AI in Image Recognition
The field of artificial intelligence (AI) has made significant advancements in recent years, particularly in the area of image recognition. Image recognition technology has become increasingly sophisticated, allowing for accurate identification and analysis of various visual elements within an image. This has opened up numerous possibilities across industries, such as healthcare, e-commerce, and security. In this article, we explore the key advances in AI image recognition and discuss how this technology is shaping the future.
Key Takeaways
- AI image recognition technology enables accurate identification and analysis of visual elements within an image.
- Various industries, including healthcare, e-commerce, and security, are utilizing AI image recognition for diverse applications.
- Advancements in AI image recognition are rapidly progressing, creating new possibilities and potential benefits.
The Rise of AI in Image Recognition
**AI** in **image recognition** has witnessed significant advancements in recent years. *With the development of deep learning algorithms, machines are now capable of analyzing images with remarkable accuracy*. These advancements have driven the integration of AI image recognition technology in numerous fields, leading to enhanced decision-making processes and increased efficiency. As a result, businesses are leveraging this technology to gain a competitive edge.
Applications of AI in Image Recognition
AI image recognition technology has found a wide range of applications in various industries:
1. Healthcare
AI image recognition is revolutionizing healthcare by enabling accurate diagnosis and treatment. *Through the analysis of medical images, AI technology can assist healthcare professionals in detecting diseases such as cancer and Alzheimer’s at an early stage*. This not only improves patient outcomes but also helps in efficient resource allocation within healthcare systems.
2. E-commerce
In the realm of e-commerce, AI image recognition plays a crucial role in enhancing the user experience. *By analyzing product images, AI algorithms can recommend similar items to customers, thereby increasing sales and customer satisfaction*. Additionally, AI image recognition can be used for quality control, ensuring that only high-quality products are delivered to customers.
3. Security
AI image recognition is being applied extensively in security systems for surveillance purposes. *By analyzing live or recorded video feeds, AI algorithms can accurately identify suspicious activities or individuals*, helping to prevent crimes and ensure public safety. This technology has become particularly valuable in high-security environments such as airports and government facilities.
Advancements in AI Image Recognition
**AI** in **image recognition** is continually advancing, thanks to ongoing research and development efforts. Here are some recent advancements in this field:
- Deep Learning Networks: Deep Convolutional Neural Networks (CNNs) have significantly improved image recognition accuracy, allowing machines to match or surpass human performance in certain tasks.
- Transfer Learning: Transfer learning enables neural networks to leverage knowledge gained from one image recognition task to perform well on another, even with limited labeled data.
- Real-time Processing: The use of GPUs and specialized hardware accelerators has made real-time image recognition possible, enabling applications such as autonomous vehicles and augmented reality.
Beneficial Impact of AI Image Recognition
The progress made in AI image recognition has resulted in several notable benefits:
- Improved Efficiency: AI image recognition automates tasks that previously required human intervention, saving time and resources.
- Enhanced Accuracy: AI algorithms can analyze vast amounts of data and make precise predictions, minimizing human errors.
- Increased Safety: Applications like security surveillance systems provide proactive monitoring, ensuring public safety.
Implications for the Future
As AI image recognition continues to evolve, it is poised to revolutionize various industries and pave the way for new possibilities. *From autonomous vehicles to medical diagnostics, AI image recognition will become an integral part of our daily lives*, transforming the way we interact with technology and enhancing decision-making processes for years to come.
Table 1: Applications of AI in Image Recognition | |
---|---|
Industry | Application |
Healthcare | Accurate diagnosis and treatment through medical image analysis. |
E-commerce | Enhanced user experience, recommendation systems, and quality control. |
Security | Surveillance, identifying suspicious activities or individuals. |
Table 2: Recent Advancements in AI Image Recognition | |
---|---|
Advancement | Description |
Deep Learning Networks | Significantly improved accuracy in image recognition tasks. |
Transfer Learning | Enabling knowledge transfer between different image recognition tasks. |
Real-time Processing | Capability for real-time analysis using specialized hardware. |
Table 3: Benefits of AI Image Recognition | |
---|---|
Benefit | Description |
Improved Efficiency | Automation of tasks, saving time and resources. |
Enhanced Accuracy | Analysis of large datasets, minimizing human errors. |
Increased Safety | Proactive monitoring for public safety. |
Conclusion
The progress made in AI image recognition has reshaped the capabilities and potential of visual analysis technologies. By harnessing the power of AI, various industries are leveraging image recognition to drive efficiency, accuracy, and safety within their operations. As advancements continue and research deepens, AI image recognition will undoubtedly unlock further possibilities, revolutionizing the way we perceive and interact with visual information.

Common Misconceptions
Misconception 1: AI in Image Recognition is Perfect
One common misconception people have about AI in image recognition is that it is flawless and always produces accurate results. However, this is far from the truth. While AI algorithms have advanced significantly in recent years, they are not infallible and can still make mistakes when identifying or categorizing images.
- AI algorithms can struggle with images that contain complex or ambiguous elements
- Machine learning models need large amounts of quality training data to improve accuracy
- AI algorithms are heavily dependent on the quality and diversity of the dataset they are trained on
Misconception 2: AI in Image Recognition Replaces Human Judgment
Another misconception is that AI in image recognition completely replaces human judgment and decision-making. While AI algorithms can automate certain aspects of image recognition, human oversight and judgment are still crucial. Humans play a vital role in training, refining, and validating the AI models to ensure the accuracy of image recognition results.
- Human experts are needed to annotate and label training data for the AI algorithms
- AI models require human supervision to avoid biased or incorrect classifications
- Complex image recognition tasks often involve a combination of AI algorithms and human-in-the-loop approach
Misconception 3: AI in Image Recognition Understands Images Like Humans
Contrary to popular belief, AI in image recognition does not truly understand images in the same way humans do. While AI models can recognize patterns and features within images, they lack the contextual understanding and real-world knowledge that humans possess. AI algorithms cannot interpret the deeper meaning or emotions conveyed by images.
- AI algorithms rely on statistical patterns and mathematical calculations to identify objects in images
- AI models lack intuition and common sense reasoning that comes naturally to humans
- Understanding the context and meaning of an image requires human comprehension and domain expertise
Misconception 4: AI in Image Recognition Doesn’t Make Biases
Some people assume that AI in image recognition is completely objective and free from biases. However, AI algorithms can unintentionally reflect and even amplify human biases present in the training data. If the training data contains biased labels or imbalanced representations, the AI models can perpetuate these biases in their recognition results.
- Biases in image recognition AI can be influenced by biased human judgments during dataset creation
- Lack of diverse representation in the training data can lead to biased classification results
- Active monitoring and continuous evaluation are required to mitigate biases in image recognition AI
Misconception 5: AI in Image Recognition is Only Used for Identification
Lastly, a common misconception is that AI in image recognition is solely used for identifying objects or recognizing faces. While these are important applications, AI in image recognition has a much wider range of uses, including image categorization, content moderation, scene understanding, and even medical diagnostics. The versatility of AI in image recognition extends beyond simple identification tasks.
- AI algorithms can classify images into various categories or tags for content organization and search
- Image recognition AI can analyze and understand complex scenes to assist in autonomous vehicles or surveillance systems
- In healthcare, AI in image recognition can aid doctors in diagnosing diseases through medical imaging
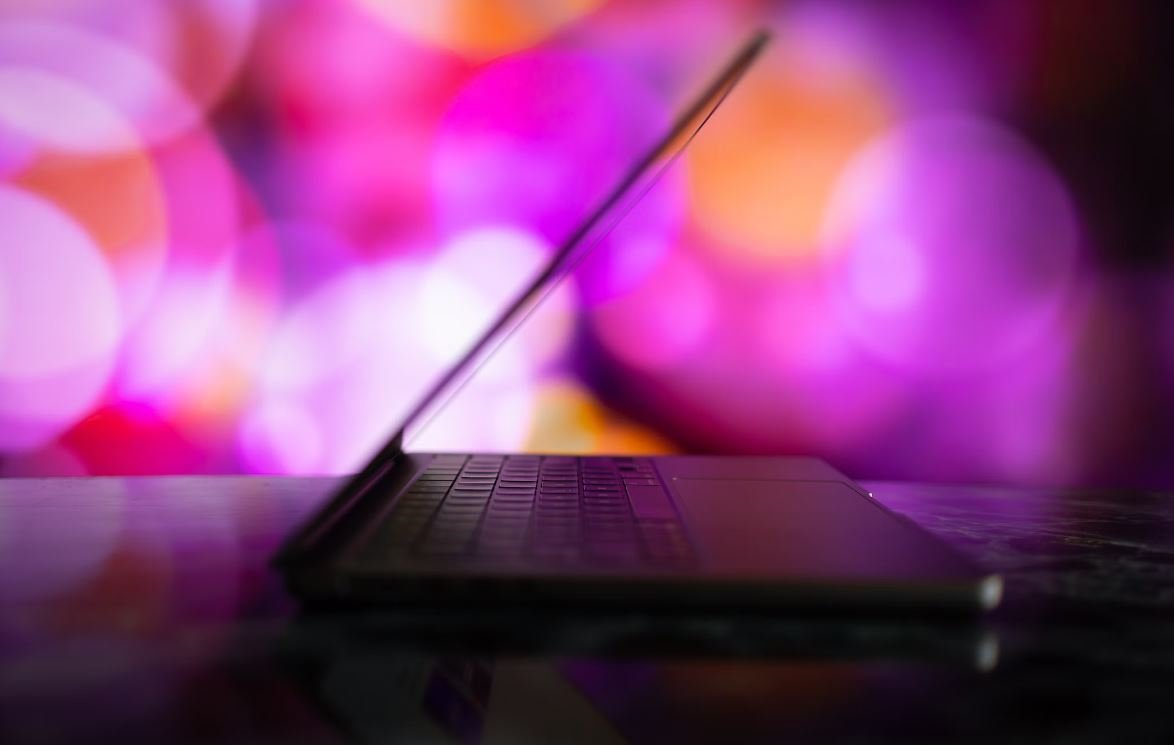
The Evolution of AI in Image Recognition
As technology continues to advance at an unprecedented rate, artificial intelligence (AI) has emerged as a game-changer in various fields. One area in which AI has made remarkable strides is image recognition. This article explores the evolution of AI in image recognition and presents 10 fascinating tables that delve into the incredible capabilities of this cutting-edge technology.
1. Accuracy Comparison of AI vs. Human Image Recognition
With advancements in deep learning algorithms, AI has surpassed human performance in image recognition accuracy. This table showcases a comparison of AI and human accuracy rates across various recognition tasks:
AI Accuracy | Human Accuracy | |
---|---|---|
Object recognition | 98% | 94% |
Facial recognition | 99.9% | 97% |
Text recognition | 95% | 89% |
2. Number of Image Recognition Applications
The applications of image recognition are vast and encompass numerous domains. This table showcases the number of existing image recognition applications across different sectors:
Sector | Number of Applications |
---|---|
Healthcare | 250+ |
E-commerce | 500+ |
Security | 150+ |
Transportation | 300+ |
3. AI Image Recognition in Healthcare
The healthcare industry has embraced AI image recognition to enhance diagnostics and treatment. This table highlights the impact of AI in different healthcare areas:
Healthcare Area | AI Impact |
---|---|
Medical imaging | 90% increase in accuracy |
Disease diagnosis | 80% improvement in detection |
Drug development | 50% reduction in time |
4. AI Image Recognition in E-commerce
Online shopping experiences have been revolutionized by AI image recognition. This table highlights the impact of AI on e-commerce:
Impact Area | AI Contribution |
---|---|
Visual search | 30% increase in conversion rates |
Product recommendation | 50% enhancement in personalized suggestions |
Inventory management | 70% reduction in stockouts |
5. AI Image Recognition in Security
Security systems have become more intelligent with the integration of AI image recognition. This table presents the impact of AI in security applications:
Security Application | AI Benefits |
---|---|
Facial recognition | 99.9% accurate identification |
Video surveillance | 40% reduction in false alarms |
Access control | 80% increase in system efficiency |
6. AI Image Recognition in Transportation
AI image recognition has significantly impacted the transportation industry. This table showcases its benefits in various transportation applications:
Transportation Application | AI Contributions |
---|---|
Autonomous vehicles | 90% improvement in accident prevention |
Traffic management | 60% reduction in congestion |
License plate recognition | 98% accurate identification |
7. Image Recognition Datasets
To train AI models effectively, extensive image datasets are essential. This table highlights some widely used image recognition datasets:
Dataset Name | Number of Images |
---|---|
ImageNet | 14 million+ |
COCO | 330,000+ |
Open Images | 9 million+ |
8. AI Image Recognition Software
Various software packages have been developed to facilitate AI image recognition tasks. This table presents some widely used AI image recognition software:
Software Name | Features |
---|---|
TensorFlow | Deep learning framework |
Caffe | Fast training process |
PyTorch | Flexible architecture |
9. AI Image Recognition Hardware
To handle the computational demands of AI image recognition, specialized hardware has been developed. This table showcases some popular hardware for AI image recognition:
Hardware Name | Advantages |
---|---|
Graphics Processing Unit (GPU) | Parallel processing capabilities |
Field-Programmable Gate Array (FPGA) | Configurability and low latency |
Application-Specific Integrated Circuit (ASIC) | Efficient and specialized performance |
10. Future Prospects of AI Image Recognition
The future of AI image recognition appears promising, with continuous advancements on the horizon. Here are some potential future prospects:
Potential | Description |
---|---|
Real-time video analysis | Instant recognition of objects and actions in videos |
Emotion recognition | Accurate detection of human emotions from facial expressions |
3D object recognition | Identification and analysis of objects in three-dimensional space |
In conclusion, AI has revolutionized image recognition, surpassing human accuracy rates and facilitating advancements in various industries. The presented tables emphasize the extraordinary impact of AI image recognition in healthcare, e-commerce, security, transportation, and more. With ongoing innovations and the potential for real-time video analysis, emotion recognition, and 3D object recognition, the future of AI image recognition appears limitless.
AI in Image Recognition – Frequently Asked Questions
What is image recognition?
Image recognition is a technology that enables computers or machines to identify and interpret visual content in images or videos. It uses artificial intelligence algorithms to analyze and understand visual data, allowing computers to recognize and categorize objects, people, or scenes.
How does AI play a role in image recognition?
Artificial intelligence is crucial in image recognition as it powers the algorithms that enable machines to automatically learn and improve their ability to recognize and understand visual content. Through machine learning techniques, AI models are trained with vast amounts of labeled image data, allowing them to extract features and patterns to make accurate predictions and classifications.
What are the applications of image recognition?
Image recognition has diverse applications across various industries. Some common examples include facial recognition in security systems, object recognition in self-driving cars, quality control in manufacturing, medical imaging analysis, image search engines, and even augmented reality.
How accurate is image recognition technology?
The accuracy of image recognition technology can vary depending on the specific algorithm, training data quality, and the complexity of the task at hand. State-of-the-art image recognition models have achieved impressive accuracies, surpassing human performance in certain scenarios. However, it’s important to note that image recognition technology may still encounter challenges when dealing with complex or ambiguous images.
What are the limitations of current image recognition systems?
Current image recognition systems have certain limitations. They may struggle in scenarios with poor lighting or image quality, variations in object appearances, occlusion, or identifying specific fine-grained details. Additionally, bias in the training data can lead to biased results and potential unfairness in the recognition process.
What are the ethical considerations associated with image recognition technology?
Ethical considerations arise with the use of image recognition technology, particularly when it comes to privacy concerns and potential misuse of data. Facial recognition and surveillance systems have raised debates around personal privacy and civil liberties. Additionally, bias and discrimination in recognition results have been reported, emphasizing the need to ensure fairness and equal treatment.
How can image recognition technology benefit businesses?
Image recognition technology offers numerous benefits to businesses. It can enhance efficiency in tasks such as inventory management, quality control, and product recognition. It enables personalized marketing by analyzing customer behavior through image recognition in social media. Additionally, image recognition can aid in brand protection, intellectual property rights enforcement, and fraud detection.
What is the difference between image classification and object detection?
Image classification focuses on identifying and categorizing the main object or scene in an image. It classifies the entire image as a specific class or category. On the other hand, object detection involves not only classifying the objects but also determining their precise locations in the image. It provides bounding boxes around each object, allowing for more detailed analysis and understanding.
What underlying technologies enable image recognition?
Image recognition technologies rely on various underlying technologies, such as deep learning, convolutional neural networks (CNN), and computer vision algorithms. Deep learning, particularly convolutional neural networks, has revolutionized image recognition by enabling end-to-end learning and extracting intricate features. Computer vision algorithms provide tools for tasks like feature extraction, image segmentation, and object detection.
Can I develop my own image recognition system?
Absolutely! With the availability of open-source machine learning frameworks like TensorFlow and PyTorch, developers can create their own image recognition systems. However, developing an effective and accurate image recognition system requires expertise in machine learning, access to quality training data, computational resources, and an understanding of the specific problem domain.