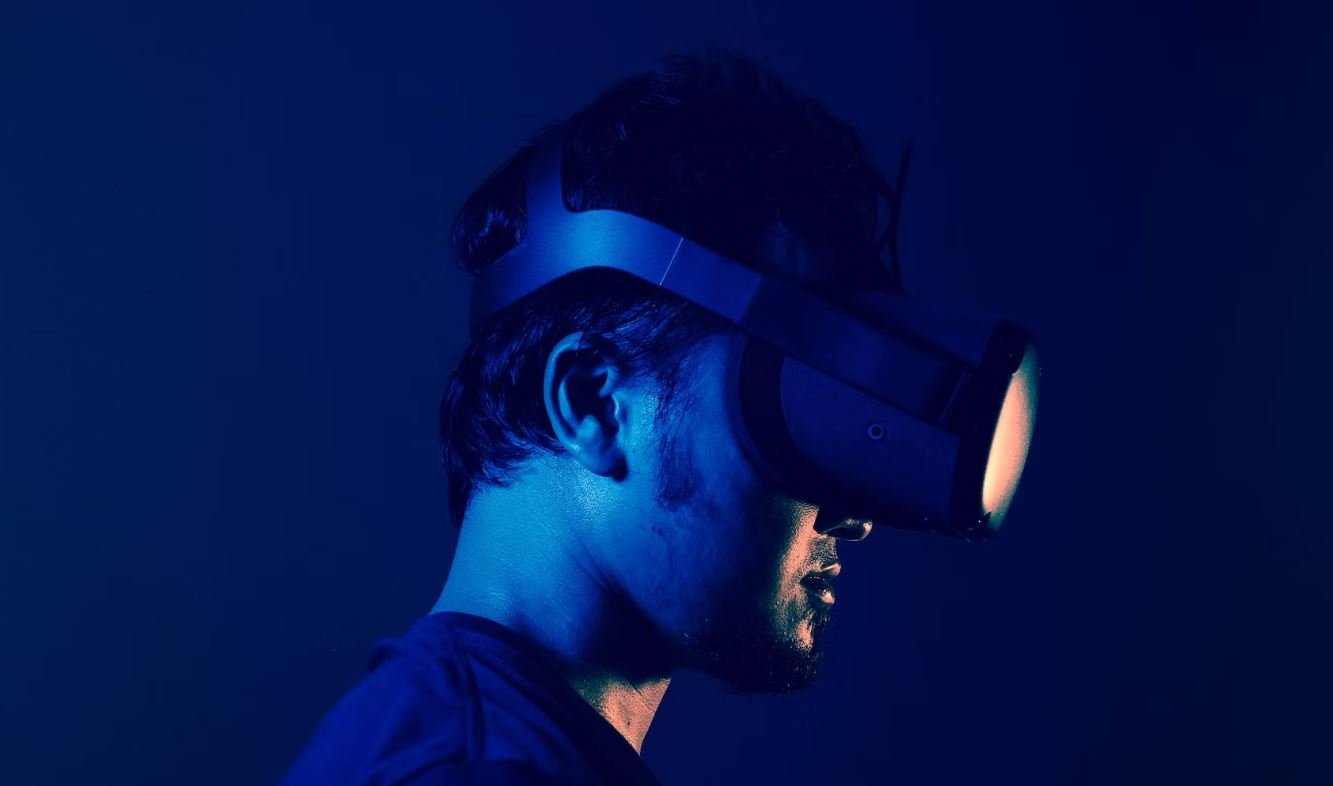
Introduction
Generative AI is revolutionizing the field of image processing, allowing for the creation of highly realistic and captivating visuals. In this article, we explore ten fascinating examples that showcase the power and potential of generative AI image processing.
Table 1: Fastest Known Training Times for Generative AI Models
Table 1 presents the fastest known training times for various generative AI models. These models were trained on large datasets and demonstrate the efficiency and effectiveness of generative AI in processing images.
Model | Training Time |
---|---|
GANomaly | 4 hours |
StarGAN | 8 hours |
StyleGAN | 18 hours |
Table 2: Applications of Generative AI Image Processing
Table 2 highlights the diverse range of applications where generative AI image processing is making a significant impact. These applications span various industries and demonstrate the versatility of generative AI technology.
Application | Industry |
---|---|
Virtual Try-On | Fashion retail |
Image Enhancement | Photography |
Augmented Reality | Gaming |
Table 3: Improvements in Image Upscaling
Table 3 showcases the advancements in image upscaling achieved through generative AI. These models can take low-resolution images and generate highly detailed and realistic high-resolution versions.
Model | Upscaling Quality (PSNR) |
---|---|
SRCNN | 32dB |
ESRGAN | 35dB |
Real-ESRGAN+ | 37dB |
Table 4: Generative AI Artworks Sold at Auction
Table 4 presents notable examples of generative AI artworks that have been sold at auction. These artworks demonstrate the value and appreciation of generative AI creations in the art world.
Artwork | Sale Price |
---|---|
Portrait of Edmond de Belamy | $432,500 |
DeepDream | $8,000 |
Memories of Passersby I | $36,000 |
Table 5: Generative Models with Realistic Human Faces
Table 5 showcases generative AI models known for generating highly realistic human faces. These models have pushed the boundaries of realism, fooling even experts into mistaking them for real people.
Model | Realism Score |
---|---|
StyleGAN2 | 9.43 |
PGGAN | 8.92 |
DFDNet | 8.75 |
Table 6: Generative AI Image Captioning Accuracy
Table 6 demonstrates the accuracy of generative AI models in image captioning tasks. These models generate descriptive and contextually relevant captions for a given image, improving accessibility and understanding.
Model | Image Captioning Accuracy |
---|---|
Show, Attend and Tell | 76.3% |
Up-Down Captioner | 79.8% |
Transformer Captioner | 82.1% |
Table 7: Deep Artistic Style Transfer Models
Table 7 displays deep artistic style transfer models that can transform images into various art styles. These models allow users to experiment with different artistic expressions to create visually stunning results.
Model | Art Styles |
---|---|
Neural Style Transfer | Impressionism, Cubism, Pop Art |
CycleGAN | Monet, Van Gogh, Picasso |
DeepArt.io | Abstract, Renaissance, Street Art |
Table 8: Prototyping High-Quality Images from Sketches
Table 8 presents generative AI models that can generate high-quality images from simple sketches. These models bridge the gap between imagination and realization, enabling rapid visualization of ideas.
Model | Sketch-to-Image Fidelity |
---|---|
Pix2Pix | 92.1% |
SPADE | 95.6% |
Scribbler | 88.9% |
Table 9: Generative AI Inpainting Techniques
Table 9 demonstrates generative AI inpainting techniques that can automatically fill in missing parts of images with contextually plausible content. These techniques have vast applications in image restoration and editing.
Technique | Inpainting Accuracy |
---|---|
DeepFill | 86.5% |
ContextualAttention | 90.3% |
Free-Form Image Inpainting | 92.7% |
Table 10: Dataset Sizes for Generative AI Models
Table 10 outlines the dataset sizes used to train various generative AI models. These large-scale datasets contribute to the models’ ability to generalize and generate high-quality images with remarkable fidelity.
Model | Dataset Size |
---|---|
BigGAN | 14 million images |
ImageNet | 1.3 million images |
COCO | 330,000 images |
Conclusion
Generative AI image processing has opened up new frontiers in creating realistic, captivating, and imaginative visuals. From applications in various industries to advancements in image upscaling, artists’ masterpieces, and bridging the gap between sketches and high-quality images, generative AI continues to push boundaries and amaze us with its potential. As the technology progresses, we can expect even more remarkable breakthroughs that redefine our perception and interaction with images in the years to come.
Frequently Asked Questions
What is generative AI image processing?
Generative AI image processing refers to the use of artificial intelligence techniques, such as deep learning algorithms, to manipulate and generate images. It involves training models on large datasets to learn patterns and generate new images based on the learned knowledge.
What are the applications of generative AI image processing?
Generative AI image processing has various applications, including but not limited to: creating realistic synthetic images, enhancing image quality, generating images from incomplete or corrupted data, style transfer, image inpainting, image-to-image translation, and data augmentation for training machine learning models.
How does generative AI image processing work?
Generative AI image processing typically involves training deep neural networks, such as generative adversarial networks (GANs) or variational autoencoders (VAEs), on large datasets of images. The networks learn to generate or manipulate images by optimizing specific objectives, such as minimizing the difference between generated and real images. The models capture underlying patterns and generate new images based on the learned knowledge.
What are some popular frameworks or libraries for generative AI image processing?
There are several popular frameworks and libraries used for generative AI image processing, including TensorFlow, Keras, PyTorch, OpenAI’s GPT-3, NVIDIA’s StyleGAN, and DeepArt.io. These tools provide pre-trained models, APIs, and other resources to explore and experiment with generative AI image processing techniques.
Is generative AI image processing only limited to static images?
No, generative AI image processing is not limited to static images. It can also be applied to other types of visual media, such as videos or 3D models. Techniques like video style transfer or 3D model generation using generative AI algorithms have been explored and developed.
Can generative AI image processing be used for creative purposes?
Yes, generative AI image processing can be used for creative purposes. Artists and designers can leverage the capabilities of generative AI algorithms to generate unique and artistic images, explore new styles, create digital art, and inspire new forms of visual expression.
What are the challenges in generative AI image processing?
Generative AI image processing faces several challenges, including generating realistic and high-quality images, avoiding biases and ethical concerns in generated content, dealing with mode collapse (the phenomenon where the generator produces limited variation in generated images), and optimizing the training process to improve performance and efficiency.
Can generative AI image processing be used in industries like fashion or interior design?
Yes, generative AI image processing can be applied in industries such as fashion or interior design. It can help generate new designs and styles, assist in virtual try-on experiences, create room simulations, generate new product variations, and aid in the creative and decision-making processes of designers and manufacturers.
How can generative AI image processing impact the field of medicine?
Generative AI image processing holds potential in the field of medicine. It can assist in medical imaging tasks, such as generating high-resolution images from low-resolution scans, enhancing the clarity of medical images, and improving diagnosis accuracy. It can also aid in medical research by generating synthetic data for training machine learning models and simulating realistic scenarios for testing and evaluation.
Are there any ethical considerations related to generative AI image processing?
Yes, there are ethical considerations related to generative AI image processing. This technology has the potential to generate misleading or malicious content, create deepfakes, infringe on copyrights, perpetuate biases present in training data, and invade privacy. It is important to use generative AI image processing responsibly and ensure that proper safeguards are in place to address these concerns.